“What is generative AI and how does it work?” This question has now taken the limelight in the field of artificial intelligence. Generative artificial intelligence is a new but rapidly evolving branch of AI, and it’s not just another tech trend but a creator. With an uncanny ability to produce content that mirrors human creativity – from written words to images and even audio and video – it first caught the world’s attention with the launch of a consumer chatbot in 2022.
The impact of generative AI is quite significant and measurable. According to the economic potential of generative AI report 2023 by McKinsey & Company, generative AI could add a staggering $6.1 to $7.9 trillion annually to the global economy by boosting worker productivity. Gartner has also spotlighted generative AI in its Emerging Technologies and Trends Impact Radar for 2022, positioning it as a critical driver in the next wave of productivity advancements.
How to gain 1000% on ROI with Yellow.ai and Generative AI
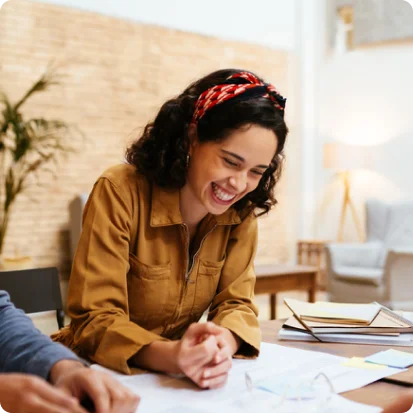
Previously, chatbots were entirely or largely rule-based, and due to that, they lacked contextual understanding. Their responses were limited to a set of predefined rules and templates. In contrast, the emerging generative AI models have no predefined rules or templates. Metaphorically speaking, they’re primitive, blank brains (neural networks) exposed to the world via training on real-world data.
Generative AI is an unfolding reality that commands our attention now. Understanding how does generative AI works is about more than just keeping pace with technological advancements. Instead, it’s about appreciating and harnessing a force reshaping the contours of productivity and creativity in our global economy. So, in this blog, we’ll embark on a journey to demystify this technological marvel. Whether you’re a tech enthusiast, a business professional, or just someone curious about the future of AI, you’re in the right place. Let’s dive into the world of generative AI and uncover its workings, applications, and potential to reshape our digital landscape.
What is Generative AI?
Generative artificial intelligence (GAI) is a transformative branch within AI, characterized by its ability to create new, realistic data that mirrors human-like patterns. These advanced AI models, trained on extensive datasets, go beyond mere data interpretation. They actively generate original text, image, audio, or video content using advanced deep learning and large language models (LLMs).
The uniqueness of generative AI lies in its ability to respond to a wide range of text prompts which can be from simple to highly complex, enabling interactions like conversing with a human. They’re changing the way we interact with machines and making computerized responses more like natural or human conversation. It’s blurring the traditional boundaries between human creativity and computer-generated outputs, and that’s genuinely exciting.
Most practiced applications of Generative AI
Understanding how generative AI works opens up various possibilities across various sectors. This transformative technology streamlines workflows for creatives, engineers, researchers, and more, transcending traditional industry boundaries. By converting inputs like text, images, audio, and video into new, innovative outputs, generative AI demonstrates its versatility and limitless potential. From text transforming into stunning visuals to melodies emerging from images, the applications of generative AI showcase its profound impact. Let’s explore some of its most prominent applications.
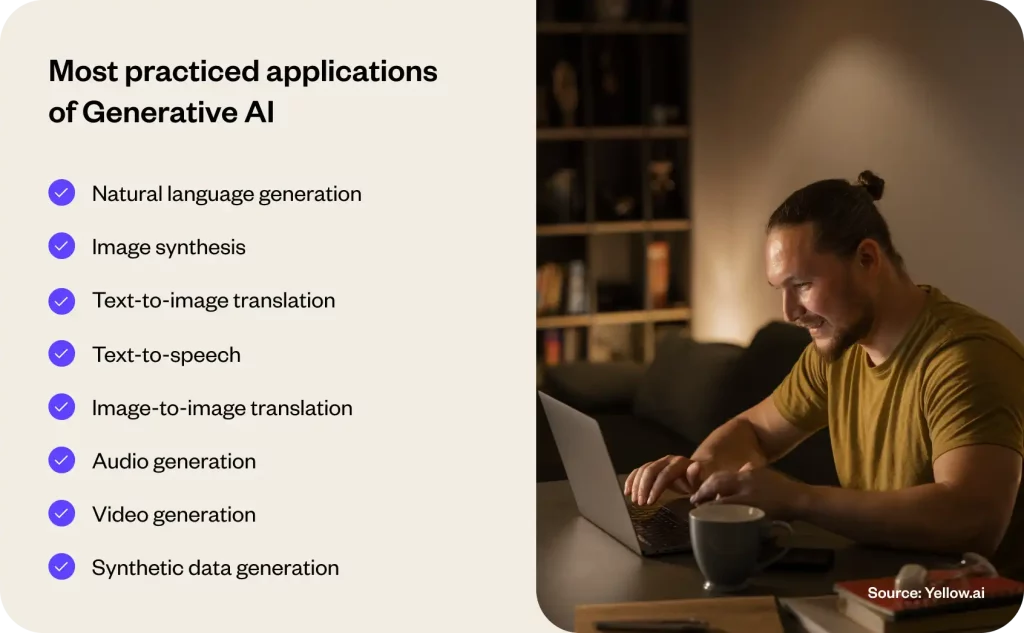
1. Natural language generation
Natural language generation (NLG), a pivotal application of generative AI, is revolutionizing how machines generate human-like text. It powers automated content creation in journalism, enhances interaction in chatbots and virtual assistants, and personalizes customer communication in marketing. By training on extensive text datasets, NLG models learn to mimic language patterns, enabling applications ranging from accurate language translation to creative writing aids.
2. Image synthesis
Image synthesis is transforming the way we create and interact with digital visuals. Used extensively in digital art and advertising, image synthesis enables generative AI models like GANs to create a wide array of images, from highly realistic to abstract designs. By learning from diverse image datasets, these models generate visuals that can encapsulate intricate artistic concepts or innovative advertising ideas, showcasing a blend of technological prowess and creative exploration.
3. Text-to-image translation
Text-to-image translation is a remarkable application where generative AI models convert written descriptions into visual images. This technology bridges the gap between verbal concepts and visual representation, enabling the creation of detailed, accurate images from simple text prompts. Widely used in creative fields, this application allows artists and designers to bring their written ideas to life visually. It also holds potential in educational tools, where complex concepts can be easily visualized, enhancing learning and understanding.
4. Text-to-speech
Text-to-speech technology transforms written text into spoken words, bridging the gap between written language and auditory communication. Essential in enhancing accessibility for visually impaired users, it converts digital text into audible speech. Additionally, it’s vital in developing interactive AI assistants and customer service bots, equipping them with natural-sounding, human-like voices. Recent advancements have led to voices that are more realistic than ever, making AI interactions more seamless and intuitive.
5. Image-to-image translation
Image-to-image translation is a fascinating application where generative AI models transform one image into another, altering its style or content while preserving its underlying structure. This technology is widely used in tasks like converting black and white photos to color, changing day scenes to nights, or even transforming sketches into detailed artworks. By understanding and manipulating the intricate features of images, AI models enable seamless and creative conversions, opening up new possibilities in visual editing and artistic expression.
6. Audio generation
Audio generation through generative AI is revolutionizing sound design and music production. This application involves creating new audio content, ranging from music tracks to sound effects, without direct human composition. AI models trained on diverse audio datasets can generate unique sounds and compositions, offering novel creative tools for musicians, filmmakers, and game developers. This technology is not just about automating music creation; it’s also about discovering new soundscapes and expanding the boundaries of auditory art.
7. Video generation
Generative AI in video generation not only reshapes content creation but also revolutionizes the backend process of video production. By analyzing and learning from extensive video datasets, these AI models understand motion, emotion, and visual storytelling patterns. They then use this knowledge to generate new video content, synthesizing frame-by-frame sequences that maintain continuity and realism. This involves intricate processing, such as predicting movement trajectories, rendering realistic textures, and ensuring lighting consistency.
8. Synthetic data generation
Synthetic data generation is a crucial application of generative AI, particularly valuable in scenarios where accurate data is limited or sensitive. AI models generate realistic but artificial datasets that mimic the statistical properties of actual data. This approach is extensively used in training machine learning models where privacy concerns or data scarcity pose challenges. Synthetic data helps in diverse fields such as healthcare, finance, and autonomous vehicle development, providing a safe and scalable way to test and train AI systems without compromising real-world data integrity.
Related read: 9 benefits of Generative AI in enterprises
What are the types of Generative AI models?
As we delve into the intricacies of generative AI, it becomes clear that a range of models is the foundation of this technology. Each model, from the pioneering neural networks to the sophisticated likes of GANs and transformers, brings a unique capability to the AI arena. Together, they not only define but also constantly redefine the capabilities of AI in mimicking and often surpassing human creativity and analytical skills. This section will take you through these critical models, highlighting their roles and evolution in shaping generative AI.
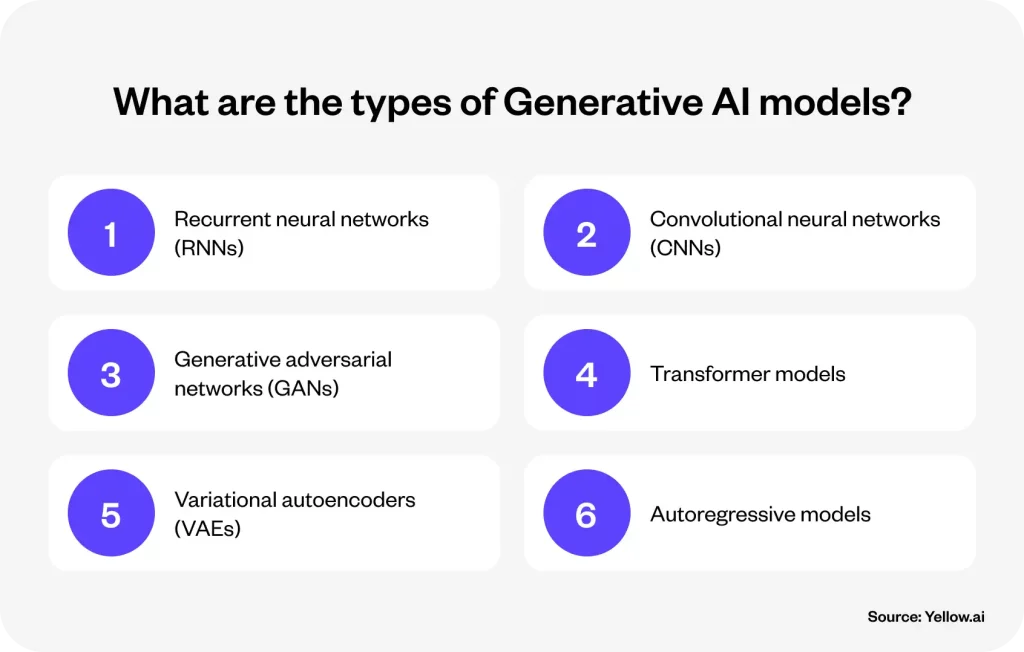
1. Recurrent neural networks (RNNs)
Recurrent neural networks (RNNs), first introduced in the mid-1980s, are specialized in processing sequential data, making them ideal for tasks where order and continuity are crucial, such as language processing, stock market analysis, and music generation. Central to various AI applications, from natural language processing (NLP) and speech recognition to financial forecasting and music-generating apps, RNNs excel in automating tasks that rely on the meaningful sequence of information. While they faced challenges with long sequences due to the vanishing gradient problem, advancements like Long Short-Term Memory (LSTM) and Gated Recurrent Units (GRU) have significantly enhanced their capabilities, solidifying their role in generative AI.
2. Convolutional neural networks (CNNs)
Convolutional neural networks (CNNs), which emerged in the late 1990s, revolutionized image processing and generation in generative AI. Mimicking the human visual cortex, CNNs excel in identifying and interpreting spatial patterns in images, making them invaluable for image classification, object detection, and generative tasks. Especially in text-to-image generation, as seen in tools like DALL-E and Midjourney, CNNs have demonstrated their prowess by turning textual descriptions into detailed, realistic images. This breakthrough has propelled AI into creative domains and democratized visual content creation, enabling anyone to bring their imaginative concepts to life.
While RNNs and CNNs maintain their utility in AI, evolving demands led to breakthroughs in more advanced models.
3. Generative adversarial networks (GANs)
Generative adversarial networks (GANs), known for their unique dual-network architecture, have significantly impacted various fields, especially image and video generation. A GAN comprises two neural networks, the ‘generator’ and the ‘discriminator,’ engaged in a continuous competitive game.
- Generator: It creates synthetic data from random noise, like images or sound.
- Discriminator: It judges whether this data is natural or artificial.
This ongoing contest pushes the generator to produce increasingly realistic content, constantly trying to outsmart the discriminator, which concurrently sharpens its ability to discern real from generated data. Originating primarily from CNN architectures, GANs can incorporate RNN or transformer variants, making them highly versatile in creating authentic and intricate content.
4. Transformer models
Transformer models, such as those in the GPT series, represent a significant evolution in handling sequential data, outperforming traditional methods like RNNs in many aspects. Their key strength lies in using attention mechanisms, which effectively model the relationships between elements in a sequence, be it text or other data types. This allows Transformers to process information in a massively parallel fashion, maintaining an understanding of sequence context without the sequential processing limitations of RNNs. This parallelizability and their capacity to manage long sequences make Transformers especially adept at generating coherent and contextually relevant text. Their efficiency and responsiveness are evident in applications like ChatGPT, which can rapidly and accurately respond to conversational prompts, making them a game-changer in natural language processing and various generative tasks.
5. Variational autoencoders (VAEs)
Variational autoencoders (VAEs) stand out in the generative AI landscape, particularly in image generation, due to their innovative neural network architecture and training processes. A VAE comprises two critical components: the encoder and the decoder.
- Encoder: It focuses on identifying key features and characteristics of data (like images), compressing this information into a condensed representation stored in memory.
- Decoder: It attempts to reconstruct the original data from this compressed form.
This dual process enables VAEs to learn probabilistic representations of input data and generate new samples that closely resemble their training data. Adaptable in their architecture, VAEs can employ RNNs, CNNs, or transformers, making them versatile for tasks beyond image generation, including text and audio applications.
6. Autoregressive models
Autoregressive models represent a fundamental approach in generative AI, particularly in natural language processing. These models generate data sequentially, one element at a time, by predicting each element based on the preceding ones. By leveraging probabilistic models, they are adept at tasks like text generation, language translation, and complex applications like stock price prediction. The key to their effectiveness lies in their training on extensive datasets, encompassing articles, books, and websites, enabling them to mimic and generate human-like text. Large Language Models (LLMs) like GPT and Google’s BERT have demonstrated remarkable proficiency in various language tasks, from machine translation to text summarization. Beyond practical applications, their ability to generate high-quality, creative content has paved the way for novel uses in chatbots, poetry, news articles, and social media content creation.
How does Generative AI work?
To truly understand the mechanisms behind generative AI, it’s essential to examine how it operates in various contexts. This section delves into the intricate processes that enable generative AI to analyze, learn, and create, transforming abstract data into tangible outcomes.
1. The neural network framework
Just like the human brain, which operates by continuously predicting and then learning from the differences between its predictions and reality, generative AI functions in a remarkably similar manner. All generative AI models begin with an artificial neural network encoded in software, startlingly acting as if they possess intelligence.
Visualize this neural network in generative AI as analogous to a 3D spreadsheet, where each ‘cell’ represents an artificial neuron, similar to neurons in the human brain. These neurons, organized in layers, are connected through formulas that define their interaction. The focus in AI isn’t merely on the number of neurons but rather on the connections between them, known as ‘weights’ or ‘parameters.’
These weights determine the strength of each connection and are fundamental to the AI’s learning and generative capabilities. In this framework, various neural network architectures play distinct roles: RNNs efficiently process sequential data for tasks like language generation, GANs with their dual-network setup excel in realistic image synthesis, and VAEs are pivotal in data encoding and reconstruction, similar to how the transformer architecture excels in large language models. These diverse architectures, each with its strengths, collectively contribute to generative AI’s almost intelligent-like ability to predict, learn, and create.
2. Data training and learning
The process of training generative AI models, particularly large language models, begins with exposing them to vast volumes of text. This initial stage involves the model making simple predictions, such as identifying the next token in a sequence. Tokens, rather than words, are the primary units of processing in these models. A token can represent a common word, a combination of characters, or even a frequently occurring sequence like a space followed by ‘th’.
During training, each token enters the neural network’s bottom layer and travels through the stack of neurons, with each layer processing and passing it upwards. The stack sizes vary but generally consist of tens of layers. Initially, the model’s predictions are rough, but the training involves a key process called ‘backpropagation.’ Here, every prediction, right or wrong, leads to adjustments in the model’s parameters or the coefficients in each neuron. This continuous adjustment aims to increase the accuracy of predictions over time.
Even correct predictions are refined – a 30% certainty might be nudged to 30.001%, enhancing the model’s confidence. After processing trillions of tokens, the model becomes adept at accurate predictions.
Post the initial training phase, generative AI models undergo fine-tuning through techniques like Reinforcement Learning from Human Feedback (RLHF). In RLHF, human reviewers assess the model’s outputs, providing positive or negative feedback, which is then used to further refine the model’s responses.
3. Generative AI in action
Generative AI’s real-world applications offer a tangible perspective on its capabilities and impact. A prime example can be seen in the case study of Pelago, a travel experiences platform reimagined through yellow.ai’s generative AI-powered conversational agents.
By implementing generative AI-powered conversational agents, Pelago enhanced its customer interactions, offering personalized and efficient service. These AI agents, trained on vast datasets of customer interactions, learned to understand customer inquiries accurately and provide relevant, context-specific responses. This application of generative AI showcases not just the automation of responses but also a deep understanding and anticipation of customer needs, leading to an improved overall customer experience.
Pelago reimagines customer experience with generative AI-powered conversational AI agents
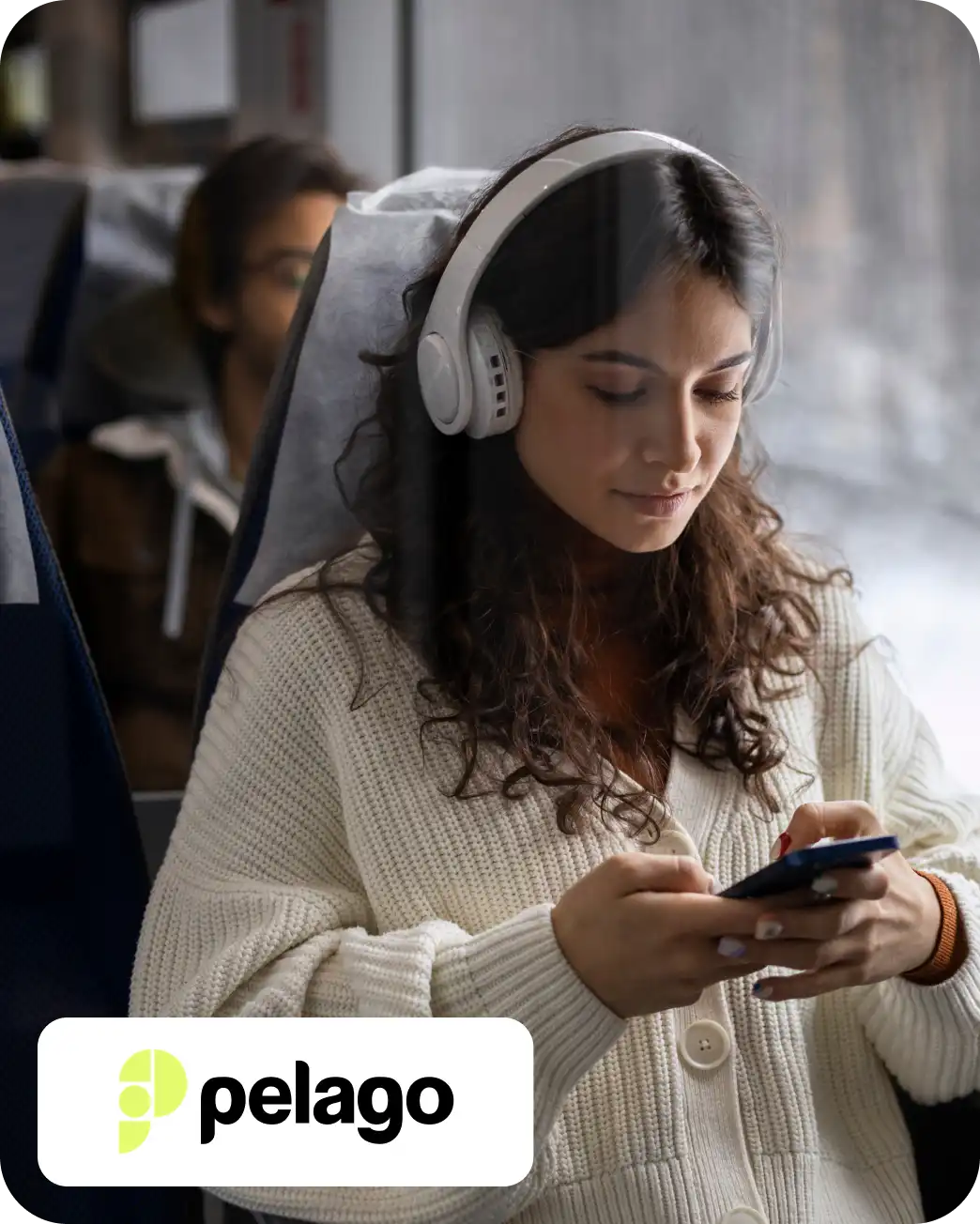
Generative AI models are adept at creating diverse content forms by identifying and applying patterns from their training data. Be it text composition, image creation, or audio synthesis, these models use learned data patterns to generate new, contextually relevant content. This ability to adapt and apply learned information is what makes generative AI a powerful tool in various fields, from customer service to creative arts.
The landscape of generative AI is continually evolving, with recent advancements enhancing its versatility and effectiveness. These include more sophisticated language models capable of understanding and generating a wide range of linguistic styles and image-generation technologies that produce increasingly realistic visuals. Such advancements not only demonstrate the growing intelligence and capability of AI systems but also open new possibilities for innovation across industries.
Conclusion: Embracing the future with Generative AI
As we conclude our deep dive into “how does generative AI work,” we stand at the threshold of a new era in technology, where artificial intelligence is a creative partner. This innovative branch of AI, with its remarkable ability to generate content that mirrors human creativity, is revolutionizing industries from journalism to design, and from customer service to entertainment.
The potential of generative AI extends far beyond current applications. Each advancement, from sophisticated language models to advanced image synthesis techniques, pushes the boundaries of what AI can achieve. It’s a journey of constant discovery, where each breakthrough reveals new ways for AI to enhance, augment, and even challenge our creative processes.
As we embrace generative AI, we embrace a future where the lines between human and machine creativity become increasingly blurred, opening up a world rich with unexplored potential. This technology is expanding the horizons of our imagination, promising a future where the synergy between human ingenuity and AI-driven innovation leads to unprecedented possibilities. In this evolving landscape, companies like yellow.ai are leading this transformation, leveraging generative AI-powered chatbots to offer personalized, dynamic customer experiences that redefine engagement and efficiency.