Gone are the days of robotic replies and lengthy implementation times. With the recent rise in Generative AI capabilities, Conversational AI platforms are now capable of having more advanced human-like conversations, while eliminating the need for coding conversational workflows and training the NLP.
This is due to the use of powerful large language models (LLMs) that are leveraged by these conversational AI platforms.
LLMs are at the forefront of Generative AI due to their ability to generate relevant and contextual text. However, they have limitations when it comes to meeting the high standards of secure, accurate, and seamless customer experiences benchmarked by enterprises.
The current LLMs are trained on large datasets, which results in slower response time as well as increased hallucinations. These challenges make it difficult for enterprises to adopt LLM.
Let’s take a look at how enterprises can leverage LLMs to provide elevated customer experiences.
Understanding large language models (LLM)
A large language model, known as LLM, is a powerful AI system designed to understand and generate human-like text. In recent times, LLMs such as OpenAI’s GPT-3 and GPT-4 have garnered attention with an easy-to-use interface, allowing users to create unique content.
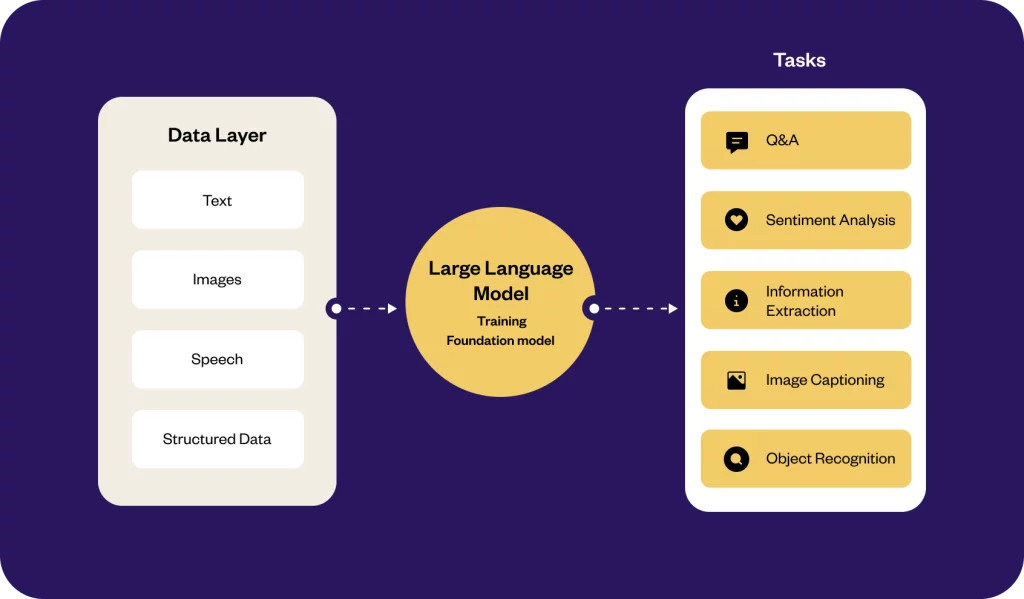
Under the hood, LLM is based on a neural network architecture that enables it to understand complex language and generate text.
- Deep learning: Using deep learning, LLMs are trained on vast amounts of data to learn grammar, nuances, and patterns of the language.
- Foundational models: There are foundational models such as Q&A and information extraction, which allow LLMs to create human-like responses.
- Generation: LLM examines the prompt and uses its training to generate a contextually correct and relevant response using natural language generation (NLG).
Currently, it is common to employ a single large language model (LLM) for multiple tasks such as text generation, question-answering, summarization, and more. However, leveraging a single LLM results in high computational time and an increased risk of generating nonsensical outputs.
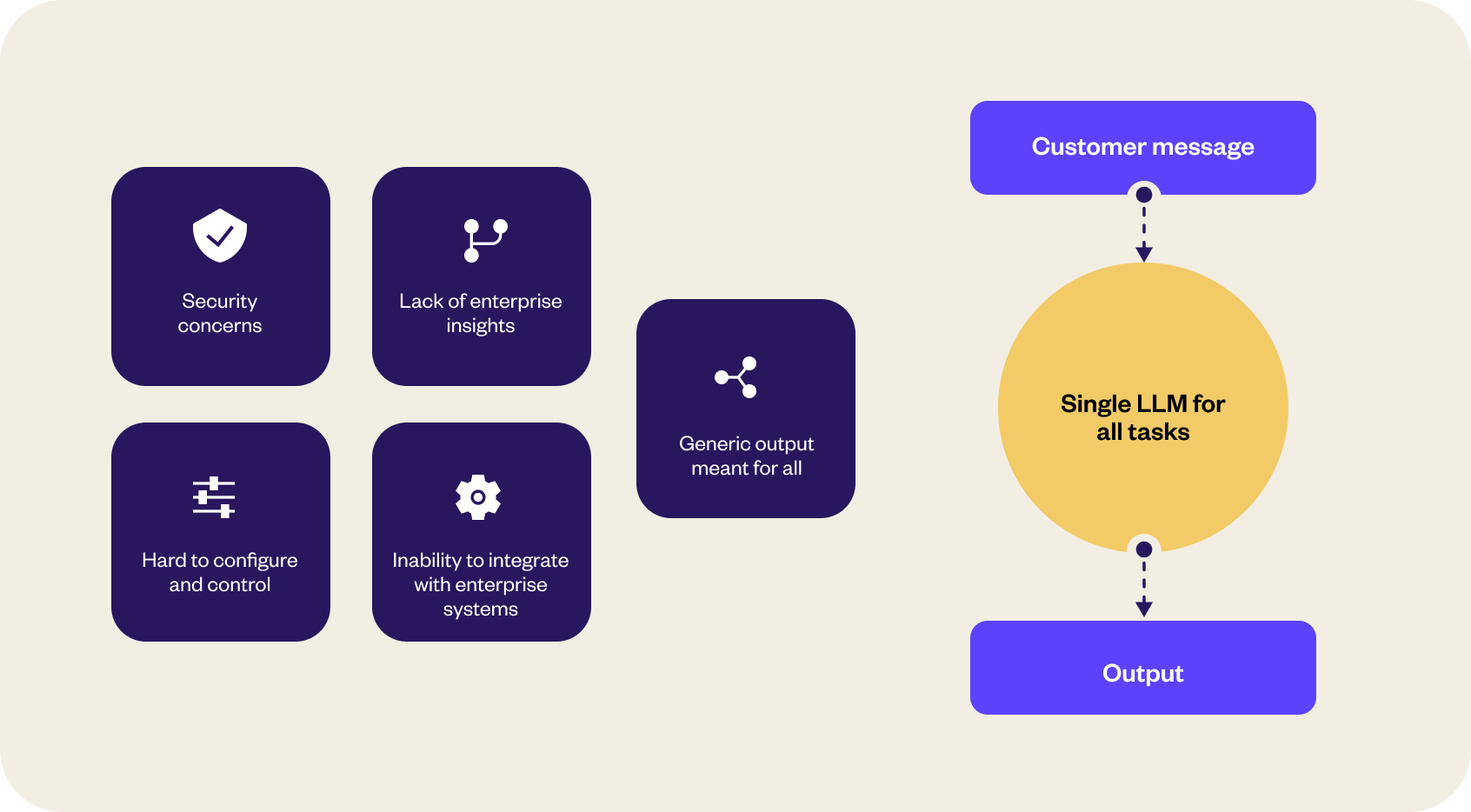
Here are some common challenges that need to be addressed before LLMs can be widely adopted for enterprise use.
- Security and data privacy concerns: Robust security measures are necessary when dealing with sensitive enterprise information, such as data encryption, access controls, and secure deployment environments, which are not guaranteed with present-day LLMs.
- Lack of enterprise insights: Since LLMs are driven toward catering to the masses, they do not provide accurate and context-aware answers specific to a business. LLMs are trained on vast amounts of data from the internet, which can contain biased or controversial information.
- Hard to configure and control: LLMs for business needs require intricate configuration and control mechanisms to meet their standards. It is difficult to tweak existing generic LLMs that can match an enterprise’s requirements.
- Inability to integrate with enterprise systems: Seamless integration with generic LLMs is quite tedious. However, this integration is essential to leverage enterprise data and interact with other systems effectively.
- Generic output meant for all: LLMs produce generic responses that do not align with the specific requirements and brand voice of enterprises.
Introducing YellowG LLM, an in-house foundation model designed for enterprise needs
While large language models (LLMs) can handle a wide range of tasks within a single model, we have taken a different approach with YellowG LLM by developing smaller models that specialize in specific tasks. Instead of relying on a one-size-fits-all model, our focus is on creating targeted models that excel in specific areas.
YellowG LLM is an advanced language model that offers a specialized and targeted approach to handle specific tasks. By leveraging these multiple smaller specialized models, YellowG LLM outperforms larger models ensuring near 0% hallucinations, improved response times, and enhanced customer interactions.
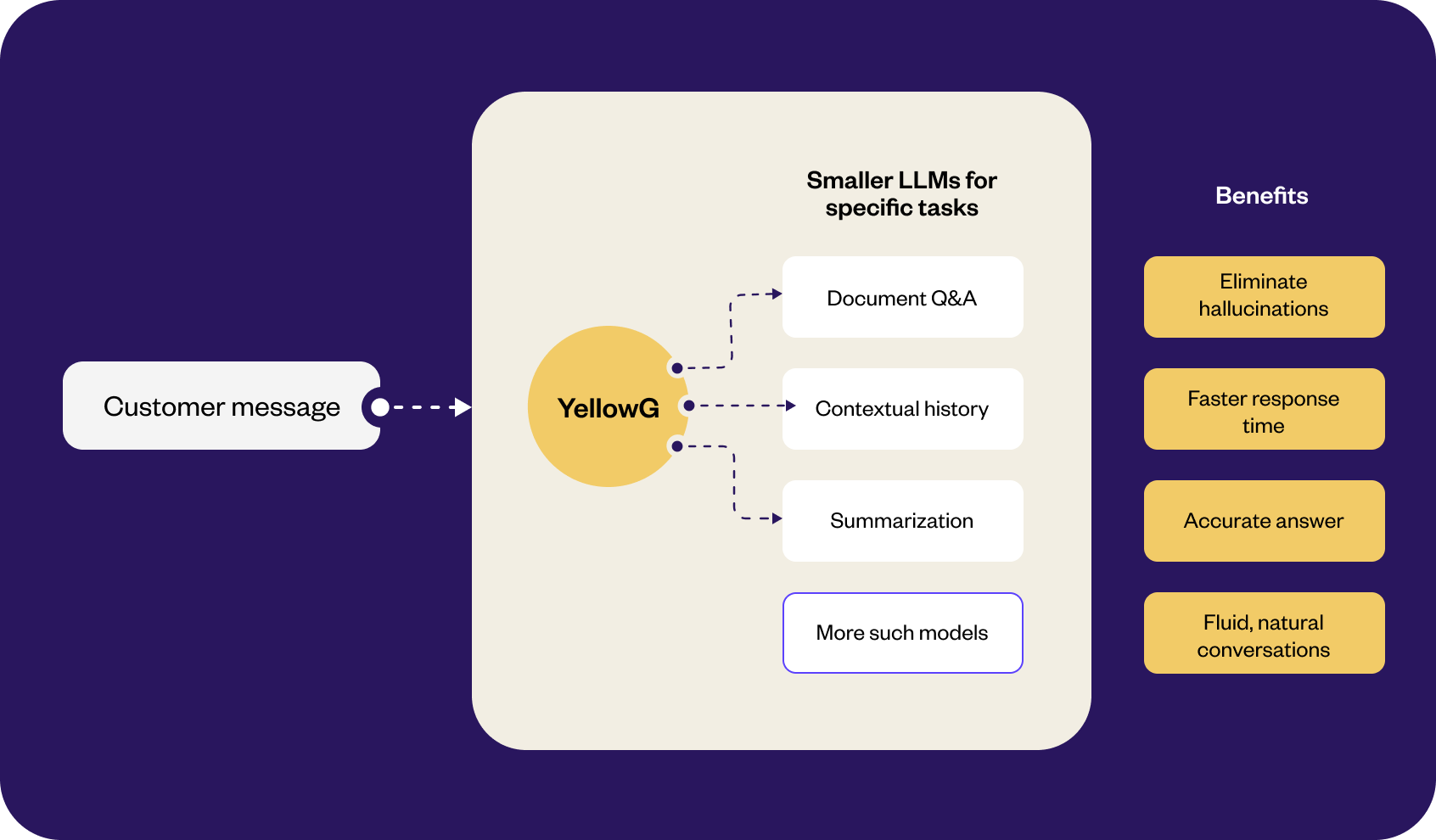
Bringing the best of both worlds, YellowG LLM with Generative AI uses multiple LLMs and proprietary insights to help businesses achieve highly personalized customer experiences.
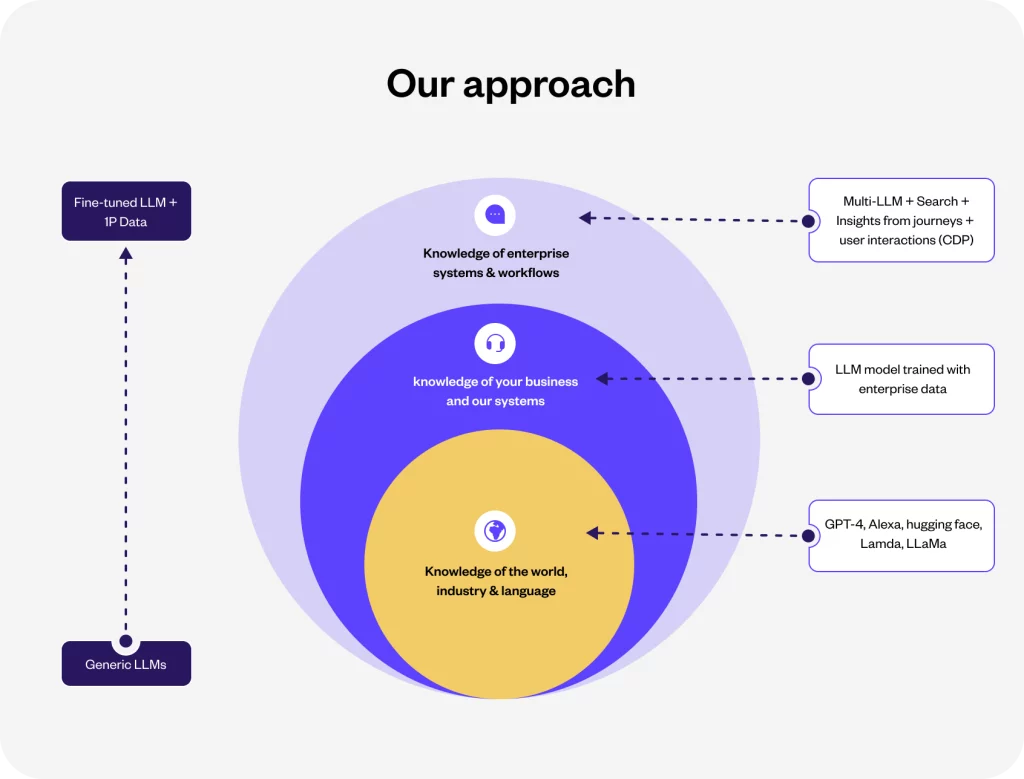
YellowG LLM combines the power of publicly available generic LLMs, specialized training on customer conversations, and integration with enterprise proprietary data to provide a comprehensive language model capable of delivering accurate, industry-relevant, and customer-centric solutions.
Comparative performance evaluation of language models
To gain a comprehensive understanding of the performance of different language models, we conducted an evaluation using a larger sample size. We took into consideration GPT-3 (Davinci), GPT-3.5 (Turbo), and YellowG LLM. Additionally, the assessment was carried out across two key parameters: hallucination rate and average response time.
1. Hallucination rate
For the GPT-3 model, the evaluation revealed a hallucination rate of 22.7%. This indicates that approximately 22.7% of the generated responses contained false or inaccurate information. For GPT-3.5, there was a reduction in the hallucination rate by 4.55%.
On the other hand, YellowG LLM showed a hallucination rate of nearly 0%, indicating that our in-house model consistently provided responses without any false or inaccurate information.
2. Average response time
The average response time for GPT-3 and GPT-3.5 was measured at 1.69 seconds and 1.34 seconds, respectively. This parameter indicates the time it took, on average, for the model to generate a response.
In contrast, YellowG LLM had a faster average response time of 0.6 seconds, making it highly efficient in generating prompt and accurate answers.
Here is the summary of the output
Parameter | GPT-3 (Davinci) | GPT-3.5 (Turbo) | Yellow.ai |
---|---|---|---|
Hallucination rate | 22.7% | 4.55% | Near 0% |
Average response time | 1.69s | 1.34s | 0.6s |
Let’s take a sample model comparison: Q&A
For better understanding, let’s take an example of the LLM for Q&A. The assessment was carried out to accurately answer questions from a given document. As mentioned above, the evaluation criteria included accuracy (hallucination rate) and speed of response provided by both the models, YellowG LLM and GPT-3.
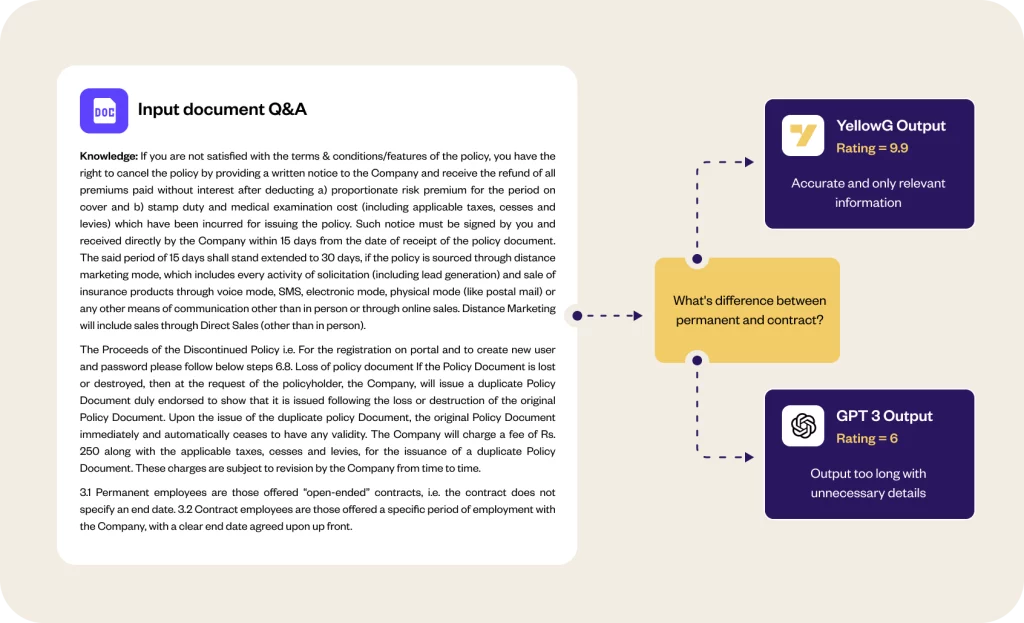
For this evaluation, both models were asked a specific question: “What’s the difference between permanent and contract?”
Answers from both models were analyzed and assessed.
GPT-3 | YellowG LLM |
---|---|
Rating = 6 Rationale = Output too long with unnecessary details | Rating = 9.9 Rationale = Accurate and only relevant information |
Response = Permanent employees are those offered “open-ended” contracts, i.e. the contract does not specify an end date. Contract employees are those offered a specific period of employment with the Company, with a clear end date agreed upon up front. If the policyholder wishes to cancel the policy, they must provide written notice to the Company within 15 days from the date of receipt of the policy document, or within 30 days if the policy was sourced through distance marketing. | Response = The main difference between permanent and contract employees is the duration of their employment contracts. Permanent employees have open-ended contracts without a specified end date, while contract employees have a specific period of employment with a clear end date agreed upon up front. |
The rationale behind this rating was that the generated output was excessively long and included unnecessary details that were not directly relevant to the question at hand.
On the other hand, YellowG LLM’s response was accurate and to the point. The output from YellowG LLM concisely explained the difference between permanent and contract employment. It clearly stated that permanent employees have open-ended contracts without a specified end date, while contract employees have a specific period of employment with a predetermined end date.
Key benefits of LLMs
1. Nearly 0% hallucination
By training smaller LLMs on relevant and specific datasets, YellowG LLM reduces the risk of generating wrong or nonsensical outputs by grounding itself within the provided enterprise-specific data. YellowG LLM refrains from delving into competition-specific information, ensuring that the provided answers align with your business goals and needs.
2. Faster response times
Because of the limited specific data on which YellowG LLM is trained, it delivers responses faster than some of the other widely used generalized models such as OpenAI GPT. YellowG LLM is thoughtfully built with streamlined architecture, focused training, and efficient computational needs, resulting in reduced response time.
3. Support customizations
YellowG LLM is an in-house proprietary model from Yellow.ai which makes it easy to fine-tune the LLM to meet specific enterprise needs. Our model is trained on billions of real-world conversations, which can then be enhanced with enterprise as well as domain-specific datasets. This improves the understanding of nuances and context that resonates with the brand of the business delivering personalized conversational experiences.
4. Ensure security and data privacy
With an in-house LLM, YellowG reduces the exposure of sensitive data such as PII to external LLMs. This means businesses have greater control over data privacy and security. Backed by ISO, HIPAA, SOC2, and GDPR certifications, YellowG is built to meet the highest security standards and compliance requirements.
Road ahead
These use-case-trained multi-LLMs are the future of Generative AI in the Conversational AI space. We have extensively developed complex end-customer-facing use cases across customer support, marketing, and employee experience within YellowG. Moreover, with YellowG LLM, we have been able to achieve real-time decision-making by leveraging LLMs in the runtime to redefine and elevate end-user experiences. We have widened the scope of bot responses and actions using Dynamic Workflow Generation that delivers individualized service through a combination of Customer Data Platform (CDP) and LLMs.
Discover the power of YellowG LLMs firsthand
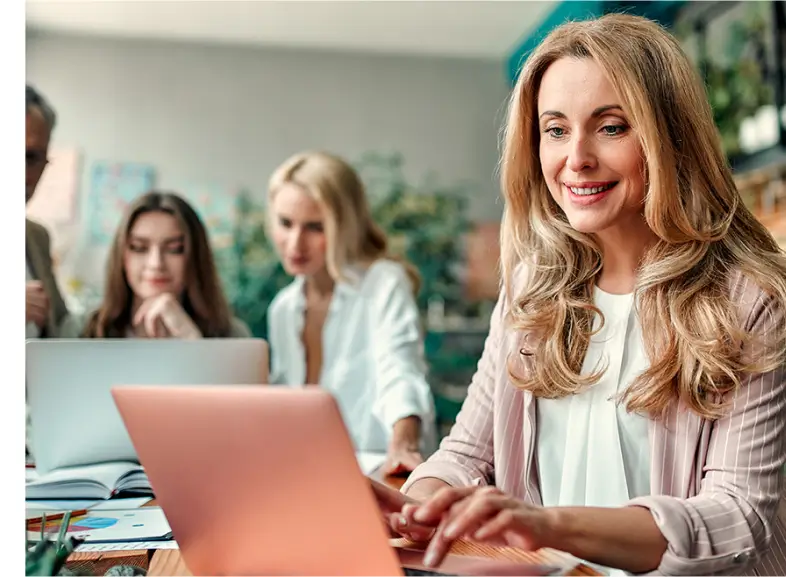