Executive summary
Natural Language Processing (NLP) holds a transformative power in the business world. This article explores the role of NLP in AI. The article also details its operations and extensive applications, ranging from sentiment analysis to topic modeling. We also explore NLP’s influence across various industries, including healthcare, banking, and retail, highlighting its capacity to enhance customer interactions and streamline operations. The article also talks about key NLP models and their real-world use cases. Read on, as this is your essential guide to understanding and leveraging NLP for business success.
As businesses navigate the digital age, Natural Language Processing (NLP) emerges as a fundamental shift in how we interact with technology. Gone are the days of rigid, keyword-based interactions; NLP ushers in an era where AI understands and responds to human language with unprecedented finesse. Picture a scenario where your AI system not only recognizes customer queries but also perceives their underlying emotions and context, offering responses that are accurate, empathetic, and contextually relevant. It is the fundamental advantage of NLP – where AI transcends code and algorithms to engage with the nuances and complexities of human language.
NLP is the hero behind many of AI’s most astonishing feats – from chatbots that can negotiate like a seasoned diplomat to systems that can sift through mountains of data to find that needle-in-a-haystack insight. But what makes it groundbreaking is its ability to democratize data, making complex information accessible to all, irrespective of technical know-how. This article peels back the layers of NLP, revealing its inner workings, practical applications, and immense potential to reshape business landscapes. Join us as we explore Natural Language Processing in AI and answer questions like ‘What is Natural Language Processing?’ and ‘How does Natural Language Processing work?’ and explain why NLP is a technology about understanding human communication’s very fabric.
What is Natural Language Processing (NLP)?
Natural Language Processing (NLP) is a revolutionary branch of artificial intelligence that enables computers to interpret, understand, and respond to human language. This process is done in a way that mimics human comprehension. NLP is a mix of computer science and linguistics, bridging human communication and machine interpretation. It enables machines to not only read text or hear speech but also grasp the nuances, context, and sentiment embedded within.
Imagine conversing with a machine as naturally as you would with a colleague. NLP makes this possible. It integrates computational linguistics, which applies rule-based language models, with sophisticated algorithms from machine learning and deep learning. This combination allows computers to process and ‘understand’ human language, whether written or spoken, capturing the essence of the speaker’s or writer’s intent.
NLP is the driving force behind many applications businesses use daily. From translation services that bridge language barriers to voice-operated systems that follow spoken commands, NLP enhances efficiency and accessibility. In the customer service industry, chatbots powered by NLP offer prompt, context-sensitive responses, transforming the customer experience. In the back office, NLP streamlines operations by summarizing vast text data, enabling real-time decision-making.
The domain of NLP is broad, encompassing two critical subfields: natural language understanding (NLU) and natural language generation (NLG). NLU focuses on comprehending and interpreting the meaning of language, ensuring the machine grasps the intended message. NLG, on the other hand, involves generating coherent and contextually relevant language, enabling machines to communicate ideas clearly and effectively.
Related must-reads:
- Top artificial intelligence (AI) trends for 2024
- The best AI chatbots in 2024
- Large language models: A guide on its benefits, use cases, and types
- Generative AI – The ultimate guide for 2024
Why does Natural Language Processing (NLP) matter?
In an era where digital communication reigns supreme, Natural Language Processing (NLP) has become indispensable for businesses striving to stay ahead. NLP matters immensely because it bridges the gap between human language and machine understanding, enabling businesses to interact with customers, analyze data, and automate processes in ways previously unimaginably. But why exactly is NLP so crucial in today’s business landscape?
1. Enhanced customer interactions
Firstly, NLP transforms customer interactions. With NLP-powered chatbots and virtual assistants like Amazon’s Alexa and Apple’s Siri, businesses can offer instant, personalized customer service. These AI-driven agents understand customer queries, process them in real-time, and deliver responses that, besides being accurate, are also contextually relevant. This immediacy and personalization in customer service foster stronger customer relationships, enhancing satisfaction and loyalty.
2. Data analytics like never before
NLP’s role in data analysis is groundbreaking. NLP is a powerful tool for extracting meaningful insights in avalanche of unstructured data generated daily – from social media posts to complex medical records. It enables machines to process vast quantities of textual data and identify patterns, sentiments, and key information, providing businesses with actionable intelligence. This comprehensive analysis, free from human bias and fatigue, is invaluable in decision-making and strategizing.
3. Human-like language processing
The diversity and complexity of human language, with its numerous dialects, slang, and nuances, present a significant challenge in communication. NLP effectively navigates these complexities, allowing businesses to communicate with customers across different languages and dialects, broadening their reach and inclusivity.
4. The business necessity of NLP
NLP’s importance extends beyond mere language processing. It brings syntactic and semantic understanding to the forefront, adding a layer of sophistication to AI models. This advancement is about understanding words and grasping their intent, sentiment, and broader context. Such deep understanding is crucial in applications like speech recognition, sentiment analysis, and text analytics, which are pivotal in shaping business strategies and customer experiences.
Gartner report
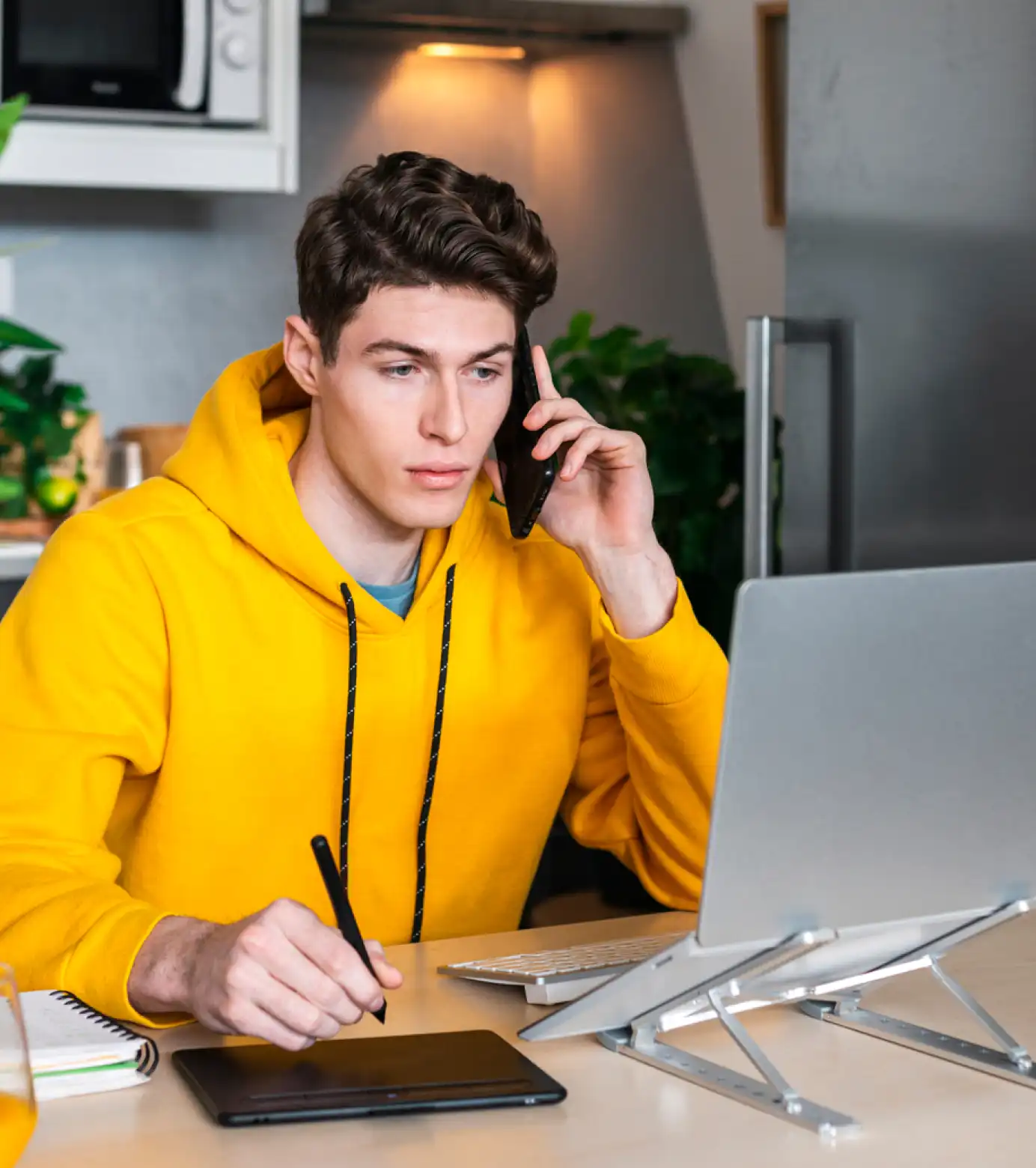
What is Natural Language Processing (NLP) used for?
NLP applications offer unique benefits and opportunities for businesses to optimize operations, engage customers, and gain insights. Let us look at the applications of NLP that companies can leverage to enhance their competitiveness and stay ahead in an increasingly digital world.
1. Sentiment analysis
At its core, sentiment analysis enables businesses to decipher the emotional tone behind texts. It is a critical tool for businesses to gauge public opinion and customer sentiment. By analyzing textual data from social media, reviews, and surveys, NLP algorithms categorize sentiments into positive, negative, or neutral.
Related read: Customer sentiment analysis – Why you must focus on it?
For instance, a company might use sentiment analysis to monitor social media reactions during product launches. Besides helping understand customer satisfaction, it also helps identify areas for improvement or respond to customer concerns proactively.
2. Toxicity classification
Toxicity classification is vital for maintaining healthy online communities and brand reputation. NLP systems analyze user-generated content to identify and filter out harmful language, including hate speech and bullying. For example, a social media platform might deploy NLP to moderate comments automatically, thus maintaining a positive and safe environment for its users.
3. Machine translation
Thanks to NLP, machine translation has made significant strides, allowing seamless translation between languages. It breaks language barriers, allowing businesses to reach a global audience. NLP-powered tools like Google Translate provide real-time translation of web content, emails, and documents. It is not just about translating words but understanding context and cultural nuances, which is crucial for businesses expanding into new international markets.
4. Named entity recognition (NER)
NER is an NLP function that identifies crucial elements like names, places, and organizations in text. It is instrumental in extracting specific information from large datasets, aiding in everything from customer data management to competitive analysis. For instance, news aggregation platforms use NER to categorize articles by entities mentioned.
5. Spam detection
NLP-driven spam detection systems protect users from irrelevant or malicious content. In email management, spam detection powered by NLP significantly enhances productivity. Email services like Gmail utilize NLP to analyze email content, separating legitimate correspondence from spam. Besides saving time, it helps in focus on important communications, a critical aspect of business operations.
6. Grammatical error correction
NLP is instrumental in tools like Grammarly, which assist in identifying and rectifying grammatical errors. It ensures that business communications are grammatically correct and professionally presented. It is imperative in customer-facing content and internal communications, where clarity and professionalism are paramount.
7. Topic modeling
Topic modeling is an unsupervised NLP task that discovers abstract topics within a collection of documents. It helps uncover themes in extensive text collections, enabling businesses to identify trends and patterns in consumer feedback or market research. It’s a tool for strategic insight, as seen in applications like customer feedback analysis, where businesses can pinpoint areas of improvement based on prevalent topics in customer reviews.
8. Text generation
NLP has significantly advanced text generation, creating content ranging from marketing copy to technical reports. AI models like GPT-3 have been used to generate creative content, automate routine reporting, and even draft code, showcasing NLP’s versatility in various business contexts.
9. Information retrieval
Information retrieval powered by NLP enhances the functionality of search engines and recommendation systems by reviewing a group of documents and producing a sorted document list that ought to be relevant to the user’s query criteria. It makes them more efficient at matching queries with relevant results.
For instance, Google’s search algorithm employs NLP for more accurate and contextually relevant search results, enhancing user experience. Another example can be an e-commerce website, which might use NLP to improve product search results, making it easier for customers to find what they’re looking for and thereby improving the shopping experience.
10. Summarization
Summarization tools powered by NLP condense lengthy documents into concise overviews. It is invaluable for businesses to digest extensive reports or research papers quickly. Such tools can extract critical points, making large volumes of data more accessible for decision-making. This application is beneficial for executives and decision-makers who need to quickly grasp the essence of data without going through the entire text.
11. Question answering
NLP enables systems to provide accurate answers to user queries, streamlining customer support. It allows systems to provide precise answers to user queries, as seen in virtual assistants like Siri and Alexa.
AI-driven virtual assistants, using question-answering capabilities, can handle routine customer queries, freeing up human agents to tackle more complex issues. It not only improves efficiency but also enhances the customer experience with quick and accurate responses.
How does Natural Language Processing (NLP) work?
The operationality of Natural Language Processing (NLP) is a fascinating mix of computer science, artificial intelligence, and linguistics. It’s a technology that allows machines to understand, interpret, and respond to human languages meaningfully. But how exactly does NLP work? Let’s decode the process that helps machines to comprehend human language.
1. Breaking down language into elements
The initial step in NLP involves breaking down the language into more minor, manageable elements. This process includes tasks like tokenization (splitting text into words or phrases), parsing (analyzing the grammatical structure of sentences), and part-of-speech tagging (identifying parts of speech in a sentence). These tasks are akin to the manual sentence diagramming we might have done in school.
2. Understanding syntax and semantics
NLP dissects language into structural components and delves into understanding the meaning and context. It involves lemmatization or stemming (reducing words to their base form) and identifying semantic relationships to understand how individual words and phrases relate to each other within sentences and larger contexts.
3. Advanced techniques and models
NLP leverages advanced machine learning and deep learning models, such as BERT (Bidirectional Encoder Representations from Transformers), to grasp the nuances of language. These models are trained on vast amounts of text data and can understand the context and meaning behind words in a sentence, thus improving the accuracy of language processing.
4. Practical applications of NLP techniques
These foundational tasks of NLP are employed in various higher-level applications:
- Content categorization: This involves summarizing documents, indexing content, and detecting duplication. It helps in organizing and retrieving information efficiently.
- Sentiment analysis: NLP analyzes vast quantities of text to gauge the mood and subjective opinions, making it possible to understand customer sentiment and market trends.
- Speech-to-text and text-to-speech conversion: NLP enables the conversion of spoken language into text and vice versa, facilitating voice-operated technologies like digital assistants.
- Document summarization: NLP can automatically create concise summaries of extensive texts, providing quick insights.
- Machine translation: One of the most well-known applications of NLP, machine translation helps in translating text or speech from one language to another, breaking down language barriers.
5. Corpus analysis and contextual extraction
NLP involves analyzing a corpus (an extensive collection of texts) to understand its structure and content. This analysis informs various tasks such as data preparation, strategy formulation for modeling approaches, and contextual information extraction from text-based sources.
6. Delivering enhanced value through linguistics and algorithms
The overarching goal of NLP is to enrich the text in a way that it becomes more valuable. Whether it’s categorizing content, extracting information, or converting speech, NLP uses a combination of linguistic knowledge and sophisticated algorithms to process and transform language data effectively.
Benefits of Natural Language Processing (NLP) across industries
Natural Language Processing (NLP) has transcended the realm of theory, cementing itself as a transformative technology across various industries. The ability of NLP to interpret and analyze human language brings unparalleled benefits to businesses, enhancing efficiency, understanding, and customer engagement. Let’s see how different sectors are leveraging NLP for remarkable advancements.
1. Healthcare: Revolutionizing patient care and data management
NLP is a game-changer in healthcare. By processing and analyzing patient data, electronic health records, and clinical notes, NLP aids in diagnosis and treatment planning. It streamlines data entry, allowing healthcare professionals to focus more on patient care rather than administrative tasks. Additionally, NLP tools help analyze unstructured medical literature and patient feedback, providing insights for better healthcare outcomes and personalized patient care strategies.
For example, NLP algorithms can scan through thousands of patient records to identify patterns and potential risk factors for diseases, facilitating early intervention and preventive healthcare measures.
Related must-reads:
- Healthcare chatbots – Benefits, use cases & how to build
- Conversational AI in healthcare
- Automation in healthcare: Transforming patient care at scale
- What is customer experience (CX) in healthcare?
2. Banking and insurance: Enhancing customer service and risk assessment
In the banking and insurance sectors, NLP plays a pivotal role in customer service and risk assessment. NLP-powered chatbots provide customers with quick responses to queries, personalized financial advice, and efficient claim processing. It improves customer experience and operational efficiency.
Moreover, NLP aids in analyzing large volumes of financial documents and reports, enabling better risk management and fraud detection. Banks and insurance companies use NLP to sift through transaction data to identify unusual patterns, enhancing the security and reliability of their services.
Related must-reads:
- 6 Examples of conversational AI in banking and why they are important for success
- Customer experience in banking: Why it matters more than ever
- Automation in Insurance: Examples, Benefits and More
- Conversational AI in insurance: Transforming the landscape of tomorrow
- Chatbots in Banking – Benefits & potential Use Cases
3. Retail and e-commerce: Personalizing shopping experiences
The retail and e-commerce industries harness NLP to offer personalized shopping experiences and enhance customer engagement. NLP tools analyze customer reviews, feedback, and browsing patterns to tailor product recommendations and marketing strategies.
For instance, an e-commerce platform can use NLP to understand customer preferences and behavior, leading to improved product placement and targeted promotions. Additionally, NLP-powered chatbots can assist customers in finding products, tracking orders, and resolving issues, elevating the overall shopping experience.
Related must-reads:
- The significance of conversational AI in retail
- Customer experience in retail: Strategies and the future of CX
- How retailers can benefit from chatbots
4. Education: Transforming learning and administrative efficiency
In education, NLP is transforming both teaching methodologies and administrative processes. It enables the development of interactive and personalized learning platforms where students receive customized educational content and assessments based on their learning styles and progress.
Furthermore, NLP streamlines administrative tasks like grading and feedback. For example, NLP tools can automatically grade essays and provide constructive feedback, saving educators time and allowing them to focus more on student engagement and teaching.
5. NLP across industries: Universal benefits of NLP
Across all sectors, NLP offers several universal benefits:
- Data analysis: NLP’s ability to analyze structured and unstructured data, such as social media posts, emails, and reports, provides comprehensive insights into consumer behavior, market trends, and operational efficiency.
- Cost reduction: By automating tasks like customer service and data analysis, NLP-enabled AI reduces operational costs, allowing businesses to allocate resources more effectively.
- Enhanced customer satisfaction: Through sentiment analysis and personalized interactions, NLP improves customer experiences, leading to higher satisfaction rates and brand loyalty.
- Market understanding: Conducting NLP analysis on data like customer reviews and social media posts helps businesses understand their target market better, informing product development and marketing strategies.
Featured Success Story
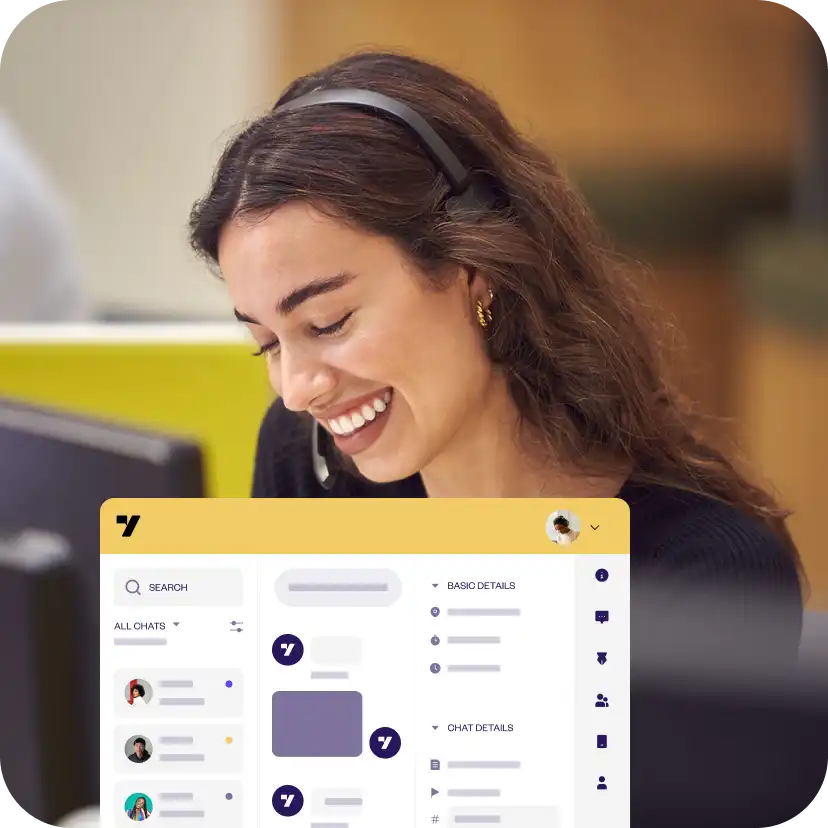
Six important Natural Language Processing (NLP) models
Natural Language Processing (NLP) has witnessed groundbreaking advancements through various models, each contributing significantly to the field. These models represent pivotal moments in the journey of NLP, showcasing the evolution of how machines understand human language. Let’s explore six key NLP models that have been instrumental in this technological revolution.
1. Rule-based models: The foundation of NLP
The early days of NLP were dominated by rule-based models, which relied on sets of hand-crafted rules. These models laid the groundwork for NLP by enabling basic parsing and understanding of language structures. They were effective in specific, limited contexts but lacked the flexibility to understand the nuances and variations of natural language.
2. Statistical models: Leveraging probabilities
Statistical models marked a significant shift, utilizing probabilities to predict language patterns. These models analyzed large text corpora to discern linguistic trends, allowing for more adaptive and context-aware language processing. They were particularly effective in tasks like machine translation and speech recognition, offering a more dynamic approach to language understanding.
3. Neural network models: Deep learning integration
The integration of neural networks, especially deep learning, brought about a transformative change in NLP. These models, powered by complex architectures like recurrent neural networks (RNNs) and convolutional neural networks (CNNs), excelled in capturing the intricacies of language. They provided a deeper semantic understanding and were pivotal in advancing tasks like sentiment analysis and text generation.
4. Transformer models: Revolutionizing contextual understanding
Transformer models, such as BERT (Bidirectional Encoder Representations from Transformers), represented a breakthrough in understanding context. Unlike previous models that processed text sequentially, transformers simultaneously analyze entire text blocks, capturing context more effectively. They have been fundamental in improving the performance of various NLP tasks, from question answering to text summarization.
5. Large language models: Scaling up capabilities
Recent years have seen the rise of large language models characterized by their vast size and scope. These models, trained on extensive datasets, excel in generating human-like text and understanding complex language patterns. They have significantly enhanced the capabilities of NLP applications, making them more versatile and powerful.
6. Customized and specialized models: Industry-specific applications
As NLP continues to evolve, there’s a growing trend towards customized and specialized models. These models are tailored to specific industries or applications, offering nuanced understanding and responses based on the particular needs of a domain. From healthcare to finance, these models are fine-tuned to handle industry-specific jargon and scenarios, providing more accurate and relevant outcomes.
Use cases of NLP: The versatile applications of NLP
Natural Language Processing (NLP) has various applications, each demonstrating the technology’s versatility and impact across different domains. The following use cases illustrate how NLP is transforming industries and enhancing human-machine interactions.
1. Healthcare: Diagnostic aid and patient care
In healthcare, NLP is revolutionizing patient care by diagnosing and analyzing patient feedback. It helps interpret electronic health records and identify potential health risks, thereby facilitating early intervention and personalized treatment plans.
Yellow.ai marketplace for healthcare
2. Customer insights: Sentiment analysis and market understanding`
The importance of customer sentiments cannot be understated in today’s business world. NLP provides invaluable insights into customer preferences and market trends. It examines social media, reviews, and customer interactions and helps businesses tailor their products and strategies to meet customer needs better.
3. Personalized assistance: Smart virtual assistants
Smart virtual assistants powered by NLP enhance personal and professional lives. They assist in various tasks, from setting reminders to providing quick information, demonstrating NLP’s role in making technology more accessible and user-friendly.
4. Financial sector: Fraud detection and risk management
In finance, NLP aids in fraud detection by analyzing transaction patterns and identifying anomalies. It plays a crucial role in risk management, helping financial institutions make informed decisions and safeguard their operations.
5. Legal industry: Document analysis and litigation support
NLP streamlines legal processes by analyzing case documents and legal precedents. It assists in litigation support, reducing the time and effort required for legal research and case preparation.
6. Recruitment and HR: Enhancing talent acquisition
NLP is transforming recruitment by analyzing resumes and job descriptions, helping HR professionals match candidates with suitable roles more efficiently. It also aids in identifying talent trends and workforce insights.
Related read: Recruitment chatbot – Ways to use for HR process
7. Education: Personalized learning and assessment
In education, NLP enables personalized learning experiences by tailoring content to individual student needs. It also assists in grading and providing feedback, enhancing the educational process.
Related read: Chatbots for education institutions – Use cases & benefits
8. Media and entertainment: Content curation and recommendation
NLP enhances user experience in media and entertainment through content curation and recommendation systems. It analyzes user preferences to suggest relevant content, enriching the viewing or reading experience.
9. Retail: Enhancing customer experience
In retail, NLP improves customer interactions by enabling more effective communication and understanding of customer needs. It plays a crucial role in providing personalized shopping experiences and improving customer service.
Related read: Customer experience in retail: Strategies and the future of CX
How Yellow.ai can help you to build an AI chatbot?
As we’ve explored the vast potential and applications of Natural Language Processing (NLP), the question arises: how can your business harness this power effectively? That is where Yellow.ai steps in, offering an advanced platform for creating AI chatbots that are not only smart but also intuitive and empathetic. Leveraging the Dynamic NLP model, Yellow.ai’s platform allows businesses to build chatbots that understand and respond to customer queries in a natural, human-like manner.
Whether it’s customer service, sales, or internal operations, Yellow.ai’s AI chatbots can be customized to meet specific business needs, ensuring seamless integration with existing systems and processes. With features like sentiment analysis, multilingual support, and real-time learning, these chatbots help improve customer engagement, reduce operational costs, and provide actionable insights. The platform’s intuitive design and powerful analytics tools make it easy to monitor and refine chatbot performance, ensuring continuous improvement in interactions.
Build your AI chatbot in minutes – no coding, no training required
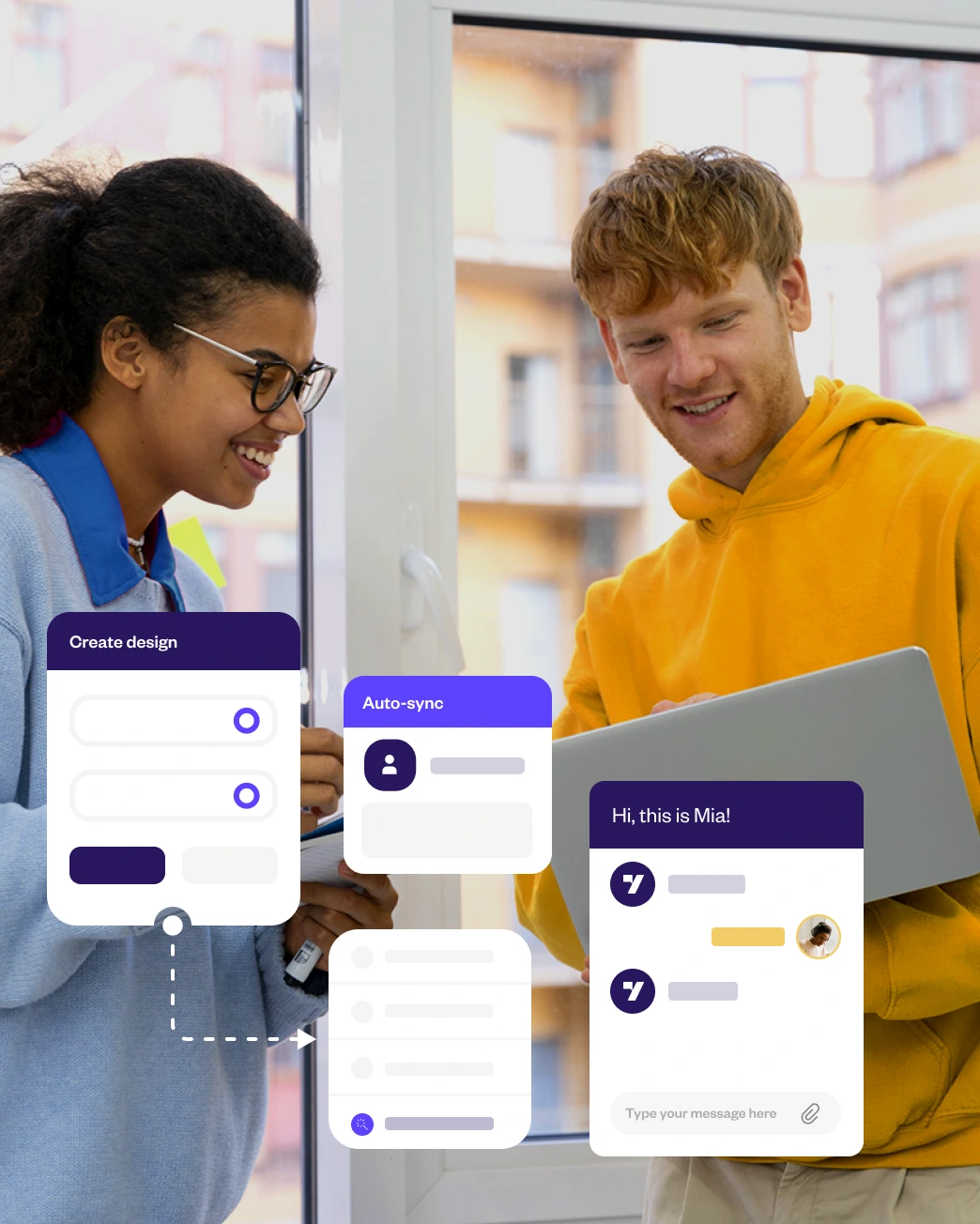
The final word on NLP
Navigating the complex world of Natural Language Processing can be daunting, but its potential to revolutionize business operations and customer interactions is undeniable. From enhancing communication to extracting valuable insights from data, NLP stands as a critical component in the evolving landscape of artificial intelligence and its application in business.
As we look to the future, the continuous advancements in NLP models and techniques promise even more innovative solutions, driving efficiency and growth across industries. With Yellow.ai’s dynamin NLP, brands can unlock new horizons of possibilities and stay ahead in a rapidly changing digital world.
NLP – Frequently asked questions (FAQs)
What is natural language processing in AI?
Natural language processing (NLP) in AI refers to the technology enabling machines to understand, interpret, and respond to human languages. It bridges the gap between human communication and computer understanding, making it possible for AI systems to process text and speech in a way similar to human comprehension.
What is NLP with an example?
NLP, or Natural Language Processing, involves training computers to understand and process human language. A classic example is chatbots used in customer service, which utilize NLP to interpret and respond to customer inquiries in real-time. Another example is sentiment analysis, where NLP algorithms analyze customer feedback on social media or review platforms to determine the overall sentiment towards a product or service, be it positive, negative, or neutral.
What are the 7 stages of NLP?
The seven stages of NLP encompass a comprehensive process for analyzing and understanding human language. They include:
- Text Preprocessing, where text is cleaned and standardized.
- Parsing and Part-of-Speech Tagging are used to understand grammatical structure.
- Named Entity Recognition to identify important terms.
- Coreference Resolution, determining which words refer to the same entity.
- Sentiment Analysis to understand the tone of the text.
- Semantic Role Labeling, identifying the underlying meaning.
- NLP Applications, where the processed data is applied to real-world tasks.
Why do we use NLP?
NLP is used to make human-machine interactions more intuitive and efficient. It allows computers to understand and respond to human language, enabling them to perform tasks like translation, sentiment analysis, information extraction, and automated customer service. NLP is crucial in processing and analyzing the vast amounts of data generated daily, helping businesses gain insights, improve decision-making, and enhance customer experiences.
What is the scope of NLP?
The scope of NLP is expansive and continuously evolving. It extends across various sectors, including healthcare for patient record analysis, finance for market trend analysis, customer service for chatbot interactions, and much more. NLP’s capability to process and understand human language makes it a valuable tool for businesses to automate operations, gain insights from data, and improve customer engagement.
What are natural language processing models?
Natural language processing models are advanced algorithms designed to understand, interpret, and generate human language. They range from rule-based models, which follow predefined language rules, to machine-learning models that learn from data. Notable models include BERT for contextual language understanding and GPT-3 for generating human-like text, among others. These models are the backbone of NLP, enabling various applications like text translation, sentiment analysis, and chatbots.
How to apply NLP in business?
Applying NLP in business involves integrating NLP technologies into various operations to enhance efficiency and customer engagement. For instance, businesses can use NLP-powered chatbots for customer service, implement sentiment analysis for market research, or use language translation tools for global communication. NLP can also automate data analysis, extracting valuable insights from unstructured data like emails, social media posts, and customer reviews.
What are the benefits of NLP in banking?
In banking, NLP offers numerous benefits, such as enhancing customer service through AI-driven chatbots that provide quick, accurate responses to inquiries. It aids in analyzing financial documents for risk assessment, detecting fraudulent activities through transaction pattern analysis, and personalizing banking services based on customer data analysis. NLP technologies can also streamline compliance and reporting processes, making banking operations more efficient and customer-centric.
What is the use of NLP in retail?
In retail, NLP is used to enhance customer experiences and streamline operations. It powers chatbots for customer inquiries, helping to provide real-time assistance. NLP is instrumental in analyzing customer feedback and reviews for sentiment analysis and guiding product development and marketing strategies. Additionally, NLP can improve search functionality on e-commerce platforms, making product discovery more intuitive and tailored to individual preferences.