“Our human agents follow strict guidelines – how do we know AI agents won’t go off track?”
This question comes up in almost every conversation I have with enterprise leaders across industries. As generative and agentic AI move from experimental projects to large-scale mainstream deployments, preventing AI hallucinations has become a top priority for business leaders, as it should. When you’re handling tens of thousands of customer interactions daily, AI hallucinations may become a critical business risk.
As someone who’s spent the last several years building AI solutions for enterprise customer service, I have learnt valuable lessons about hallucinations, especially as generative AI reshapes the landscape. For enterprises, consistency and reliability are just as crucial as advanced capabilities – every response needs to be accurate, every time.
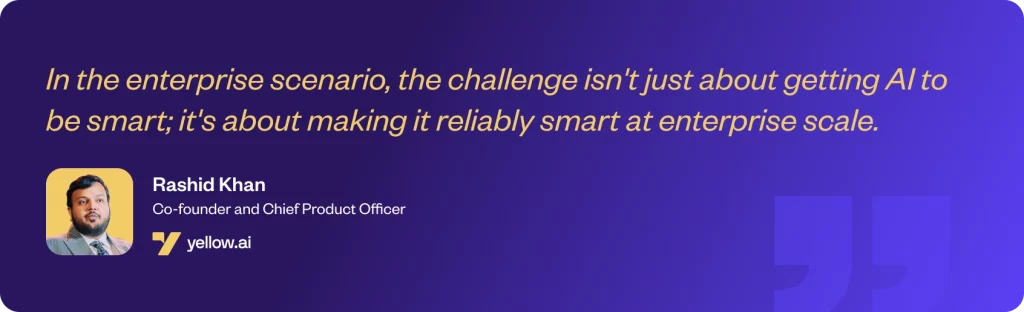
What we’ve learned at Yellow.ai, through extensive R&D, countless iterations, and real-world deployments, is that solving hallucinations for enterprise AI requires a fundamentally different approach. It’s not just about better models or more training data – it’s about understanding the unique demands of enterprise support and building safeguards from the ground up.
Let me share what we’ve learned along the way.
Understanding the Enterprise Challenge
During a recent conversation with a retail CX leader at an event, they shared their experience with their previous AI deployment: “We had to pull back our AI support after just two weeks because it started quoting incorrect return policies and making up discount offers, in about 1.35% of tickets. The cost of honoring these mistakes and managing customer complaints was far more than what we’d hoped to save.“
This highlights exactly why hallucinations in enterprise support are fundamentally different. It’s not just about accuracy – it’s about the scale of impact. When you are handling tens of thousands of conversations daily, even a small percentage of hallucinations can create significant business risk.
The enterprise context adds unique complexities – strict compliance requirements, frequent policy updates, complex customer journeys, and brand reputation at stake with every interaction. When AI agents represent your brand and handle customer queries, “mostly accurate” isn’t good enough.
Our Journey of Ensuring Reliability
Building AI that’s truly enterprise-ready has been an enlightening journey. Early on, we realized that traditional approaches to preventing hallucinations – like simply adding more training data or using larger language models – weren’t enough for enterprise scale.
What became clear through our work with enterprises was that the solution needed to be more systematic. It wasn’t just about making AI smarter; it was about building multiple layers of verification and control. Each customer conversation needed to be grounded in accurate, up-to-date information, with clear paths for handling uncertainty.
The breakthrough came when we started thinking about it from an enterprise architecture perspective – building safeguards and validation at every step of the conversation, not just at the response level.
How We Tackle Hallucinations & Build Enterprise-Grade Reliability in AI Agents
Let me break down the key challenges with AI hallucinations and how we address each one at Yellow.ai:
Challenge 1: Context & Intent Understanding
AI systems often hallucinate when they misinterpret user intent or lose track of conversation context. This is particularly risky in enterprise support, where conversations often involve multiple topics and complex queries.
Our Solution: Multi-step Evaluation Architecture
Our platform examines both user inputs and AI responses in real-time through multiple checkpoints:
- Input validation to understand user intent clearly
- Context verification to ensure response alignment with conversation history
- Policy compliance checks to maintain enterprise guidelines
- Response relevance scoring to guarantee answers match customer queries
Challenge 2: Uncertainty in Responses
Traditional AI systems often try to generate responses even when they’re uncertain, leading to made-up information. In enterprise support, this can create serious business risks.
Our Solution: Intelligent Fallback Handling
We’ve designed our AI agents to gracefully handle uncertainty. When a query is ambiguous or the confidence level isn’t high enough, the system:
- Executes predefined fallback flows to guide conversations back on track
- Provides relevant suggestions based on verified information
- Seamlessly transitions to human agents when needed
- Maintains context during handoffs to ensure a smooth customer experience
Challenge 3: Edge Cases & Unusual Queries
AI systems are prone to hallucinations when facing unexpected or complex questions they weren’t specifically trained for.
Our Solution: Comprehensive Bulk Testing
Before any of our AI agents go live, they undergo rigorous testing with real scenarios including:
- Simulation of thousands of real-world customer conversations
- Testing against historical support data
- Stress testing with edge cases and unusual queries
- Continuous monitoring and refinement based on performance metrics
Challenge 4: Data Accuracy & Currency
Hallucinations often occur when AI systems rely solely on their training data, which can be outdated or incomplete for enterprise needs.
Our Solution: RAG Integration
Our platform is built to ensure responses are always grounded in accurate data by:
- Pulling information from verified knowledge bases in real-time
- Updating responses based on the latest data
- Maintaining version control of information
- Ensuring responses are always grounded in accurate, current information
Challenge 5: Industry-Specific Complexity
Generic AI models often struggle with industry-specific terminology and requirements, leading to inaccurate responses.
Our Solution: Domain-specific Fine-tuning
Every industry has its unique requirements. Our platform is constantly trained on 16B+ conversations annually to ensure they are continuously getting better and our AI agents are fine-tuned with:
- Training modules built with sector-specific terminology and processes
- Built-in compliance checks for industry regulations and policies
- Customer journey mapping for specific business workflows
- Custom response templates designed for industry scenarios
Challenge 6: Response Generation Accuracy
AI systems can sometimes generate plausible-sounding but incorrect responses, especially in complex conversations.
Our Solution: Advanced Prompting Techniques
Our platform combines multiple prompting strategies:
- One-shot prompting for clear, direct instructions
- Few-shot learning with contextual examples
- Dynamic prompt adjustment based on conversation flow
- Industry-specific prompt engineering
Challenge 7: Added Complexity in Voice AI Agents
Voice interactions add another layer of complexity to preventing hallucinations. Speech recognition errors, real-time processing demands, and the need for natural conversation flow make voice AI agents particularly challenging.
Our Solution: Voice-specific Safeguards
The results we’re seeing from our voice AI deployments have been remarkable and particularly rewarding. When customers tell us they’ve never seen voice AI work this reliably at scale, it validates our approach to building enterprise-grade safeguards. Here’s what makes this possible:
- Real-time speech verification to ensure accurate interpretation
- Latency optimization for immediate response validation
- Natural language processing tuned specifically for voice interactions
- Voice-specific fallback mechanisms for uncertain responses
- Multi-step verification optimized for voice conversations
What This Means for Enterprises Like Yours
From our early days of building traditional conversational AI to today’s world of generative and agentic AI, one thing has remained constant at Yellow.ai – our understanding that enterprise AI support needs fundamentally different safeguards than consumer AI. Through each evolution of AI technology, we’ve continuously refined our approach to preventing hallucinations, building on lessons learned from thousands of enterprise deployments.
Here’s how this translates into real value for you:
Built-in Protection Against Hallucinations
Every Yellow.ai deployment starts with built-in guardrails. Our platform comes with predefined boundaries, clear escalation paths, and robust accuracy monitoring – all ready from day one.
Continuous Verification at Every Step
Our validation process is automatic and continuous. The platform tests every conversation, monitors responses across channels, and ensures compliance with your policies. You get reliability without the complexity.
Security That Doesn’t Cause Customer Friction
All our safety measures work behind the scenes. Your customers get smooth conversations, natural handoffs between AI and human agents, and consistent responses across channels – while our platform handles accuracy checks automatically through all the checkpoints in the background.
Designed to Scale With You
Start where you’re comfortable – whether that’s simple queries or complex interactions. Our platform grows with your needs in no time, maintaining accuracy even as you expand use cases and volume.
Let’s talk
As we, at Yellow.ai, continue pushing the boundaries of what’s possible with enterprise AI, I’m always keen to discuss how we can solve your specific challenges. If you are facing a unique challenge that’s stopping you from deploying AI at your enterprise, let’s discuss some possible strategies
Related read
Unpacking Common Concerns About AI Agents in Customer Service: A Strategic Guide
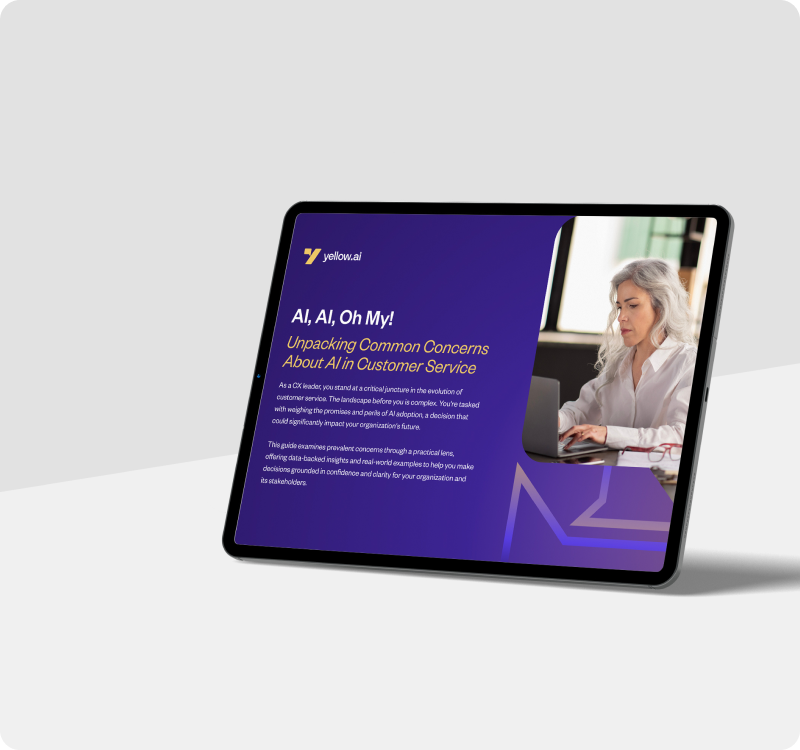