“Generative AI vs Predictive AI” are not just catchwords but pivotal forces driving innovation across diverse sectors, including healthcare, finance, and retail. As we stand at this crossroads, industry leaders, tech enthusiasts, and forward-thinking enterprises must grasp the distinct capabilities, applications, and implications of each.
Generative AI, celebrated for its ability to craft new and original content, is catapulting industries into new realms of creativity and innovation. Predictive AI, the seasoned strategist, leverages its analytical prowess to forecast future trends and behaviors, offering a strategic edge in decision-making.
In this comprehensive exploration, we’ll delve deep into the core of “Generative AI vs. Predictive AI,” dissecting their unique attributes, contrasting their trajectories, and highlighting how they are redefining the contours of the enterprise world. Join us on this enlightening journey through the cutting-edge landscape of artificial intelligence, where the potential is limitless, and the future implications are as profound as they are transformative.
What is Generative AI?
Generative Artificial Intelligence stands at the forefront of AI innovation, marked by its unparalleled ability to create new, realistic data that mirrors human-like patterns. This transformative branch of AI doesn’t just interpret data; it actively generates original content such as text, images, audio, and video. Trained on extensive datasets, gen AI uses advanced deep learning techniques and large language models (LLMs) to craft creations that push the boundaries of what machines can do.
Generative AI is a blend of algorithms and deep learning neural networks like Generative Adversarial Networks (GANs) and Variational Autoencoders (VAEs). These systems observe and learn from a plethora of content, understanding and replicating complex patterns and styles. This enables Generative AI to respond to a wide array of text prompts, from the simple to the highly complex, fostering interactions that are increasingly akin to conversing with a human.
What is Predictive AI?
Predictive Artificial Intelligence is a key force in AI, distinguished by its profound capability to analyze past and present data to forecast future events. This visionary branch of AI goes beyond mere analysis; it utilizes sophisticated models and algorithms to predict future trends, behaviors, and outcomes.
In a nutshell, It is a complex array of statistical models and machine learning techniques, including neural networks, decision trees, and regression analysis. These sophisticated systems carefully analyze and learn from past data, identifying patterns, irregularities, and connections. This capability allows Predictive AI to grasp and make sense of historical and current events and make educated forecasts. In doing so, it becomes a critical tool in the strategic decision-making arsenal.
Key differences between Generative AI and Predictive AI
Generative AI crafts novel content, including visuals, narratives, videos, and code, from simple prompts, embodying digital creativity. In contrast, Predictive AI excels in deciphering data patterns to forecast future trends, offering insightful predictions and outcomes.
Aspect | Generative AI | Predictive AI |
Definition | Generative Artificial Intelligence is an AI designed to create new and original content, including text, imagery, audio and synthetic data. | Predictive artificial intelligence is a subset of AI that predicts future outcomes for a company with the help of historical and current data. |
Primary function | Generative AI’s main function is to create entirely new and original data or content. It does this by learning from existing data and applying its understanding to generate fresh, unique outputs. This process is integral to fields where innovation is key, as it provides novel solutions and creations, from art and music to product design and beyond. | Predictive AI specializes in analyzing existing data to forecast future events or trends. Identifying patterns and correlations within historical information predicts what might happen next. This ability is crucial across various sectors, enabling more informed decision-making by anticipating future needs, behaviors, and risks. |
Technologies used | Generative AI utilizes advanced technologies like Generative Adversarial Networks and Variational Autoencoders. GANs involve two neural networks contesting with each other to generate new, authentic-seeming data, while Variational Autoencoders are about encoding data, tweaking it, and then decoding it to create new variations. These technologies empower Generative AI to produce highly innovative and diverse content, pushing the boundaries of creative possibility. | Predictive AI employs various technologies, including regression analysis, classification algorithms, decision trees, and neural networks. Regression analysis predicts continuous outcomes, while classification algorithms sort data into categories. The decision trees break down data into decision paths, leading to outcomes, and neural networks simulate human brain functions to find patterns and make predictions. Together, these technologies enable Predictive AI to analyze past data and predict future trends with remarkable accuracy. |
Data handling | Generative AI learns the intricate distributions and variations within input data to produce new content reflecting known patterns. This allows it to generate realistic and diverse outputs, from synthesizing speech to creating art, by mimicking the underlying structure of its training data. | Predictive AI examines past patterns and relationships within historical data to forecast future events and trends. Understanding these historical patterns provides valuable foresight, informing decision-making and strategy across various fields. |
Applications | Generative AI is widely used in creative domains, such as art and image creation, where it can produce unique visuals and designs. It’s also prevalent in text generation for things like storytelling or content creation, music composition, where it can create new melodies, and in synthetic data generation, which is crucial for training other AI models without the need for real, potentially sensitive data. | Predictive AI finds its applications in market forecasting, financial trends, and risk assessment, helping businesses understand potential future challenges. It’s also instrumental in predicting customer behavior and aiding in personalized marketing and sales strategies. In healthcare, predictive AI is used in diagnostic tools to anticipate medical conditions and outcomes. |
Output | The output of Generative AI is novel creations that closely resemble real-world data but are entirely new and unique. These can range from images that look like authentic photographs to text that reads as if written by a human and music that resonates with human compositions. The key is that while they mimic reality, AI completely generates these outputs, offering new and often innovative content. | Predictive AI produces outputs from predictions or classifications based on historical data analysis. It might forecast future market trends, predict customer behaviors, or anticipate possible health outcomes. The outputs are typically probabilities, trends, or categorical decisions, providing insight into what is likely to happen next based on past data. |
Complexity | Generative AI typically stands on the higher end of the complexity spectrum. It requires substantial computational power and sophisticated algorithms to perform its tasks. Learning patterns and creating entirely new, realistic outputs is computationally intensive. Technologies like GANs and VAEs, commonly used in Generative AI, involve complex interactions and training processes that demand robust hardware and advanced algorithmic understanding. | The complexity of Predictive AI can vary widely depending on the application and the specificity of the prediction required. Some models, like linear regression, can be relatively simple for forecasting sales in a predictable market. Others might be more complex, utilizing deep neural networks to analyze vast datasets with numerous variables, like predicting consumer behavior or complex financial trends. |
Real-world example | Generative AI is revolutionizing the art world by creating new artworks that blend different styles and eras. It designs virtual models and innovative clothing in fashion, streamlining the creative process. In entertainment, it’s creating realistic video game environments, and in healthcare, it’s generating synthetic data for research without compromising patient privacy. These diverse applications highlight its ability to enhance creativity and innovation across various industries. | Predictive AI is vital in business for predicting customer churn and helping companies retain their client base. It forecasts stock market trends in the financial sector, aiding investment strategies. It’s also transforming agriculture by predicting crop yields and detecting diseases, and in urban planning, it optimizes traffic flow and city layouts. These examples demonstrate Predictive AI’s wide-ranging impact, from enhancing business strategies to improving quality of life. |
Advantages | Generative AI is a powerhouse of innovation, capable of creating new content, especially useful where data is scarce or sensitive. It generates synthetic data for healthcare research and advances security systems, all while fostering creativity and ensuring privacy. This makes it invaluable for pushing boundaries and inspiring new solutions across various industries. | Predictive AI acts as a crystal ball for data-driven decisions, anticipating future trends based on historical patterns. Its wide applicability, from market forecasting to personalized customer experiences, makes it an invaluable tool across sectors. Predictive AI helps shape a smarter, more prepared future by enabling efficient resource allocation and proactive strategies. |
Limitations | While Generative AI is a marvel of innovation, its outputs can sometimes be unpredictable and not always align with expectations, leading to quality and relevance issues. Furthermore, it raises significant ethical concerns, particularly with the creation of deepfakes and the potential for spreading misinformation. These aspects pose challenges regarding trust and the responsible use of technology, requiring stringent measures to ensure its advancements are used ethically and constructively. | The effectiveness of Predictive AI is inherently limited by the quality and quantity of the input data it receives. Poor or biased data can lead to inaccurate predictions, potentially reinforcing existing prejudices or misconceptions. This limitation highlights the need for comprehensive, unbiased datasets and continuous monitoring to ensure that Predictive AI’s insights are as accurate and fair as possible. |
Future trends | Generative AI is set to revolutionize creative fields, data augmentation, and AI training, offering innovative tools for artists and comprehensive data enhancement. It’s crucial for synthetic data creation, maintaining privacy without compromising quality, signaling a move towards more secure, creative, and ethical AI applications. As technology advances, expect to see Generative AI permeate more sectors, fostering innovation and new solutions. | Advancing towards sophisticated real-time analytics and deeper IoT integration, Predictive AI is gearing up for smarter, quicker predictions across daily life and various industries. This integration promises enhanced efficiency in sectors like energy, traffic, and public health management, making Predictive AI’s insights more immediate and actionable. The future points to Predictive AI becoming an indispensable tool for dynamic, informed decision-making. |
Ethical considerations | Ethical concerns with Generative AI primarily revolve around authenticity issues and potential misuse. As it becomes increasingly adept at creating realistic content, distinguishing between what’s real and AI-generated becomes challenging. This capability raises concerns about creating false information or deepfakes, which can be used in misleading or harmful ways. Ensuring responsible use and developing robust verification methods are essential to address these ethical challenges and maintain trust in digital content. | Predictive AI’s ethical considerations are deeply tied to privacy and the potential for inherent biases. As it relies on vast amounts of personal and behavioral data to make predictions, ensuring the confidentiality and security of this data is crucial. Additionally, if the input data contains biases, Predictive AI can inadvertently perpetuate and amplify these biases in its decision-making, leading to unfair or discriminatory outcomes. Continuous monitoring, transparent methodologies, and inclusive data practices are necessary to mitigate these risks and ensure Predictive AI’s ethical application. |
Generative vs Predictive AI for optimal application
Identifying the most favored applications of Generative and Predictive AI is key to leveraging their potential effectively. This section delves into where each technology excels, providing insights into their optimal use cases. We’ll explore the scenarios where Generative AI’s innovative capabilities shine and where Predictive AI’s foresight is most valuable, guiding you towards strategic and informed application in your endeavors.
Generative AI
1. Innovation and creativity:
Generative AI excels in creating new content like art, music, and design, offering unique solutions where traditional data is scarce. It propels sectors forward by enabling artists and innovators to explore uncharted creative territories, turning abstract concepts into tangible realities and pushing the boundaries of conventional creativity, making it a cornerstone in fields that thrive on innovation.
2. Data augmentation:
Generative AI is invaluable for data augmentation and creating synthetic datasets where real data is limited, or privacy is a concern. It enhances AI training by providing diverse, realistic scenarios and improving model robustness without compromising sensitive information. This capability is crucial in healthcare, finance, and any domain requiring extensive data for accurate, reliable AI models, ensuring better performance and ethical data usage.
3. Flexibility:
Generative AI’s flexibility allows the exploration of hypothetical scenarios, making it indispensable for research and development. It can simulate environments, forecast potential outcomes, and provide visualizations for situations that don’t yet exist, aiding in everything from urban planning to climate change modeling. This adaptability makes it a powerful tool for innovators looking to address complex, future-facing challenges across various sectors.
Pelago reimagines customer experience with generative AI-powered conversational AI agents
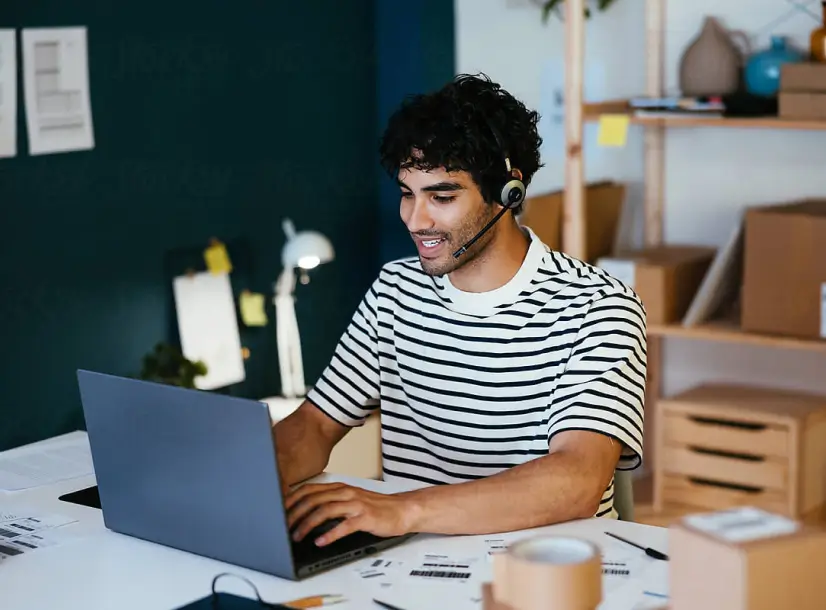
Predictive AI
1. Decision-making and forecasting:
Predictive AI is pivotal in decision-making and forecasting, analyzing historical data to predict future trends. It’s invaluable in market forecasting, risk assessment, and customer behavior prediction, turning data into actionable insights. Anticipating what’s next empowers industries to strategize proactively, reducing uncertainty and optimizing outcomes in dynamic environments.
2. Wide range of applications:
With its versatility, Predictive AI is crucial across sectors, from finance to healthcare. It deciphers data patterns to inform decisions, improving financial strategies, enhancing patient care, and personalizing customer experiences. Its ability to adapt to various data types and contexts makes it an essential tool for any data-driven organization seeking to leverage insights for a competitive edge.
3. Real-time analysis:
Predictive AI thrives in real-time analysis, which is essential for immediate decisions in systems like financial fraud detection or IoT environments. It processes streaming data to provide instant insights, enabling rapid response to changing conditions. This capability is critical in maintaining security, optimizing operations, and ensuring efficiency, marking it as a linchpin in modern, fast-paced decision-making scenarios.
Benefits of leveraging Generative AI with Yellow.ai
Embracing Yellow.ai’s generative AI-powered chatbots transforms business-customer interactions, offering advantages that enhance efficiency, engagement, and satisfaction. This section explores the key benefits of integrating these advanced AI solutions, highlighting how they revolutionize communication and operational strategies.
Transformative customer interactions
Yellow.ai’s generative AI chatbots revolutionize customer service by delivering instant, context-aware responses. This dramatically improves response times and resolution rates, fostering customer satisfaction and loyalty.
Insight-driven performance
Beyond responding, these chatbots analyze interactions to provide insights and suggestions, enhancing agent performance and productivity. Automating routine tasks allows human agents to concentrate on complex issues, optimizing workflows and reducing support costs.
Marketing and engagement
The chatbots excel in creating personalized, dynamic content for marketing and engagement, resonating with individual customer preferences. This ensures that each interaction isn’t just reactive but also deeply engaging, enhancing every customer’s experience with the brand.
Operational efficiency
With the ability to handle numerous interactions simultaneously, Yellow.ai’s chatbots significantly boost operational efficiency. They free up valuable human resources, reduce overhead costs, and improve service delivery, contributing to a healthier bottom line.
Empathy at scale
Generative AI enables these chatbots to interact with empathy and understanding, ensuring every customer feels heard and valued. This elevates the customer experience and strengthens their relationship with the brand, providing a competitive edge in the market.
Continuous improvement
Leveraging generative AI capabilities, Yellow.ai’s chatbots continually learn and adapt from each interaction, ensuring they grow more effective over time. This continuous improvement cycle promises a future of ever-more personalized and efficient customer service.
Conclusion
As we’ve journeyed through the intricate landscape of “Generative AI vs Predictive AI,” it’s clear that these technologies are not mere facets of innovation but are fundamental forces reshaping industries. Generative AI, with its unparalleled creativity and innovation, is transforming the way we conceive and create, from arts to complex data solutions. Predictive AI, on the other hand, is revolutionizing decision-making processes by providing foresight and precision like never before, from market trends to real-time analytics.
The synergy between these AI subsets signifies a leap toward a future where interactions, decisions, and creations are automated, deeply intelligent, and insightful. Leveraging Generative AI through Yellow.ai’s chatbots, businesses can expect a transformative shift in customer engagement, operational efficiency, and overall satisfaction. Meanwhile, Predictive AI continues to underpin strategic decisions across sectors, offering a clarity that drives progress and innovation.