Transcript
Tara Shankar – 00:00:03:
Generative AI takes the center stage. But is your enterprise still watching from the sidelines? Come on in, let’s fix that. This is Not Another Bot, the generative AI show, where we unpack and help you understand the rapidly evolving space of conversational experiences and the technology behind it all. Here is your host, TJ. Hello and welcome to Not Another Bot, the General AI Show. I’m your host, TJ. Joining me today, Surbhi Rathore. She’s the CEO and co-founder of Symbol.AI. You might have seen a lot on this news so far. A pioneering machine learning startup that’s transforming how businesses communicate with their products and solutions. With a wealth of experience, starting from her days as a software developer in India to leading an innovative technology company, Surbhi has been at the forefront of AI-driven customer experience solutions. As an advocate for immigrant founders and a believer in the power of borderless collaboration, she’s not just breaking boundaries, but also redefining them. It’s a privilege to have her with us to share her insights and experiences on AI, machine learning and customer experiences. Welcome, Surbhi, pumped to have you here.
Surbhi Rathore – 00:01:11:
Thank you so much. Yeah, I’m so excited to be here. Oh my God, those were really nice and heavy words. I hope I live up to that for the next 20, 30 minutes here.
Tara Shankar – 00:01:20:
You will 100% for sure. All right, Surbhi, it’s always something which we start with is to know more about you. So could you share a bit more about your journey, going all the way, being a software engineer and becoming the CEO and co-founder of Symbol.AI. What were the pivotal decisions and experiences that led you here?
Surbhi Rathore – 00:01:39:
Yeah, so I did my engineering in India. I was born and brought up there. My dad was in the armed forces, so had the privilege of actually spending time at multiple locations and states and getting thrown into a new group of friends every two years. So that kind of a figure-it-out thing, I think, runs in the DNA of the family. Actually, my dad also worked in communications. So communications is pretty core to my upbringing as well in his armed forces career. Started in India, joined a startup right out of college and worked and built their software systems that was a lot in network security. And then eventually two years later joined a company called Amdocs. Where I spent the majority of time building products and experiences, specifically for customer experience products that Amdocs is building, and worked with large Telcos. And got an opportunity to spend like seven-plus years in actually figuring out the little changes that can make massive impacts when the volumes of conversations are so high. And was a big part of bringing their conversational AI practice and product that they were launching before starting Symbol and the journey from figuring out and building the tech, which is scalable for Telcos to automate human conversations and replace them with bots and virtual assistants to now with Symbol in trying to do, started with augmentation, but I think eventually both the worlds, merged together with each other and one system should be able to do the both. Kind of that’s where we are all leading right now, but yeah, it’s been an exciting journey. From India to Montenegro for a while, to Sydney, to other places, and then finally San Jose and then Seattle, and now back to SF. So. Which is why I don’t understand the borders and the boundaries. And just like, I love interacting and working with people across different cultures. It brings such a diverse perspective to the way that we build products. So yeah, here I am now. I’m dialing in today from Seattle, although I live in SF now.
Tara Shankar – 00:03:39:
Brilliant. There’s so many similarities. My dad was in defense too. He was in Air Force back in India. I lived in Melbourne too, for like five years. I live in Seattle now. So thanks so much for that introduction. Now looking at the passion I’ve had, or you have for AI and you know, soft engineering, and the fact that you have also been an advocate for immigrant founders, how has your own background as an immigrant shaped your approach to entrepreneurship and leadership in the steak industry?
Surbhi Rathore – 00:04:10:
Yeah, this whole, in India there’s a word called jugaad. I don’t know like how much other people would know about it, but definitely search it. I think most of the immigrants and eventually leading up to immigrant founders, like the whole figure it out attitude and like don’t give up. Even if you have limited resources, like of course you won’t be entitled to the best. So figure out what you have and you don’t make your mark there. So of course there’s a little bit of more gratefulness and grit towards building the company, which I feel is so critical. Especially me being a first-time founder, it really shapes the way that we have put ourselves out there. Sometimes more than what we are supposed to, but at the end of the day, it’s the journey that matters. So yeah, we are very grateful for the community that has worked with us. We really embrace that. And that also shaped the huge developer community that symbol champions and supports through its products, the humbleness that we have for other startups. Because we, being a startup ourselves, a lot of companies supported us with like free credits and freebies and like all of that at the beginning when we were bootstrapping the company until we raised our first round. So to give back, we also run a startup program where we give credits back to startups where they can come and build with us. So I think the journey on how you build a company really shapes the way that you provide for your customers, partners, and core values of the company that you strengthen upon. So yeah, a lot of that you will see in the way that we are building Symbl.

AI in itself is not explainable completely, whether it’s deep learning models, whether it is now large language models, even more. And predictability is crucial for enterprise adoption because they want to make sure that when they apply technology to solve a problem, it can predictably do X things with X, Y percentage of accuracy or precision. Knowing that is more important.

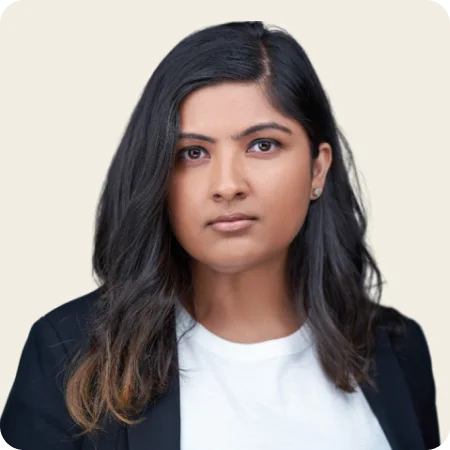
Surbhi Rathore
CEO and Co-founder of Symbl.ai

Tara Shankar – 00:05:47:
Absolutely, Brun. Another thing we know more about you, and we have been duets, we have been looking at how your journey has been. We see you have several patents under your name, and that’s massive and exciting too at the same time. Can you tell us more about the inspiration behind those patents and how they integrate into your work at Symbol.ai?
Surbhi Rathore – 00:06:06:
Yeah. So when we started the company back in 2018, a lot of the meeting, conversation analysis and all that kind of stuff revolved around a bot-like experience. Like you speak to the bot, take an action item, then the BOT takes the action item. There were very, a lot of early companies like that. And then a lot of non-bot experiences centered more around recordings and how to review recordings in the best way and how to get access to recordings. When Symbol started, we wanted to kind of like break the barriers between the both and said, well, conversation data is just like another form of data that should be analyzed and converted into structured insights, building specialized models and not by building general models. And some people took that even one further step away that, you know, I’m going to build something for only customer service conversations and customer support conversations. And businesses that have capital can really invest and build like, you know, spend one-third or one-half of the company spend to building that AI strategy. But a lot of businesses don’t because their core AI strategy revolves around maybe predictive models or recommendation aspects, or maybe more indexing work. And so that’s why we wanted to bring a very simple way, almost like following an 80-20 rule, like What can symbol generate? Percent out of the box for you that gets you to 80 percent. On understanding conversations. And then lets you do the configuration. The customization, the personalization of that data to your business, to your use case, to your product for the rest of the 20% so that we meet you somewhere in the middle. And that’s really how we architected our API platform. And of course, businesses that need more control can get access to the models directly. So we always. With them strategically. So all the technology that we have built so far in the IP that is generated is around generating new and new use cases, new type of insights, new type of ways to think about how human brain comprehends conversation, how can we replicate a machine to do the same thing. So yeah, that’s a little bit about it.
Tara Shankar – 00:08:08:
Awesome. Well, Symbol.AI is focused on creating a machine learning platform that promotes secure, scalable, and explainable AI for human-to-human conversations, to exactly what you just mentioned. Why do you think these three aspects, security, scalability, and explainability, are crucial in today’s conversational AI landscape? And how does Symbol.AI ensure these aspects in their services or the offerings which you have?
Surbhi Rathore – 00:08:33:
Absolutely. I think us coming from a very large enterprise that worked in the Telco kind of created that roots to these three aspects like both the founders meet my co-founder, we both come from Amdocs. And so we have seen the level of security and scalability and explainability, which is needed. All these three core pillars. To really make a technology give value at such a high impact and scale. When we started Symbol, this was very core to us building the platform to an extent that after the first year of the company, we actually had a CISO on board, which is very rare for companies to make the first dollar investment into. But we wanted to make sure that we have a security officer, we have the right kind of policies and restrictions and access and authorizations in order to make sure that being a small company, big companies can still trust us and make sure that conversation data is safe and secure because conversations are a little unique in data aspect. I think we feel personally to conversation data, which might not be for other forms of data. There’s like an emotional connection to that. And so bringing very high security and privacy in the platform was a big part of that because of the nature of the data itself and the type of customers that we would want to work with. That’s one. Scalability aspect, of course, we would want our technology to create massive impact and you can only have massive impact when you can apply it across huge volumes of data. So scalability was always a part of it. And so with scalability also comes latency and the real-time aspect because the platform caters to a lot of real-time intelligence. So how can we detect and trigger insights and indicators and intents and what we call trackers in like less than 400 milliseconds in order to take an action in real-time? So that kind of goes hand in hand with the scalability aspect to it. And explainability is interesting because I think that’s the nature of the technology that we use to solve the problem. AI in itself is not explainable completely, whether it’s deep learning models, whether it is now large language models, even more. And predictability is crucial for enterprise adoption because they want to make sure that when they apply technology to solve a problem, it can predictably do X things with X, Y percentage of accuracy or precision. Knowing that is more important. Then, you know, like, oh, we are going to get 200% very quickly, but do you exactly know where you stand is very critical because then you set the right expectations for the customers, for the support team, you can create processes around your organization to support that kind of predictability. So that was important. And that’s why we’ve been very cautious of making sure that we understand and also communicate the type of data that we use for training. And how can we use that data in a very privacy-first approach? So is it redacted? There are no biases in it. We use data protection from the very beginning. And how do we make sure that what kind of combination of data is it very central to meetings? Is it all conversations? Is it distribution? Like all that kind of stuff.
Tara Shankar – 00:11:49:
Interesting. Well, I think that opens up quite a few questions from there, right? And this is all about GenoDB and language models this entire episode and discussions. So I’m going to ask three questions together. And we can always go back in case we have to backtrack the question. Can it help into how large language models play a role in your platform today? And how are these models actually helping in shaping customer experiences? Now, that adds two things to it, which I think you mentioned right now. One is how is Symbol.haven handling the challenge of bias that might be present in the data? And second, how if you can elaborate on the process of training and fine-tuning large language models for Symbol?
Surbhi Rathore – 00:12:27:
Yeah, I mean, we are a platform company, which means that we build the technology that we provide as APIs and scalable. And it’s very important for us to own the end-to-end tech stack because then we can control and customize it when we deploy it on-prem or as a cloud-agnostic tech stack for customers. So it was very important from a strategy of the business since the very beginning to own the tech we built. And with predictability in the center, we focused first on solving more narrower and deterministic tasks for conversations and then added a generative layer to it. So when I say deterministic or discriminative tasks like sentiments and entities and action items, segmentation and classification of data, all that kind of stuff. And then in 2021, we added our first generated model for summarization. And that opened up the doors for generating more unstructured data out of unstructured data, which is very interesting. And we saw a massive adoption of that in the year 2021, which also led us to our Series A and beyond. So post that, it was very helpful to work directly with customers and businesses that are integrating summarization at that point. That time it was called abstractive summarization. Now everyone just refers to it as a generated insight. That summarization and that model evolved into generating summaries for smaller parts of the conversation as well. So if you want to generate a summary around a specific intent or a timestamp of the conversation to kind of bookmark of customer feedback and things like that. So I think we launched and called it Bookmarks API in the year 2022. And so we kept evolving that to finally, I think last week when we announced Nebula, which is our large language model built for human conversations. And it is built with conversation, for conversation-centric use cases. Because there is a gap when you look at general large language model and a conversation-specific large language model on just the speak to value that you can get to audio, video conversations, chats, emails, multi-party conversations that really are talking for an objective. So we saw that gap. We had our own models first. We tested other models and thought that that is a space that we want to enable our customers to develop solutions faster. And so, yeah, we just announced it last week. And it’s really amazing to see the interest in just a week that we have got from. People and community and AI researchers that are looking to take that beyond and apply to use cases. So that’s a little bit about what we do with our language models. And Nebula today powers a lot of our APIs. Already. So we have a foundation model layer. Of course, there’s a foundation AI layer that we have, which is Nebula I mentioned. Then there is APIs, which are built on top of Nebula, some of them, and some of them use our existing task-specific models, which are more predictable in nature for discriminative aspects. And then we have more applied APIs also now that actually takes the combination of all of this to solve a specific business problem. And the one that we announced last week was call score, which enables you to actually programmatically score the call on certain parameters instead of just identifying an intent in the call. Now you can also score on that intent or that indicator. So yeah, we are trying to marry both the worlds to deliver an applied intelligence being an API-first company.
Tara Shankar – 00:16:01:
Interesting. And that’s in real-time. You can score while you’re talking.
Surbhi Rathore – 00:16:05:
Yeah, so today the scoring happens after the call, but the next version of the product will be able to give you indicators to that score in real-time. So today we have something called as trackers that lets you identify those indicators already in real-time.
Tara Shankar – 00:16:18:
We have a journey on the similar lines with our platform, so I think it does bring in the real-time analytics, sentiments to kind of be addressed or during the conversation itself, especially over the voice even more. Because later on in post-call or post-discussions, you can definitely look into the metrics, but it’s critical to understand what’s really going on during the time of the conversation. Glad to hear about that. Now, certainly a lot, and we all know that everything is dependent on the data, the clarity and the curation of the data. Now, that pretty much lets you build your models. Machine learning is all about that, right? So can you give us a little bit more insight into how AI systems like Symbol.AI is able to translate raw customer data into actionable insights for businesses or enterprises across different segments and sectors?
Surbhi Rathore – 00:17:10:
We have the same API first approach to do that. I hinted a little bit upon it in the beginning that we follow this 80-20 rule with our APIs where we extract more capability level insights and now applied insights that we just launched and that enables you to get structured insights out of the box. And then you have the platform to be able to customize, fine-tune, apply and set up a business-specific RLHF with your own data. So that makes sure that the ongoing adaptation of the AI is specific to the use of the intelligence in your business setting along with your data. So that way you can start very fast, but it gives you a much better starting point to start personalizing the outcomes because that’s where we feel is the most gap. I believe every business should have AI that is personalized to themselves. Like there is no general AI basically for a business, at least today. How we get there is a journey that we will take. And like with the advancement of the AI, like it could be as simple as just configuring the name of my business and boom, like everything automatically works. But until we get there, there’s a little bit of like the how to that we all need to do in order to be there. So one approach is that you take an open source model and just like start training it with your own data, start there, build a whole infrastructure yourself and own it. And then continue to evolve and invest into fine-tuning adaptation for your own specific data. The other approach that businesses take is going to reuse a full product out of the box, which they feel is more central to the use cases, like a point solution, because they know that it has some domain expertise, a specific area, and then I can just go in and use that out of the box as a business. I don’t need that much of a training, so it’s a part of their own strategy. And then there’s something in the middle, which is where we fit, which is like, well, we can give you acceleration immediately out of the box and give you the control to go and customize and fine-tune it and personalize it to your data without having to build completely from the scratch. So it just saves tons of upfront investment, but also not have the rigidity of point solutions. And I think there is a customer segment for each of these, right? Depending upon the problem they’re trying to score, the stage of the company, the size of the company and how much crucial is additional data for them.
Tara Shankar – 00:19:34:
And you narrated it so well. I think given there’s so many different layers to it where you can talk about, let’s say, generative models with chat, GPD, GPD-4s, Llamas, and whatnot, which is like more in the scale. And then you have the knowledge, which is basically about the industries and the models trained on that specific knowledge. And then you have your proprietary data on which you’re building your rare study models. I think there are different layers to it. And then eventually the fact that different large language models for different use cases actually tremendously help with the accuracy and hallucinations further too. So that’s a great one. Now with that comes a little bit more of the aspects of privacy. And I think that’s what we started discussing initially. So with privacy becoming an increasingly critical concern, especially with the generative AI aspects of things and how it’s going. How does Symbol.Ai balance the need for robust customer insights with the obligation to respect customer privacy? Can you share some of the safeguards you have in place to ensure data protection and privacy today? It could well be your viewpoint. Could be your purely your vision and thought leadership too. Would be great to hear your thoughts on that.
Surbhi Rathore – 00:20:44:
So we support, just on a very base level, we support enterprise deployments that are private to their own infrastructure settings. So that means that you can take simple models and like just deploy it on your own cloud. So that the data never leaves your environment and stays in the network references. For folks that don’t want to do that, we can, you know, with VPC tunneling, we can provide a more secure network connection to their own clouds. That’s another thing. We have the flexibility to deploy it in the region of choice. This is just basic housekeeping, I think, which everyone should anyway. But I think the most important part is the use of data in itself. That’s where I feel like the most privacy aspects come there because there’s always like pros and cons, like, well, if you don’t give data, how are we gonna train the models and make it better? And well, guess what? Like, I can’t give you my data because I’m running a FinServe organization. And of course my data is not gonna leave my ecosystem. So it is a challenge for a lot of businesses to solve. So how we solved, it was created a pre-built aspect of the models that again, you know, go back to kind of like the 80-20 rule of applicability. And that way you can get something out of the box with the general form of data that we have gathered through. We’ve had free product for a while and through the use of the product and access to the data, it has helped us kind of like figure out the nuances of it. Also, I think we’ve been a little smart about how we train the models. We don’t have like huge amounts of data training that’s going on. We focus a lot on quality over quantity. So we wanna make sure that whatever data we use is very high quality and really is a true representation of the outcomes that we wanna drive in the behavior of the model. And it’s not just any data. So we are very careful about it. We’ve had a data team since the very beginning of the company. And then when enterprise actually deploy into their ecosystem, the further auto-tuning of the models itself happen with their data. And that stays in their surrounding. So that auto-tuning doesn’t get transformed into other businesses auto-tuning because it is very different from business to business. How my agents interact with summary and correct it in CRM and How the summary needs to evolve is very different for an insurance business. To a healthcare company. It’s really not the same, right? So there is a little bit of personalization that happens on the top. So we let businesses take care of that into their environments. And that way there’s very high privacy standards that we can reach to.
Tara Shankar – 00:23:16:
Just brilliant. I think it’s the way we are approaching these different segments of customers, training the data based on the requirements of that particular sector. Segmentation is super critical. Well, on our side, we kind of do the same. We have a lot of conversations that’s happening on the back end. It’s eventually training the data on that regularly and automized certainly. Just helps with building a better intent recognition. We last year launched something called as Dynamic NLP, which was based on zero-shot learning. And the whole idea was to not spend time on training the intents and the utterances. So that saves a lot of time because now you’re going from months all the way. It’s a pre-trained model, continuously learning. It goes from months to literally days to kind of get your BOT ready for the sort of intents you may be looking at to even talk to the BOT about. So great, great, great point there. You have such rich experiences with working with enterprises and customers. Would you like to share a situation or an example where data-driven customer insights significantly impacted a company strategy or decision-making process? And how did it shape the company’s approach towards their customers?
Surbhi Rathore – 00:24:28:
Yeah, absolutely. I think we started our journey of building for real-time AI. That was like our first SDK that we launched back in. 2019 with pilots. And since then, we’ve been working with customers that are using real-time AI to influence the NPS on the call, to increase engagement on a sales call, to be able to create margin use cases when someone like an agent needs help immediately, and to sometimes also create very highly empathetic communications, which is a pretty general use case for most of the systems that are working in customer experiences. So we’ve seen rapid and drastic changes in all these different key metrics across these use cases, whether the more engagement on a sales call, of course, leads to increasing deal closure rates and, of course, sometimes even reducing sales cycle. Yeah, so both across engagement of the sales calls that influence the sales cycles by figuring out whether the question answers are being indicated in real-time and is the rep able to answer the question? And if not, they have to come back to it either post-call. So no questions are left unanswered and there is high engagement on the call. It really elevates the experience of a sales conversation. NPS use case is also very interesting because we’ve seen a lot of these different deterministic and then with webinar platforms, it’s very interesting because the statistics of the engagement are very like open-ended today for webinars and events. And there is so much that can be done with the parts of the webinar or the talk track that actually led to massive engagement and how you can stitch different modalities together. So the chat conversations, the emojis that can link to the parts of the how people are talking in real-time and influence that. So it really creates a highly engaging webinar that leads to more leads basically, which is what marketers are looking for. Yeah, I think those are some aspects. There’s another very interesting one, which is more about cost saving. And that relates to detecting answering machine, human in real time, doing intelligent call routing after that, that can really save the cost of calls that go into like voicemails unnecessarily. And if it does go into voicemail, identify and leave a message from the right points that you don’t get like half-cut messages in your voicemail that you see all the time. So there’s a bit of that, that work, which is very valuable, I think, in outbound calls that BPO’s and call centers are doing. Yeah. So I would say those are where we have seen like tremendous change in behavior before after with the application of real-time AI, but they’re all unique. Even NPS is so uniquely built in a FinTech company versus like an insurance company, it’s not the same. And so that’s why programmability sits at the core of Symbol, because that’s what we believe that every business and every company is unique in itself and the way they handle these metrics are unique to themselves. And so they should have the control to be able to flexibly experience.
Tara Shankar – 00:27:59:
Interesting. Are there any specific industry segments that you believe stand to gain significantly more from these personalized interactions and maybe even with generative AI? Is there a set of specific segmentation you think will benefit in the long term compared to the short-termcs gains?
Surbhi Rathore – 00:28:17:
I’m very excited about healthcare personally, because I’ve lived in different countries and US healthcare system is not the most efficient. There is just so much that can be done there. So personally, I’m very excited about it. I mean, it has advanced, but it’s not efficient. So there’s a difference in like bringing efficiency to every business process, right? I think that that’s something that I’m very personally excited about. And I think sales is an interesting spectrum specifically in insurance and automobiles and like all of that. We are gonna see new ways of doing these things given the kind of world we live in. But I would say if I have to pick one, it’s really healthcare that I’m very excited about.
Tara Shankar – 00:28:58:
Brilliant. Well, many companies are still trying to, you know, just beginning to scratch the surface when it comes to leveraging generative AI. And you know how it is, right? You have been there for the longest time. I’ve been seeing this for the longest time. And I think the adoption has never been like skyrocketing. Like a lot of the companies which made an impact in the innovative fast were the one to adopt machine learning. But as I said, like, you know, this lot of organizations still kind of just beginning or scratching the surface when it comes to leveraging AI to enhance customer and user experiences. What are the common misconceptions businesses have today about implementing AI-driven strategies? Like, have everybody figured out the recipe of success or sort of still thinking, oh, this is probably the way to go? I was speaking to Jim Stern in one of the other episodes, and I think he was like, you have to unlearn everything and then think about your strategies. What is SME gonna be? And then eventually start thinking about and a process to kind of land one after the other to get there. We’d love to hear your thoughts. This is pretty common to see those misconceptions in businesses and they are still thinking about the AI-driven strategies. And the right direction or not? And how do you really go about telling them what to do?
Surbhi Rathore – 00:30:08:
Yeah, I feel like we’re all learning together as a community, and it’s really important to embrace that we will make mistakes. On this new journey, right? So we have to be open to failures and we have to be open to experimentation and say, let’s do it. Like doing something and failing at it is better than not doing it at all. So one is we have to get out of the whole fear factor and find alternatives and options for your business that would work in order to experiment, test, and get things out, even if it’s a POC, but at least start your journey first and be open to failing. Second, I think as businesses learn and evolve out of what JNI means for them as a business, that is very critical. So just like I will adopt GPP in all my workflows, it’s not the answer. You have to figure out what AI means for you. So you almost need to set up two teams in any company. One team that is the experimental team that is going to take the open source models, non-open source models, that approach, this approach. I’m gonna test multiple pooling and figure out technically how we are going to build it. And then on the other side, you have to build a little small, a cross-functional SME, almost a team, which is going to touch as an outsider, all the functions and see, okay, where is the biggest pain and how can we drive the maximum predictable implementation of generative AI? And maybe your answer is going to be summarization and that’s totally fine. Like I’m gonna implement automated summaries for every call and that is going to create a massive increase in visibility and the time efficiency across the organization. And then I will implement maybe automated a search or a question answering for my support teams. And then I will provide a sales agent and automated follow-up email. And so you can also go like one by one and pick up the use cases where you have the maximum impact that you see depending upon like, what are you driving towards? Are you driving towards retention, churn, customer experience as like your P zero as a business or are you more, I wanna accelerate growth, new logos, like that is my focus? Or maybe you’re changing your product strategy and you need to really focus on product roadmap. And in that case, you need to derive product intelligence from every customer interaction which is happening. And so you need to know that which customer is talking about what product featured and how do they think of existing product in terms of upsell, in terms of gaps and feedback and use cases. So I think it really depends upon tying GenAI initiatives to the core KPI in the business that is priority for you and then breaking it down into like an SME or almost like a product person and then an implementation person.
Tara Shankar – 00:32:56:
So nicely explained. I have to commend you for that. Very nicely and thoughtfully creating those two different buckets and how we need to think broadly. I think that’s how we can strategize better too. You need to have that SME and then eventually the strategy to kind of go ahead in the direction you need to, to make the changes and adopt. Now AI and generative AI specifically and custom experiences as rapidly evolving, what trends or patterns do you currently see and that excite you and also concerns you? I mean, I can say, you know, hallucination is always a concerning thing for me. And that’s why we are building these, you know, smaller models, trying to definitely reduce hallucination, better accuracy, but would love to know your thoughts or be trends and patterns you currently see that concerns you.
Surbhi Rathore – 00:33:42:
Yeah, I second that too, TJ. I feel like hallucinations and less predictability of outcome is a very big showstopper for mass adoption. So we have to figure out a way in order to solve that problem, whether it is implementing more smaller models or more specific models or more domain-specialized models, whatever that may be, and accelerating the speed to do that. That could be one approach for sure. I think the other approach is just the privacy aspect of it completely, which we really obsess about privacy at every stage. And I feel like businesses also need to just educate their employees as well. In addition to building new products and solutions and adopting them, I think employees also need to know what data should be shared and where it should be shared and how to use that. So there’s just a more higher education on privacy that needs to be done. And it’s not just about sharing your data with external tooling. It’s also about. Understanding. That how are we fact-checking the answers? That we are getting in context to our business and based on the accesses that we have. So… An engineering team has very different access to the exact team. And how do we make sure when we give search experiences and knowledge management experiences? We take into account the privacy of data and information. Also. Within the business itself while fact checking the latest source of truth, because even source of truths keep changing with the business. I think that this adaptability aspect to privacy is very crucial. I’m very excited about the work that the whole research community is doing on both of those things. It’s an exciting time to be a part of the ecosystem.
Tara Shankar – 00:35:31:
Indeed. I forgot the name of the company. I was attending an event, and I think they actually literally created something called a truth checker. I don’t know if you probably know the name of the org, but this is completely in the custom experience and context center side. And that was eye-opening because you know which entity we are. You will get an answer no matter what. Now, whether it’s really good enough in terms of the accuracy of the answer, I think you need to add some sort of validation. To your point, totally agreed in terms of how you want to do that check, whether internally or to actually have a tool which shows, hey, look, what I did for you was able to validate the truth. And I’m actually going to give you the data or the outcome or the output, which eventually is going to be more accurate for the question you asked. So any thoughts on that in terms of how you see that further? I mean, great point is this. So I just thought I’ll add this because it definitely matters a lot to the organization’s day.
Surbhi Rathore – 00:36:28:
Absolutely. I agree to that. I think there are two aspects of this. One is I am adopting Gen .ai for my internal operations and then two is I’m building a product. That is GenAI password. And I think there’s a version of privacy which is very central to both of those aspects. So, and you can learn about that by doc-fooding that internally within your own company. So if you are building a product doc-food internally, learn about the user behavior because the user behavior is also super new. And how do you add the whole privacy element to the user behavior and user experience is also equally critical.
Tara Shankar – 00:37:05:
Well, as we come towards a closure of this wonderful, wonderful discussion, I want to just hear one thing from you, especially for the audiences, because we have a lot of enterprises and people from the customer experiences side and generative AI side will be listening to this podcast. What is the best piece of advice you would like to give to organizations seeking to leverage AI, especially generative AI, to elevate their customer experiences?
Surbhi Rathore – 00:37:31:
Yeah, well, I would say start your journey sooner. Don’t worry about failures. I think users are more receptible to the flaws in the AI systems than they were three, four years back. So you have a great audience that is looking to be receptive to new use cases, new ways of doing things, new ways of using Gen AI. And data is critical. So think about data as a product and not as a derivative of your business. And try to find out high-quality specialized data sets, which is going to help your business, not just build specialized AI systems, but also test them, because testing is as critical as building.
Tara Shankar – 00:38:14:
Brilliant. Think of data as a product. Beautiful thought. Well, we’ll take a pause on that thought. And the fact is that it’s been an amazing discussion. Thank you so much for your time, Surbhi. And I can tell you that there’s so much of learning I had while talking to you, given we work in the same space, such clarity and thought process, which are kind of drilling into Symbol.Ai. So wishing you all the success on that. We’ll talk more for sure. You know, there’s so much, as I said, to learn from you. So we’ll keep bringing you around. And other few episodes as we kind of continue this journey on the Northern Otherbot. But on that note, I would like to extend my thanks and hopefully the audiences will get that insights they’re looking for to take the journey in the next steps into building their AI-centric applications, keeping, you know, ethical AI in mind, personalization, and the human-to-human conversations. Thank you so much, Surbhi.
Surbhi Rathore – 00:39:06:
Thanks to you for having me. It was fun, Jai.
Tara Shankar – 00:39:09:
How impactful was that episode? Not Another Bot, the generative AI show, is brought to you by Yellow.Ai. To find out more about Yellow.Ai and how you can transform your business with AI powered automation visit Yellow.Ai. And then make sure to search for The Generative AI Show in Apple Podcasts, Spotify and Google Podcasts or anywhere else podcasts are found. Make sure to click subscribe so you don’t miss any future episodes. On behalf of the team here at Yellow.Ai. Thank you for listening.