Different types of Generative AI stand as a testament to the field’s versatility and depth. Each type is meticulously designed for distinct functions and, together, weave an intricate neural network that mirrors the complexity and adaptability of the human brain. This advanced interplay allows Generative AI to not only perform tasks but also to create, innovate, and solve problems with unprecedented efficiency and ingenuity.
Generative AI is promising to amplify human potential and redefine the boundaries of what’s possible. The excitement around this technology is palpable across industries. According to a Forbes survey, 80% of Chief Data Officers (CDOs) express enthusiasm about the transformative power of Generative AI, with a belief that it will significantly impact their organizations. Furthermore, 45% either anticipate or are already witnessing its widespread adoption within their sectors, signaling a shift towards more innovative and intelligent business models.
In this blog, we will delve into the world of Generative AI. We’ll explore the various types of Generative AI and understand their unique functionalities, which are capable of learning, evolving, and creating. From artistic endeavors to complex problem-solving, Generative AI is not just reshaping industries; it’s setting the stage for a future where AI and human creativity coalesce to unlock new horizons.
Join us as we embark on this enlightening journey, decoding the complexities and celebrating the possibilities of Generative AI, a field that’s not just evolving but revolutionizing the very fabric of how we live, work, and think.
Related must-reads:
- Generative AI – The Ultimate Guide [2024]
- 9 Benefits of Generative AI in enterprises
- Conversational AI vs Generative AI: Explained (with examples)
- Goal-based conversations, powered by Generative AI
Introduction to Generative AI
The inception of Generative AI traces back several decades ago, with the development of the Eliza chatbot by Joseph Weizenbaum in the 1960s, marking the first instance of Generative AI. As technological advancements progressed and access to data widened, the potential and sophistication of Generative AI have grown exponentially, resulting in the systems we encounter in the present day.
Generative AI is primarily concerned with the generation of innovative and unique content, including text, images, and music. It employs intricate algorithms and neural networks that are adept at learning to emulate, adapt, and build upon the discovered patterns and styles, capturing the very core of creativity and innovative thinking.
How does Generative AI work?
Generative AI operates in a way that’s strikingly similar to the human brain’s method of making predictions and learning from the variances between those predictions and the actual outcomes. It starts with a software-encoded artificial neural network that remarkably simulates aspects of human intellect. This network can be envisioned as an elaborate 3D grid, with each point representing an artificial neuron. Organized into layers, these neurons interconnect through specific formulas known as ‘weights’ or ‘parameters.’ The true essence of Generative AI’s capability lies not merely in the number of neurons but in the extensive network of these interconnections, which are crucial for the AI’s learning and generative functions.
Within the domain of Generative AI, different types of Generative AI models hold specific roles. Recurrent neural networks (RNNs) for processing sequential data, generative adversarial networks (GANs) for the creation of lifelike images, variational autoencoders (VAEs) for encoding and reconstruction of data and transformers for large language models. These distinct types of Generative AI models, each with its specialized functions, collectively endow Generative AI with an almost intuitive ability to forecast, assimilate, and invent, continually refining its creations in a manner akin to human learning.
So, having skimmed the surface of the different types of Generative AI models and their unique roles, let’s dive deeper to understand each one in-depth, unraveling their capabilities fully.
Types of Generative AI models
Each Generative AI model is a unique architect of digital innovation, offering a specialized set of tools and methods. Their collective contributions shape the ever-evolving realm of generative artificial intelligence. Now, we’ll delve into the specifics of these models to uncover the depth and breadth of their influence.
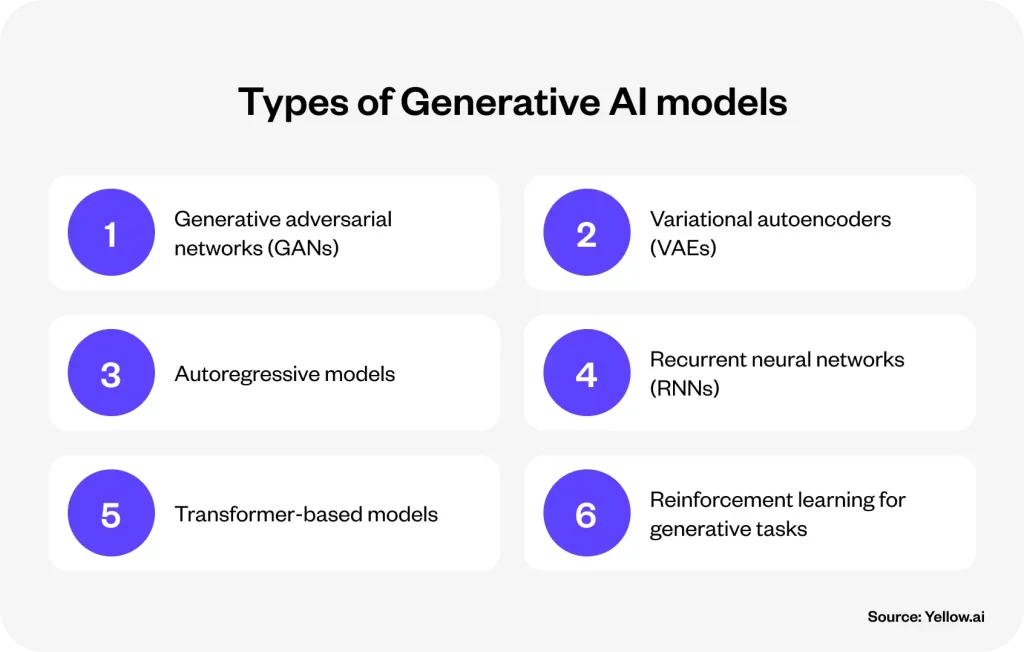
Type 1 – Generative adversarial networks (GANs)
Generative adversarial networks (GANs), a cornerstone in deep learning innovation, have profoundly influenced fields like image processing and biomedicine. At the heart of a GAN are two interdependent neural networks, known as the ‘generator’ and the ‘discriminator,’ engaged in a strategic contest.
Generator: This part of the network embarks on a creative quest, generating synthetic data based on a randomized noise signal. Whether it’s crafting images or emulating sounds, the generator continually refines its outputs to mimic real-world data closely.
Discriminator: Acting as the arbiter of authenticity, the discriminator compares the newly generated data against actual data, honing its skill to discern the real from the fake. Its role is crucial in guiding the generator towards producing more convincing and intricate content.
This intriguing game of deception and detection unfolds through an adversarial training process. The generator, striving to create data indistinguishable from reality, seeks to fool the discriminator. Meanwhile, the discriminator, aiming to preserve its ability to separate true from false, learns to identify subtle cues and nuances. GANs have embraced RNN and transformer variations, enhancing their adaptability and scope. This has led to significant advancements in creating photorealistic images, style transfers, and complex simulations, showcasing their unparalleled potential to synthesize authentic and detailed content.
Type 2 – Variational autoencoders (VAEs)
Variational autoencoders (VAEs), a class of generative models, have carved a niche in the AI landscape with their sophisticated approach to understanding and recreating data. They are particularly noted for their encoder-decoder architecture, which allows them to learn probabilistic representations of input data and generate new samples that capture the essence of the original dataset.
Encoder: This component of VAEs takes the input data and efficiently maps it into a latent space, a compressed representation capturing the data’s fundamental characteristics.
Decoder: Complementing the encoder, the decoder then takes this latent representation and reconstructs it back into the original data domain, aiming to mirror the initial input while introducing potential variations.
VAEs stand out due to their emphasis on learning the underlying distribution of the data. They balance the need for accurate reconstruction with regularization, ensuring that the generated samples not only resemble the original data but also maintain a degree of diversity and realism. During the training process, VAEs optimize their parameters to minimize reconstruction error while regulating the latent space, making it a rich source for generating new and diverse samples.
In the practical realm, VAEs have demonstrated their versatility and power across various domains. In image generation, they’re known for producing realistic and varied outputs. They’ve also made strides in anomaly detection, where understanding the distribution of normal data is crucial, and in data compression, where efficient encoding and decoding are key. Their ability to synthesize art and facilitate interactive exploration of latent spaces further underscores their potential, making them a valuable asset in the expanding universe of Generative AI.
Type 3 – Autoregressive models
Autoregressive models stand as a testament to the sequential nature of certain types of data, like text or music. These models excel in generating data one element at a time, basing each new piece on the previously generated elements. They work by predicting the probability distribution of the next element given the context provided by the preceding elements and then sample from this distribution to generate new data.
Autoregressive models like the Generative Pre-trained Transformer (GPT) have revolutionized fields like language modeling, generating coherent and contextually relevant text. But their influence doesn’t end there; they’re also instrumental in music composition and other sequence-based generation tasks, showcasing their ability to understand and replicate complex patterns and dependencies. Their unique structure and approach make them invaluable for tasks requiring a deep understanding of sequential context and the ability to produce coherent and relevant outputs.
Type 4 – Recurrent neural networks (RNNs)
A recurrent neural network (RNN) is a type of artificial neural network designed to recognize patterns in sequences of data, such as text, genomes, handwriting, or numerical time series data. Unlike feedforward and convolutional neural networks (CNNs), RNNs have a unique “memory” feature that allows information from previous inputs to influence current inputs and outputs. This makes them ideal for tasks like language translation, natural language processing, and speech recognition, where the sequence and context of the data are crucial for understanding and prediction.
RNNs operate using a backpropagation through time (BPTT) algorithm, a variant of traditional backpropagation suited for sequential data. However, they often encounter issues like exploding or vanishing gradients due to their deep and complex structure. While exploding gradients can lead to unstable models with overly large weights, vanishing gradients make learning halt prematurely. Advanced RNN variants like Long Short-Term Memory (LSTM) units and Gated Recurrent Units (GRU) have been developed to mitigate these issues, enhancing RNNs’ ability to capture long-term dependencies and maintain robustness in learning over time.
Type 5 – Transformer-based models
Transformer models, epitomized by the GPT series, have revolutionized natural language processing (NLP) and generative tasks with their advanced architectures. At the core of their innovation is the attention mechanism, which effectively models the relationships between different elements in a sequence. This allows Transformers to handle long sequences while maintaining context and coherence, a critical factor in generating contextually relevant text.
Unlike traditional models that process data sequentially, Transformers are parallelizable, significantly reducing training time and making them well-suited for tasks requiring an understanding of complex relationships within the data. One of the most notable applications of Transformer models is the Generative Pre-trained Transformer series, like GPT-3. These models have demonstrated remarkable capabilities in various NLP tasks, from text completion and question answering to translation and summarization. Their ability to generate coherent and contextually relevant text from a given prompt has set new standards in the field, showcasing their potential as powerful tools for a wide range of applications.
Type 6 – Reinforcement learning for generative tasks
Reinforcement learning represents a paradigm shift in generative tasks, emphasizing the role of feedback and interaction in the learning process. This method involves an agent that generates data and iteratively improves based on rewards or feedback from its environment. The crux of this approach is the continuous cycle of action, feedback, and adaptation, driving the agent towards producing higher-quality outputs.
One of the compelling applications of reinforcement learning in generative tasks is in text generation. Here, the model, acting as an agent, generates text and then refines it based on user feedback or predefined reward mechanisms. This approach ensures that the generated text isn’t just coherent but also aligns more closely with the desired outcome, whether it’s engaging dialogue, accurate information, or stylistic consistency. Reinforcement learning’s adaptability and focus on iterative improvement make it a powerful tool for enhancing the quality and relevance of generative models, pushing the boundaries of what these systems can achieve.
Pelago by Singapore Airlines reimagines customer experience with Generative AI-powered bots
Established by Singapore Airlines, Pelago, an innovative travel experience platform, partnered with Yellow.ai to deploy a bot on multiple digital channels. The bot is capable of understanding customer queries and responding with high accuracy for tasks such as bookings, cancellations, retrieving vouchers or tickets, live agent support, and addressing any issues or queries related to Pelago or the travel domain.
Other types of Generative AI with examples
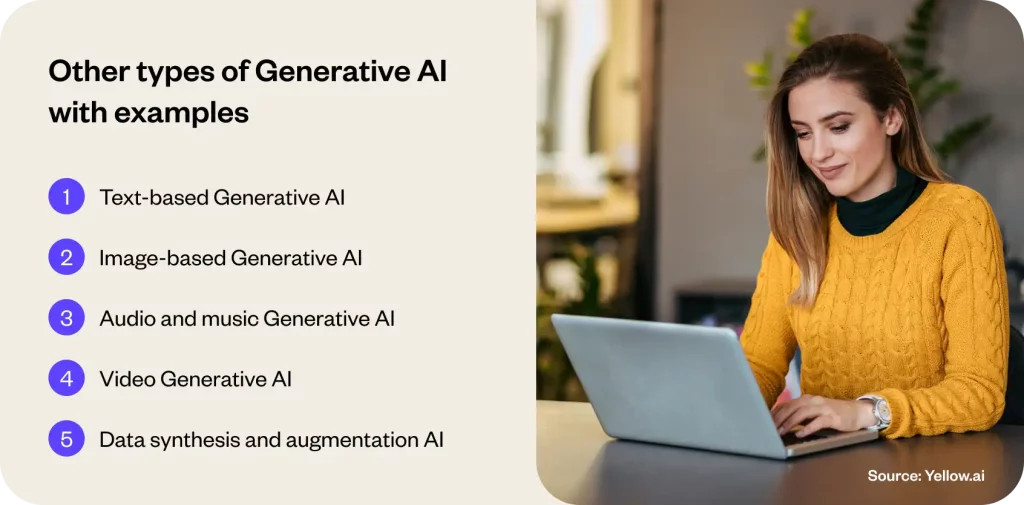
Text-based Generative AI
Text-based Generative AI is a branch of artificial intelligence focused on creating new, original text that mirrors human language. It begins by absorbing a vast array of textual data from books, articles, and websites, analyzing this information to understand patterns and relationships in human communication. Through deep learning and natural language processing, it learns syntax, semantics, and the intricate nuances of language, enabling it to generate coherent and contextually relevant content.
Once trained, these AI models can produce a wide variety of written content, including essays, blogs, scripts, news articles, and even poetry. The potential of text-based Generative AI extends beyond mere content creation; it’s an augmentative tool that assists writers, automates corporate communications, and enhances educational experiences. As this technology advances, it’s set to revolutionize further the way we create and interact with textual content, offering new avenues for creativity and communication. Examples of such advanced models include GPT-3 and BERT, which have set new benchmarks in the field.
Image-based Generative AI
Image-based Generative AI is trained to create new and unique images. It learns by examining large collections of images, often accompanied by captions or text descriptions, to understand what different objects and scenes look like and how they relate to each other. This understanding allows the AI to combine different concepts creatively, like imagining a tree with butterfly wings if it’s learned what both trees and butterflies look like.
The capabilities of Image-based Generative AI are vast. It can produce a wide array of images in different styles, from photorealistic pictures that look like a camera took them to artistic renderings that mimic the style of oil paintings or anime. Whether it’s creating new art, designing virtual environments, or helping visualize data in more understandable ways, Image-based Generative AI is opening up new possibilities for creativity and innovation. Examples of such advanced models include DALL-E and StyleGAN.
Audio and music Generative AI
Audio and music Generative AI is revolutionizing the way we create and interact with music. By digesting a wide array of musical tracks and associated metadata such as artist names, genres, and lyrics, these AI systems learn to recognize and replicate the complex patterns and emotional nuances that define various musical styles. The result is an AI capable of generating new, unique music that echoes the essence of its training data.
For instance, if an AI’s learning is predominantly based on the works of Beethoven, the compositions it generates will likely carry a classical tone, reminiscent of his style. This indicates the crucial role of diverse and comprehensive training in shaping AI’s creative capabilities. The potential of audio and music Generative AI extends beyond mere replication of existing music; it’s about forging new sonic landscapes, blending traditional elements with innovative AI-driven creativity. As this field matures, it’s set to redefine music production, offering tools for artists and creating entirely new musical experiences. Examples of such models include Jukebox and WaveNet.
Video Generative AI
Video Generative AI is reshaping how we produce and interact with video content. Unlike static images or text, videos are complex, dynamic compositions of audio, visual, and textual elements. Generative AI in this domain learns from a diverse set of sources, including existing videos and individual audio, visual, and text components, to understand how to craft new, coherent video content.
Some video Generative AI systems focus on learning from existing videos and analyzing the way elements interact over time to create engaging narratives or visual experiences. Others take a more component-based approach, learning how to effectively combine audio, visual, and text elements into a cohesive whole. Additionally, there are advanced AI programs equipped with knowledge of video editing software, enabling them to not only generate content but also apply sophisticated effects, transitions, animations, and captions, enhancing the final product. For example, Gen-1 Runway and Invideo are among the platforms leading the way in Video Generative AI.
Data synthesis and augmentation AI
Data synthesis and augmentation AI plays a critical role in enhancing and expanding the datasets used for training machine learning models.
Data synthesis: This aspect of AI generates entirely new, synthetic data points that closely resemble real-world data. The role here is to provide additional, realistic data where collecting real data might be challenging, expensive, or sensitive. In industries like healthcare or finance, where privacy is crucial, synthetic data allows for continued model training and testing without compromising individual confidentiality.
Data augmentation: Augmentation AI takes existing data and creates varied versions of it. Its role is to increase the diversity and volume of data available for training, leading to more robust models. For example, by slightly altering images or changing sentence structures in text data, models can learn to recognize patterns and make predictions more accurately, even when faced with new, unseen data. This is particularly important for preventing overfitting, where a model performs well on training data but poorly on new data.
Together, data synthesis and augmentation AI ensure that machine learning models have access to a wide range of high-quality data, enhancing their performance, accuracy, and ability to generalize. These technologies are vital for pushing the boundaries of what’s possible in AI, driving innovation, and improving outcomes across various fields.
Benefits of leveraging Generative AI with Yellow.ai
Yellow.ai enables businesses to offer interactions that are strikingly human-like and intelligent to their customers by incorporating different types of Generative AI models into chatbots and virtual assistants. Yellow.ai’s Dynamic Automation Platform (DAP) with Generative AI capabilities is a prime example of how such technology is transforming business-customer interactions. So, let’s understand the benefits of harnessing the power of Generative AI.
- Enhanced personalization: Yellow.ai’s DAP utilizes Generative AI to understand and adapt to individual customer preferences and behaviors. This leads to highly personalized interactions, where chatbots can provide tailored recommendations, responses, and services, making each customer feel understood and valued.
- Improved engagement: Generative AI enables the platform to conduct dynamic, goal-oriented conversations that go beyond simple question-answering. This capability ensures that customers are more engaged, leading to higher satisfaction rates and deeper brand loyalty.
- Greater efficiency: By automating routine inquiries and tasks, Yellow.ai’s DAP allows businesses to handle a higher volume of interactions with consistent quality. This not only improves response times but also frees up human agents to focus on more complex and sensitive issues.
- Continuous learning: The Generative AI in Yellow.ai’s DAP learns from each interaction, continuously improving its understanding and conversational abilities. Over time, this leads to smarter, more effective communication and an ever-improving customer experience.
- Scalability: As businesses grow, the demand for customer support can escalate rapidly. Yellow.ai’s DAP, powered by Generative AI, can easily scale to meet increasing demand without compromising on the quality or personalization of interactions.
- Insightful analytics: With Generative AI, Yellow.ai’s DAP can analyze and interpret vast amounts of interaction data, providing businesses with valuable insights into customer needs, preferences, and trends. This information can inform decision-making, strategy, and personalized marketing efforts.
How to gain 1000% on ROI with Yellow.ai and Generative AI
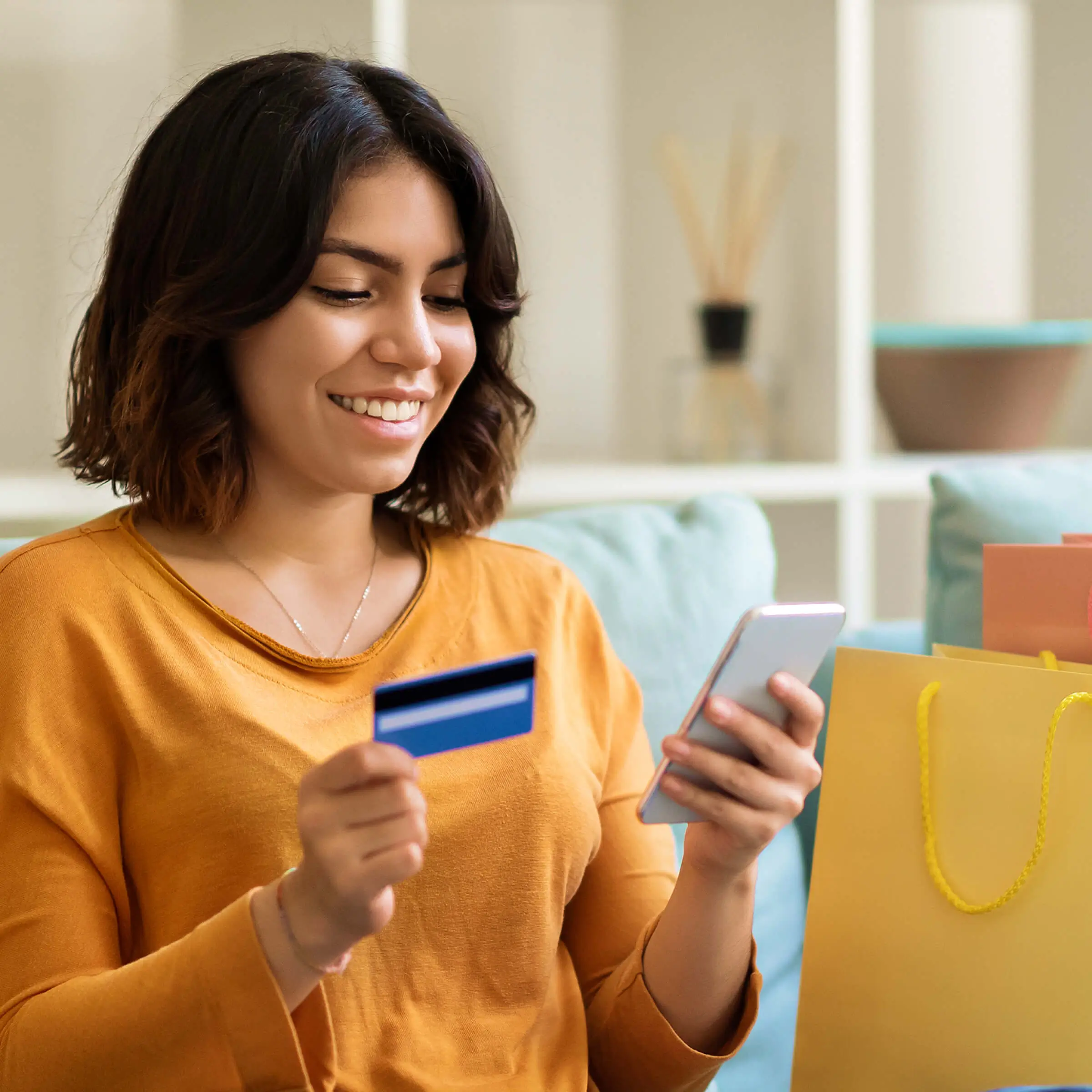
Conclusion
As we look to the future, it’s evident that the potential of Generative AI is only beginning to be tapped. With each advancement and application, we’re not just reshaping industries; we’re redefining the very essence of creativity and problem-solving. From text-based models that can draft essays and scripts to video generators that can craft immersive experiences, the types of Generative AI demonstrate an extraordinary range of capabilities. These systems, learning and evolving from vast datasets, reflect the complexity and adaptability of the human brain itself, promising to amplify human potential and push the boundaries of innovation.
This evolution of Generative AI is not just a technical achievement but a transformative force in society. It’s enabling more efficient ways of working, sparking new forms of art, and providing solutions to complex problems that were previously out of reach. As these technologies continue to advance, they will increasingly become partners in our daily lives, enhancing our capabilities, and opening up new avenues for exploration and discovery. In this transformative journey, platforms like Yellow.ai stand out by integrating Generative AI into dynamic and intelligent chatbots, setting new standards for innovation and interaction in the digital age.
Unleash creativity with Generative AI
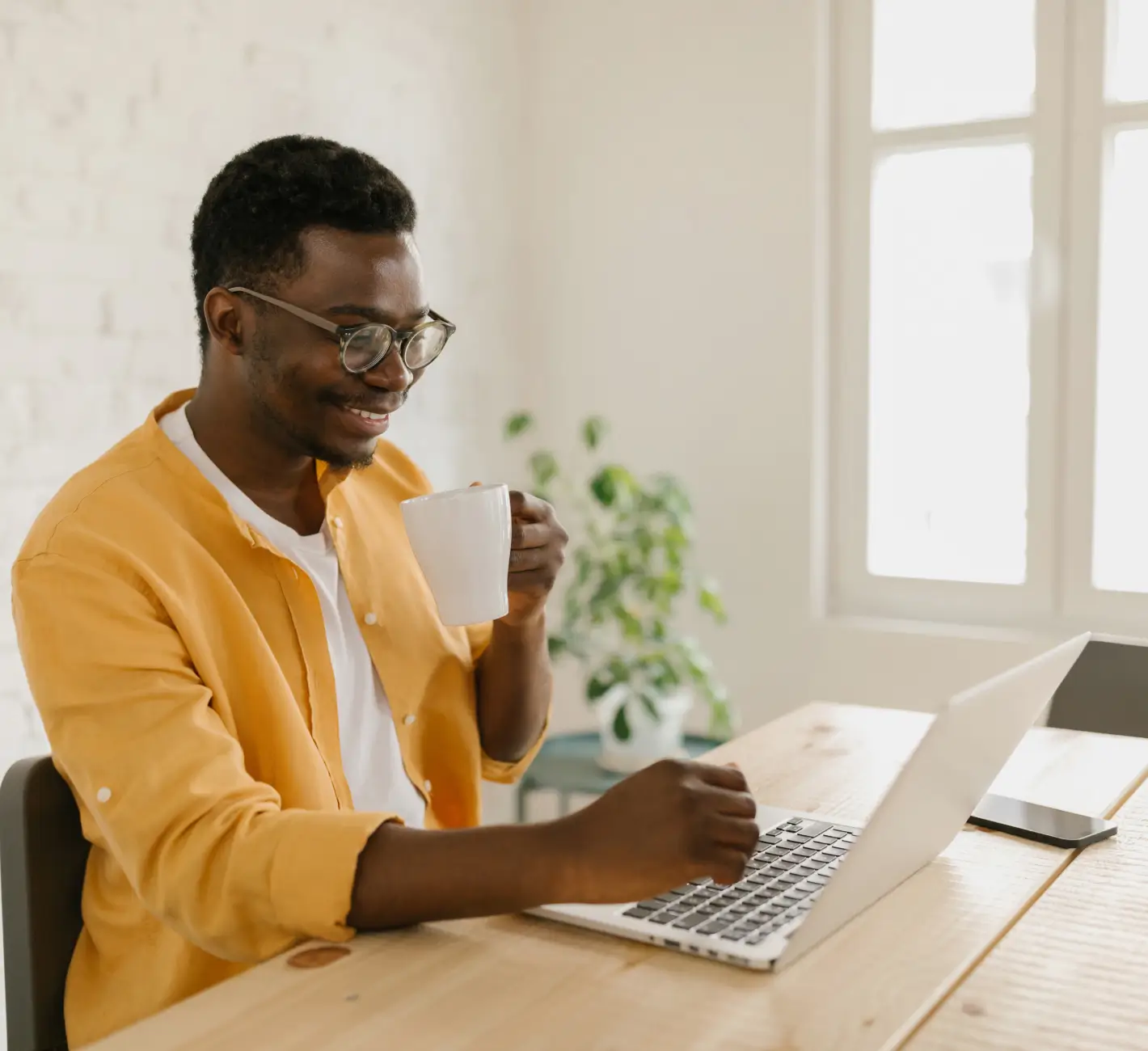