As businesses continue to expand their online presence, chatbots have become increasingly popular as an efficient tool for customer service. Chatbots can help answer customer queries and provide support 24/7, without the need for human intervention.
One key component of an effective chatbot is a decision tree, which helps guide the bot’s responses based on the user’s input. In this blog post, we’ll discuss what a chatbot decision tree is, why it’s important, and how to create one.
Related must-reads:
- 9 Best chatbot examples for businesses by industry [2023]
- How to create a chatbot – Best practices to follow in 2023
- Future of Chatbots: Key Trends to Watch in 2023!
- Enterprise Chatbot – A Guide for Enterprises [2023]
- Customer service chatbots: Benefits and examples [2023]
What is a Chatbot Decision Tree?
A chatbot decision tree is a flowchart-like structure that maps out the conversation flow between a user and a chatbot. It’s a series of predetermined questions and answers that help the chatbot understand the user’s needs and provide the appropriate responses.
The decision tree is created based on a set of rules, which are predetermined based on the user’s potential questions or actions. The chatbot uses the decision tree to navigate through the conversation, providing accurate and relevant responses to the user.
Today, Chatbot Decision Trees are highly interactive and user-friendly. They can be designed to mimic human conversation and provide a seamless experience for users. Decision trees are no longer limited to just text-based chatbots—they can also be used for voice-activated assistants such as Amazon’s Alexa or Google Assistant.
The evolution of chatbot decision trees has been significant in recent years. Here are some key differences between then and now:
Then | Now |
Rule-based and limited in their ability to understand natural language or provide personalized responses | Powered by advanced NLP and machine learning algorithms, allowing bots to understand complex queries and provide personalized responses |
Simple and linear, providing customers with limited options for responses | Sophisticated, providing customers with multiple options for responses, allowing for more complex interactions |
Not scalable to be adopted across different industries, use cases and channels | Scalable and cost-effective way to automate routine tasks and improve customer interactions |
Importance of Chatbot Decision Tree
Consistency: Chatbot decision trees ensure that responses to customers are consistent and accurate, regardless of the time of day or the customer service representative handling the query. This helps to build trust and credibility with customers, as they know they can rely on the chatbot to provide accurate and consistent information.
Efficiency: Chatbot decision trees can automate routine and repetitive tasks, allowing customer service representatives to focus on more complex queries that require human intervention. This can save time and reduce costs for businesses.
Scalability: Chatbot decision trees can handle a large volume of customer queries simultaneously, making it possible for businesses to handle spikes in demand without needing to hire additional staff.
Accessibility: Chatbot decision trees can provide 24/7 support to customers, making it possible for customers to get assistance outside of business hours. This is especially important for businesses with a global customer base.
Personalization: Chatbot decision trees can be designed to personalize interactions with customers by using data to tailor responses to the individual’s preferences and history. This can help to improve customer satisfaction and retention.
Overall, chatbot decision trees are an important tool for businesses looking to improve customer interactions, reduce costs, and improve efficiency. By automating routine tasks and providing consistent and accurate responses, chatbot decision trees can help businesses provide a better customer experience and gain a competitive advantage.
Read more: Conversational AI – A complete guide for [2023]
How to create a Chatbot Decision Tree?
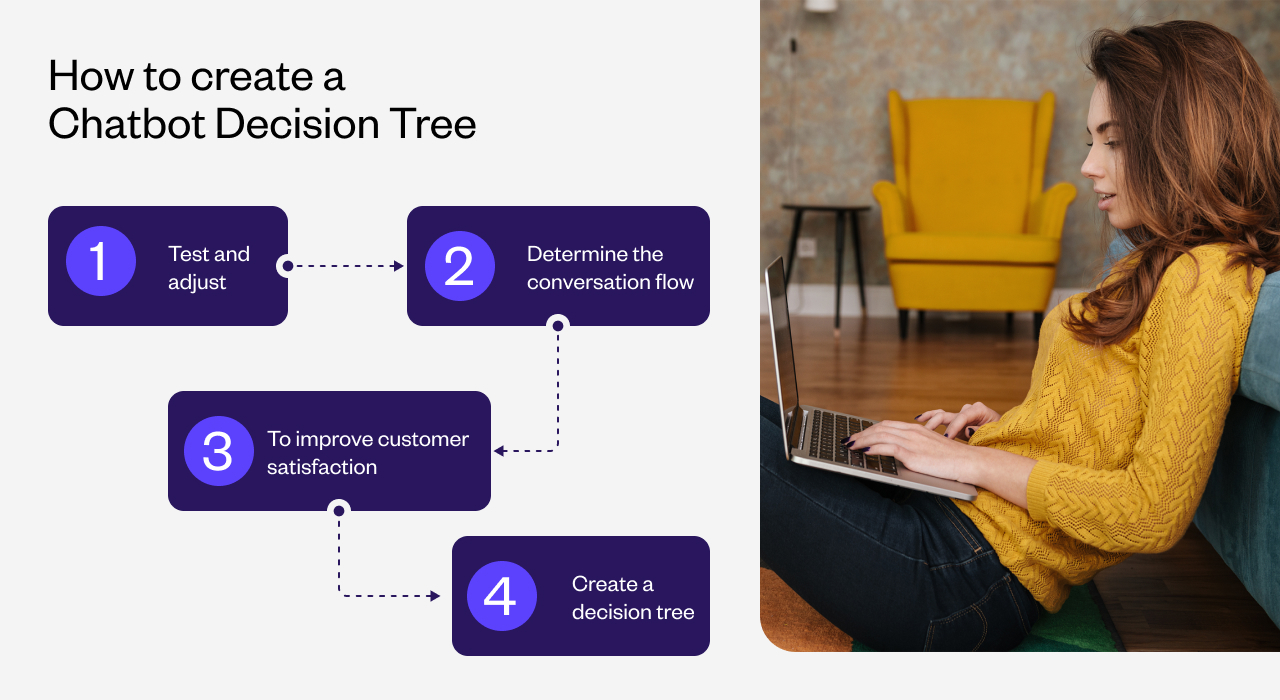
STEP 1: Identify the user’s potential questions or actions
Start by listing all the potential questions or actions a user may take when interacting with your chatbot. This can include frequently asked questions, common issues, or specific requests.
STEP 2: Determine the conversation flow
Once you have identified the potential questions or actions, map out the conversation flow based on a set of rules. Start with a broad question and then narrow it down to more specific questions to help guide the user toward the appropriate response.
STEP 3: Create a decision tree
Using a flowchart-like structure, create the decision tree based on the conversation flow determined in Step 2. Each branch of the decision tree should represent a potential question or action by the user, with the responses determined by a set of rules. Ensure that the decision tree is easy to navigate and understand.
STEP 4: Test and adjust
Once the decision tree is created, test it out to ensure that it’s working as intended. Test it with a group of users and make any necessary adjustments based on their feedback. This will help ensure that the chatbot is providing accurate and relevant responses to the user’s queries.
By following these simple steps, you can create an effective Chatbot Decision Tree that guides users toward the appropriate response and enhances their overall experience.
Tips to consider when creating your Chatbot’s Decision Trees
When creating your chatbot decision tree:
- Keep it simple: Avoid creating a decision tree that’s too complex or difficult to follow. Stick to a simple flowchart-like structure that’s easy for the user to navigate.
- Use clear and concise language: Make sure your questions and responses are written in clear and concise language that’s easy for the user to understand.
- Provide options: When creating your decision tree, provide the user with options to choose from to help guide them toward the appropriate response.
- Test and adjust: Test your decision tree with a group of users to see if it’s working as intended. Make any necessary adjustments based on user feedback.
Incorporating voice into your Chatbot Decision Tree
Chatbot Decision Trees and voice bots share some similarities in terms of their underlying logic and branching structure. While it is possible to design a decision tree that can be used with both text and voice interfaces, there are some important differences to consider. For example, voice bots may need to be programmed to recognize different accents and pronunciations, while chatbots may need to be programmed to handle typos and misspellings.
In general, designing a successful chatbot or voice bot requires careful consideration of the user experience and the specific needs and preferences of the target audience.
Here are a few things to consider:
Voice user interface (VUI): Voice bots use a VUI to interact with users through spoken commands and responses. This means that the decision tree will need to be designed to accommodate voice inputs and outputs.
Speech recognition: In order for the voice bot to understand user inputs, it will need to be equipped with speech recognition technology. This means that the decision tree will need to be programmed to recognize a variety of spoken inputs and respond accordingly.
User testing: Once you have built your voice bot decision tree, it is important to conduct user testing to ensure that the VUI and speech recognition are working properly and that users are able to navigate the decision tree effectively using voice commands.
Takeaway
In conclusion, Chatbot and Voice bot Decision Trees are powerful tools that can be used to improve customer service by automating routine tasks and providing personalized experiences to customers across a wide range of industries and applications.
By guiding users through a series of questions and responses, decision trees can help to automate customer support, e-commerce, healthcare, and education services. Decision trees can be designed to handle a wide range of inputs and provide appropriate responses based on user preferences and history.
What is a chatbot decision tree?
A chatbot decision tree is a flowchart-like structure that maps out the conversation flow between a user and a chatbot. It’s a series of predetermined questions and answers that help the chatbot understand the user’s needs and provide the appropriate responses.
What is an example of a decision tree?
Here’s an example of a decision tree for a chatbot that helps users in deciding whether to buy a new car or not.
In this decision tree, the first question is whether to buy a new car. If the answer is “Yes,” then the next question is whether the budget is limited. If the budget is limited, the options are to buy a sedan or an SUV. If the budget is not limited, the options are to buy a luxury sedan or an SUV. If the answer to the initial question is “No,” then the next question is whether to keep the current car or buy a used one. If the decision is to buy a used car, then the options are to buy a car or a truck.
What are the KPIs to measure the success of a chatbot?
Here are some common KPIs for chatbots:
- Conversation Completion Rate: This KPI measures the percentage of conversations that are successfully completed without any user drop-offs. It indicates how well the chatbot is able to engage users and provide them with useful information.
- Average Handling Time: This KPI measures the average amount of time it takes for the chatbot to handle a user’s request or question. A low average handling time indicates that the chatbot is efficient and able to provide quick and accurate responses.
- User Satisfaction: This KPI measures the user’s satisfaction with the chatbot’s performance. It can be measured through surveys, feedback forms, or other forms of user feedback.
- Accuracy: This KPI measures the accuracy of the chatbot’s responses. It indicates how well the chatbot is able to understand the user’s intent and provide relevant and accurate information.
To measure the success of a chatbot, track these KPIs over time and set specific goals for each one. Conducting user testing and surveys to gather feedback from users and identify areas for improvement also helps to improve the chatbot’s performance.
What do you mean by “chatbot accuracy”?
The accuracy of a chatbot refers to how well it is able to understand the user’s intent and provide relevant and accurate responses. It is typically measured as a percentage of the chatbot’s total responses that are correct. A high accuracy rate indicates that the chatbot is able to effectively understand and respond to user requests, while a low accuracy rate indicates that there may be issues with the chatbot’s natural language processing or decision-making algorithms
Want to know more about no-code platforms for designing and building AI-powered bots? Learn more about Dynamic Conversation Designer here.