Transcript
Tara Shankar – 00:00:03:
Generative AI takes the center stage, but is your enterprise still watching from the sidelines? Come on in, let’s fix that. This is Not Another Bot: The Generative AI Show. Where we unpack and help you understand the rapidly evolving space of conversational experiences and the technology behind it all. Here is your host, TJ. Hello and welcome to Not Another Bot: The Generative AI Show. I’m your host, TJ. Joining me today is Jim Sterne, a renowned speaker and consultant. Jim’s expertise is sought by Fortune 500 companies and ambitious Internet entrepreneurs alike. With an impressive career spanning over 45 years, his focus has primarily been on understanding and harnessing the power of digital media to foster robust customer relationships. Jim has authored a dozen books covering a wide array of subjects from advertising, customer service, and web analytics to AI. His career trajectory is a testament to his adaptability and forward-thinking vision. From the days when the term personal computer was an oxymoron to selling business computers to first-time owners, and then creating platform for digital analytics, Jim has always been ahead of the curve. Now his focus is on the application of artificial intelligence and machine learning and marketing. I’m absolutely excited to be here, Jim, and welcome to the show.
Jim Sterne – 00:01:21:
Thank you very much. It’s an honor to be here, and I am slightly intimidated by what you might be asking me today.
Tara Shankar – 00:01:29:
Will make it very exciting for the audience for sure. There’s so much to learn from you and your insights and experiences. I think it’s gonna be one of our amazing episodes for sure. All right, so Jim, my first question, you have had a truly impressive career, right? Spanning to a long time, but eventually primarily focusing and measuring the value of digital media to enhance customer relationships. Can you share with us how your journey began and what motivated you to dive into this relatively new domain at that time when you’d gotten?
Jim Sterne – 00:01:58:
It’s all about me, huh? Okay, fine. I tripped over the Internet on April 22, 1993 when Mosaic was first released and thought, oh, this changes everything. So I ran around asking people what their online marketing strategy was and everybody said, we have no idea. We’re inventing this as we go along. What do you think? And I told them what I thought and they thought that was interesting. And so I became a consultant. My method of getting clients was public speaking. I would speak at online Internet world conferences all over the world and tell people how ugly and poorly designed their websites were. And they would then come up to me and say, can you come back to my company and explain that to my boss? Sure, that’s what I do for a living. And then in the late 90’s, I discovered web analytics. And it wasn’t just my opinion that your website is ugly. We could actually measure it. And then we could test whether or not my ideas about improving your Website actually were valid or not. And that became, that was a book called Webometrics. It was a conference that is now called Marketing Analytics Summit. That’s been going on for 21 years. The audience from that conference created the Digital Analytics Association. And then along came Machine Learning. So 5 years ago, my book on machine learning and marketing came out. And now we have Generative AI. So I’m just fascinated by whatever is over the horizon. What comes next and how does an organization make use of it?
Tara Shankar – 00:03:32:
Oh, brilliant. And then just to tap onto the same kind of question and following up to what you just said, given you have seen personal computing booming, online marketing booming, and then certainly the whole surge in artificial intelligence, and especially applications and in marketing, how difficult that transition, how did you navigate through these different transitions and what inspired you to consistently stay ahead of the curve? Because we keep listening to you and the thoughts you generally share is already ahead of the time. So I mean, how do you really kind of transition from personal computing all the way to artificial intelligence? Would love to hear that for sure.
Jim Sterne – 00:04:10:
It’s a matter of where to focus. So very early on, I identified things I would not pay attention to. To start with online security, data security. Oh, that’s just a rabbit hole that would be too fascinating and requires unique expertise. And my background is sales and marketing. So I’m gonna stick with what I know and focus on marketing. It’s also a matter of not being a practitioner. So I pay attention to what’s going on rather than actually doing the work. And that means instead of being an expert in Google Analytics, I understand what it’s for and how difficult it is and how it compares to Adobe Analytics and PwC Pro and other tools that are out there in order to advise companies on strategy for using them. And strategy is this combination of people process, technology, politics, and prioritization. So when companies right now are saying, well, wait, what does this mean? I just hark back to, well, when we were selling personal computers, it means that you can have a computer in your home. When we have business computers, it means that you can get rid of all those file cabinets. When we have the Internet, it means that you can access whatever people are publishing all over the world. And then Web 2.0, it can be interactive. What does that mean? Oh, online commerce. Oh, it means you can personalize the experience for me. Then comes machine learning. Oh, well, that means that we can take all of this data that we have and actually make sense of it. We can have the machine find correlations and anomalies and point out things we didn’t know. Then we have Generative AI. Okay, what does that mean? Well, a whole different kind of computing.
Tara Shankar – 00:05:56:
Awesome. So in light of your extensive experience, how do you perceive the influence of Generative AI evolution on the analytics landscape with large corporations or even with startups and beyond? It would be good to hear what sort of key differences and improvements have you observed data analysis with Generative AI compared to traditional computing?
Jim Sterne – 00:06:14:
Well, let’s talk about traditional computing for a second. The historical perspective is programming. We’re at the prompt level. We tell it what we want. We write a program. It executes. Then we create applications, like a spreadsheet, where I can put in a variable and a relationship to another variable and change one of those and it recalculates for me. Ok, so that’s a level higher. Then we move to predictive analytics, where I’m taking a lot of data and crafting a model that says, well, this is what happened in the past and therefore this is what is likely to happen in the future. And we can determine, oh, look, we’re better than flipping a coin, so that’s useful. Then machine learning that says, oh, the machine is going to determine things and structure and rubrics about the data from the data and tell us things we didn’t know.
In each of these steps, the amount of education required was significant, but the adoption was slow enough that you could say, okay, tell me who’s a leader in predictive analytics who can walk me through this? Tell me who’s a leader in Website design that can help me understand what makes a good web experience? The biggest difference we see with Generative AI is that it has happened so suddenly and been adopted so broadly that everybody is equally uninformed about it. So when you ask for, I need an expert about Generative AI, well, let’s see, let’s go on LinkedIn and get hit with a thousand offers of the ultimate prompt engineering cheat sheet. Do I need an expert in creating my own large language model, integrating a large language model, training my own proprietary large language model? Do I need an expert in prompt engineering? Do I need an expert in how to use these things for medicine or education or marketing? We have a new horizontal piece of technology. It is a new operating system or a new user interface is actually a better way to describe it. This new User Interface is applicable across the board. Every application will have a large language model front end to it. Every image generator or video application will have a diffusion model associated with it so that you can interact with it conversationally. That’s just the way it’s going to be from now on. We went from the command line to drag and drop to conversational. So the impact is enormous across the whole organization when it comes to analytics. The fun part is that it can do some of the boring bits for you.

We went from the command line to drag and drop to conversational, so the impact is enormous across the whole organization.

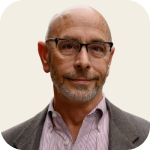
Jim Sterne
President at Target Marketing of Santa Barbara

Now, with all Generative AI, the caveat is always it hallucinates. So be careful. Well, if I have a standard data set, I’m using Google Analytics, it is structured data, and I ask the ChatGPT-4 code interpreter to interpret my data for me, it will happily and confidently, and it won’t necessarily be correct. So we have to remember, we have to understand it’s not a database, it’s not a search engine, it’s not an analysis, an analytics system like SPSS or something. It is just generating the next word based on what it sees. So today, the value is looking at the data as an individual and asking my specific questions of the data in an analytics tool, and then turning to Generative AI and say, help me create a narrative that describes this, help me create PowerPoint slides, take this data and make these seven different kinds of charts. But don’t ask it to do formal analysis. It will give you results, but you have to be a very high level subject matter expert to determine whether it’s hallucinating or not.
Tara Shankar – 00:10:08:
Interesting. And just to add to that, when you talk to organizations or you generally discuss the implementation of Generative AI, is hallucination a common discussion point, or is it mostly around security, privacy, or is it a combination of all? What do you generally get to hear from the organizations, especially large corporations when you’re talking to them?
Jim Sterne – 00:10:27:
Hallucinations are central to the conversation because people think a computer gives you an answer. People believe that it’s a database, it’s a search engine, and it is absolutely not. It’s a different kind of thing. And so you have to ask it different kinds of questions. We are learning slowly what kind of questions are appropriate for a large language model. What is the answer is the wrong question. Here’s an outline of what I’m thinking about. What else should I include? What are alternative ways to describe this thing? If you were an expert subject matter expert in this field, and I made this statement, how would you argue against me? Those are the kinds of things that aid creativity, aid brainstorming. This is a revolution in creativity rather than a revolution in data or analysis.
Tara Shankar – 00:11:22:
Well, as organizations are undertaking the journey from traditional computing all the way to Generative AI, and thankfully I was lucky enough to kind of work on some of the Generative AI-centric products, use GANs, VAs in the past, which was awesome. But then it was all around creating music or art, but now with the models like ChatGPT, which is completely changing the game in customer experiences and the way you kind of get answers to questions in a more human-like fashion, which is awesome, right? Now, what I wish to learn is what challenges do you often observe when these organizations are taking the journey from traditional computing to Generative AI, and what innovative solutions have been employed to address them? Like if you have any sort of case study or any sort of thought that comes to your mind, up of mind you want to share, that would be awesome to learn what the journey looks like today, because a lot of the organizations are still trying to evaluate and figure this out. And I think if we can get that sort of insight, it will be awesome.
Jim Sterne – 00:12:20:
There are multiple paths, of course. The first one, historically, is hire a whole bunch of data scientists and rent a whole bunch of compute time and invent stuff. And half the time, you’ll hit a brick wall, and that’s called learning. And that’s fine. For the breadth of what Generative AI can do, that is not an economical way to go. Instead, the first step is this concept of letting 1,000 flowers bloom. Let’s teach everybody in the company about Generative AI and ask people to play with it. Now, we need policies in place, of course. If we’re going to use ChatGPT, then we need to make sure that no proprietary data is uploaded. That’s fine. We get it. But we can also use proprietary. We can license or use open source behind a firewall and let people play with it all they like, understanding that there is a compute cost. So all they like is in air quotes. But getting people just to understand what it is. So imagine that a spreadsheet had just been invented. What can I use a spreadsheet for? Well, anything. Use your imagination. What’s it good at? Numbers, almost anything. And that’s hard to explain to everybody in the organization. They just have to play with it. The next level is from the top down, what are our corporate priorities? If I can use this horizontally for the front end of anything, what’s the most important task to accomplish or the most important goal or start with a vision and then give me which of the five business problems we’re trying to solve are the most critical today in priority order.
The five business goals are increased revenue, lower cost, increased customer satisfaction, improved capabilities, and lower risk. And if you’re working on something that’s not associated with one of those five, you better find another job or at least another task. So which of those, and this is a corporate culture question. Some companies say, oh, well, we absolutely need to raise revenue. It’s like, well, fine, sell $5 bills for $2 and revenue will go through the ceiling. Are you sure that’s what you want? But some people say, oh, we have to cut costs. Some people will say, customers are number one concern. If customer satisfaction goes up, revenue will go up. Everything will, great, great. Whatever the corporate strategy is, that’s what we’re gonna prioritize for, let’s put some money behind this. Let’s get alignment in the senior management to agree that that’s where we should be putting it. So if somebody in department A has to relinquish some resources so the department B can successfully execute strategy, it could happen rather than them fighting each other. I’m just quoting the five dysfunctions of a team by Patrick Lencioni here. So from bottom up, learn how to use it. Top down, prioritize where to invest.
Now, the next step is how to invest. Hire a bunch of data scientists. It could be partner with universities. It could be wait until this afternoon when another venture capital backed startup produces the thing that you were trying to create. I mean, the breakneck speed is just bizarre. So I think what I’ve seen people do successfully is prioritize data curation because the moat is how good is your data. I’m going to use one of a dozen large language models that are out there as my foundational model, and then I’m going to fine tune it and train it with my proprietary data in a secure space. How good is my data? Are my subject matter experts properly trained on identifying, curating, tagging, and ranking the value of the data? And do I have an absolute robust process for managing the addition of new data to that model for the given priority? Across the board, the whole company, we need a data provision policy and process that is bulletproof and nobody has that yet. So there’s priority number one. Then it’s a matter of, as happens in every organization, which proposal gets put forward first.
So if we say, okay, raising revenue is our most important strategy. Okay, good. Do we want to invest then in advertising at the beginning of the process or customer service and sales in the middle of the process or conversion optimization at the end of the process? And depending on who comes forward with what concept, oh, if we implement Generative AI on our website and every individual has an individual experience, we’ll make more money. And then the next person says. We really need to double down on account-based marketing so that if three different people from the same company come to our Website and interact, that our large language model is aware that they’re all from the same company and maybe puts them together in a conversation. Maybe not. There’s where we need the human in the loop. Or it might be the web designer saying, if we apply this to the shopping cart, we can double the conversion rate. Now somebody has to decide, okay, which of those three is going to get funded first because you cannot do everything.
Tara Shankar – 00:17:50:
Exactly. One thing, Jim, here is as this journey continues, and one of the, well, we as a company, our platform company helps with Automation, right? And we could see the whole impact we could generate with Generative AI. We literally launched our product. We were working on it for some time anyways, and we were able to launch it, like, record three to four weeks because we had all the things that was required, and it’s certainly helping us round a better narrative which is super critical with customers as we are in the CX space, right? My question would be as we continue the journey from there, could you articulate how large language models such as GPT-4 or the others in the market today are reshaping custom experiences in automation? And how do you see the future of automation changing? Would love to get your, you know, more of a visionary perspective on how these models have transformed or can transform the automation space and custom experiences.
Jim Sterne – 00:18:42:
Automation happens by channel. So am I going to automate the personalized experience on the website? Am I gonna automate the call center interaction or email marketing or billing? We have to pick and choose. What things can be automated? The decision space has grown dramatically. Anything that we would typically give to an intern can now be managed and handled. As long, again, the caveats are in place. There is hallucination. We need a human in the loop. All of these things apply. But as we train our own large language models with how we work internally, we can start asking it, what is the next thing we can automate? And it will do process mining and say, well, generally, the product management people hand off to the marketing people at this point in time with these assets so the marketing people can create the promotional and branding products they need. This happens the same way every time and can be automated with this sort of API and that sort of file transfer program. Oh, that’s great. Can you please create the API for me? And now we’ve discovered that English is the next programming language.
Tara Shankar – 00:19:57:
Correct. which is amazing. My wife is an English literature person and I keep talking about her about this. She’s always been away from technology, but whenever she did, she’s like, oh, it’s kind of boring, you know, I’m in a better world. But now that I keep talking to her about her, that English is the new programming language and that you need to know, and that’s how it’s changing the entire game, you know, in the industry. So it’s amazing to see how things have changed over a period of time.
Jim Sterne – 00:20:24:
I want to double down on that by when I’ve talked to my wife about it, my wife who is a Superior Court Judge, and she said, okay, if I understand what a large language model is, you’re telling me that it will give me the best answer. but not necessarily the right answer. And I say, yes, that’s exactly how it’s designed to work. And she said, oh, so it’s just like people. And in her courtroom every day, she hears people trying to give the best answer rather than the facts. So that’s part one. The second thing I wanted to cover regarding your wife is using this in school and specifically English literature. My favorite perspective on it is a high school teacher in the Northeast way back at the beginning, like within two weeks of ChatGPT being released said that she was excited. Instead of having her students write essays and bring them into class and have everybody critique them, which is a horrible blow to a teenager’s ego, she had the students take the assignment and ask GPT to write the essay, bring those into the classroom and critique those. So they’re learning good writing skills. They’re learning critical thinking without it being an ego blow. That’s the sort of thing that this is really good at, right? It’s great at, write me an essay about something. Now let’s critique it for fact checking and structure and, oh, repetition and just boring writing. Oh my God.
Tara Shankar – 00:21:53:
So true. Like my wife actually launched her book recently. She’s an author. She writes nonfiction. This is the second book. And then I was just telling her, hey, if you have to summarize, how would you? And then I just showed her ChatGPT and like, Okay, look, I’m just going to take the things you wrote about this book. And here is my sort of, and I said, I’m going to put a sort of a recommendation for your book and a review. And let me ask ChatGPT what that looks like. Well, when it came out and she’s like, oh, there’s so many errors into it. It’s like too flowery. It’s not this and that. I said, Okay, hang on. I used a different prompt to say, hey, make it more precise, concise, write it like as if you are a real human, read the book already. And it just wrote a very strong five lines. And she’s like, hang on, what did I just see? And that’s how I’m making her believe. how technology can really make the change. She’s like, hang on, this is really cool. And she high level of critic in terms of you are not speaking the right English or not writing it properly. So she would always tell me or the kids, it’s always a thing, right? No matter what. So it was great to see, kind of getting some sort of a nod head from her.
Jim Sterne – 00:23:01:
And we’re going to find that happening absolutely everywhere. Everybody that I’ve explained to this to previously, because now the knowledge has spread widely, said, wow, that sounds great, but it wouldn’t be at all useful in my industry because there’s too many things to know. Well, yes, it is useful because people say, “Oh, I’m worried that this is going to take over my job.” It won’t. The most astute thing I heard was that a job is a bundle of tasks and AI can help with specific tasks, but they cannot do the job.

People say, “Oh, I’m worried that this is going to take over my job.” It won’t. The most astute thing I heard was that a job is a bundle of tasks, and AI can help with specific tasks, but it cannot do the job.

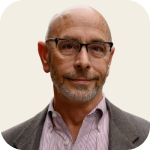
Jim Sterne
President at Target Marketing of Santa Barbara

Tara Shankar – 00:23:34:
Exactly. And I think as we got know, they did predict this year, it’s going to be even more jobs created for people, I think 2.3 million jobs by this year, which is actually true because a lot of jobs out there, people are looking for prominent engineers to be on. I’m just taking an example, but that’s truly the thing. So thanks for calling it out, but that’s the reality. I think it’s augmenting intelligence, but rather than just taking away anybody’s creativity or the job, I think it’s just helping the whole aspect of how to augment human intelligence into the ecosystem or the applications itself.
Jim Sterne – 00:24:08:
I remember being concerned when I was writing, as you said, I’ve written a dozen books. When I was writing and using Microsoft Word Thesaurus, am I really writing or am I just getting the machine to do it for me? And my wife said, well, you’re choosing which words it suggests. It’s still you finding the best word to fit your idea. So it’s a tool, just like spell check is a tool.
Tara Shankar – 00:24:36:
It is indeed. That’s where I could see the value. And seeing and doing things for the longest time with the AI and Generative AI now, I see the impact like 10-folds from what at least I did with GANs and beyond. The applications have become more easier to adopt and understand. Well, on that note, the next question is, as many businesses harness AI more effectively in the CX and UX domains today, could you share any of the strategic recommendations you may have, Jim, for these organizations, that how they can evaluate the business, enhance its readiness to adopt AI into the custom experience strategy? Because a lot of our viewers for this podcast will be in the CX domain, whether it’s contact center, customer support, or even more. But I think Generative AI is reshaping experiences predominantly in the customer experience space. So we’d love to know what sort of recommendations you may have for them.
Jim Sterne – 00:25:27:
The first concern is expectation levels are going through the roof. We all dealt with pre-November 2022 Chatbots. Before ChatGPT, Chatbots were horrendous. Hi, I’m your Chat bot. I can answer your questions. Yes, I have a question about this. I don’t understand your question. And we all just said, Okay, forget that. Voice response for English Press 1, for Sales Press 2, oh, horrendous. But now I can call up and say, I have a question about my airline ticket. It looks like I know that I applied for the upgrade using my mileage points, but I don’t see it on the ticket. Can you tell me what’s going on? That’s a very random, complex set of words that a human understands completely.
Now Generative AI can interpret the meaning in order to now use a rules-based system to find a proper answer, a curated answer, or hand me over to a human. And my expectation from this moment on is that every system, every website, every phone call can be managed with a computer because it can understand my intent. Saw a rather frightening example two days ago of a outbound sales call, supposedly from Tesla, where the machine said, Hi, so you came to our Website and I’m wondering, are you in the market for a car? And the human blathers on about, yeah, it’s time to be like, no, I’m a Tesla fan, but, you know, I’ve never really thought I could buy one. And it engaged the machine, engaged this guy in conversation. It was obvious to those of us who were paying attention that the four or five second pause between the human answer and then the machine answer was the clue. But the vocal inflection, the types of questions that were being asked, the ability to mimic back what the guy had said means that my expectation is one.
That’s what I expect from now on. I expect everybody customer service to be able to have a machine actually talk to me. And then I will lower my expectations because I will not be fooled. We are not really at the uncanny valley yet. It’s pretty obviously a robot, but it works and that’s fine. But it does mean that every application, internal, external website, email, everything has to have this Generative AI interpretive capability because suddenly the bar is just jumped way high.
Tara Shankar – 00:28:06:
So true. We, as a company, we have, we work on a multi-lens architecture. So we have specific elements for summarization beyond and like document cognition and whatnot. And voice is something we have been working on like pretty strongly. And I think we did see how and why building an in-house model was better because of the lag, which you have to deal with the accuracy issues, the hallucinations and smaller data set or smaller models helped. Now, just I’m not trying to go off track, but just your point of view, Jim, do you see there’s more scope? That’s what I’m hearing and I’m reading to myself, but want to definitely validate with you. Smaller language models. Is that what the future is going to be like given the large language models, like very difficult to kind of scale and go beyond? Do you have any take on that? And if you think enterprise will be more kind of eager to adopt, given they may have a easier adoption path, less hallucinations probably and better accuracy.
Jim Sterne – 00:29:03:
Application specific. So for the majority of us, we can start with a large language model, an open source one, we can use the one from Meta or the one from Microsoft or OpenAI. And then in a safe space, a walled garden, train it with our proprietary data and layer it with rules and guidelines and directives to say, never answer questions about this. If the question is that, always refer to a human. If the question is not about this specific domain, apologize, I’m just a large language model, but don’t just invent things. And then of course, there’s the technical approach of let’s create a adversarial model that says I’m looking for something in the output that is inappropriate or incorrect or out of scope. And I’m going to make sure that the results don’t reach the human being until this is corrected. And I think that’s going to be a standard approach. I believe that if I were any of these large language foundational model creators, I would make sure that there were a ecosystem of control capability around that large language model that enterprise can subscribe to.
On the other hand, we watched Bloomberg, for example, it start with the bloom large language model and then add their own millions of documents about financial advice and financial planning and then news and marketplace. What are the results? What’s the stock market doing this hour and creating an internal model that their financial advisors could use when talking to a specific client. On the other hand, Morningstar took a more generic large language model and they claim within just a couple of a few weeks and $3,000 trained it with their advisory information. Which one would you prefer to work with? If it is very specific, if Morningstar said, look, we’re just going to recommend mutual funds in the agricultural sector and that’s why we’re creating this large language model. It’s a much smaller model. It will be more accurate, but we always need the human in the loop.
Tara Shankar – 00:31:17:
Exactly. Completely agreed on that. Just to kind of add to that, we do have within our platform a specific protocol in bots, which helps with agent assist. So you’re talking to the bot now, the bot says, okay, our bots can actually do up to 90% automation, which is awesome. You can answer queries. But when the complex questions come like even beyond the levels we can touch, it just goes to the human. Now we’re trying to bring more Generative AI capabilities into their mailboxes or things so that they can type a better response, give it back to the bot or to the customer, understand the sentiments. I think that’s definitely quite, but I totally agree the human in the loop makes that difference. And I think that completes the narrative and the entire feedback loop as well. So that is so critical. On that note there, Jim, for any organization to stay competitive. Now there’s a lot of things going on. Everybody wants to get a piece of the pie, certainly want to get Generative AI incubated or do the adoption of it. And some of them already done it. Some of them are still thinking, but the ones who are doing it, probably they are seeing all that goodness, hallucinations, accuracy issues, or all the benefits of it. But how everybody should stay competitive and ensure that effective applications of these concepts are applied. How should companies navigate the swift progress in the AI field to keep themselves at the What are some recommendations you may have there?
Jim Sterne – 00:32:38:
Continuous learning, I’ve started a methodology when it came to the World Wide Web and how to do marketing and then how to do analytics, which is bringing everybody together in a workshop and everybody in a specific department. So the marketing department for a large enterprise brings their hundreds of managers together for a day. It opens with the most senior person they could find saying, this is important, pay close attention. I’m interested to hear what you come up with at the end of the day. And that person leaves the room. And then it’s time for, here is an hour of overview. This is assuming that there’s somebody in the room that knows nothing and assuming that there’s somebody in the room who knows everything. In this hour, we’re going to pack as much as we can to bring everybody up to speed.
Then we’re gonna bring in people from within the organization who are already doing things. How is artificial intelligence being used in this company today? Then break up into discussion groups. How might you use this capability in your group department organization project? And then feed all that back to the whole group so that everybody learns from everybody else’s brainstorming. Okay, now it’s time to prioritize. Let’s start with the senior level executives. What are our priorities? Whether it’s make more, spend less, customers happy, increased capability, lower risk. And given those priorities, how are we going to go about choosing? What attributes are we going to use to choose which of these ideas should be put on the start line? And then brainstorm, what will it take? What does the project plan look like? And oh, by the way, go ahead and ask ChatGPT to help you with the project plan.
By all means, this is brainstorming time. That’s step one. Get everybody on the same page. Get everybody going in the same direction. And then periodic come together. Google and Amazon do this really well. And a standard cadence bring people together to show off what they’ve created and learn from each other because the experts in the field, the McKenzie’s and the Accenture’s, they’re all learning this stuff as we go along as well. So you can teach each other in your special domain better than an outsider can. Another point I’d like to make is purpose fit. A large language model is entertaining, it’s fun.
It’s harking back to 1979 when I was selling Apple IIe’s. What’s it good for? Anything you wanna do, you can program it to do anything you want, was not useful until somebody came in and said, well, I wanna use it for accounting for my small business. Oh, we have these packages up here on the wall, Peachtree Software, General Ledger, Payroll, accounts receivable, accounts payable, and it’ll do that for you. Well, we’re now on the verge of finding these purpose fit point solutions. So my personal example, for the last five years, I have been looking for a system that will take everything that I’ve ever written and put it into a bots and allow me to ask myself questions. And how hard can that be? And for the last four years, I’ve been sent over to GitHub and Stack Overflow. I’m an English literature major. I’m not a programmer. This has been a dead stop for me. Until two weeks ago, somebody reached out to me on LinkedIn saying, hey, we’ve got a new product that we’re creating. It’s in Alpha. It is expert in a bots. And our intent is to take your content and turn it into you. You can ask yourself questions and you can sell access to people that they can ask questions of you, the expert, based on everything you’ve ever written. Would you be interested in working with us on a prototype? It’s like, oh my God, you have all of my attention. This is the product I want. Let me add it. And they said, well, how do we get access to your baseline training material? I said, well, Dropbox. I created this already waiting for somebody to come along here.
Three days later, they came back and said, okay, here’s your login. What do you think? I think it’s great, but it’s missing. oh, and now I’m a product manager. Okay. But it is fit for purpose. So it’s a large language model, a wonderful tool to play with. Yeah. Is it great for brainstorming for I’m planning a trip to New York City? What should I see? It’s great for that. But now specific for purpose. And as it gets better and better for a given domain, that’s where the competition will be. So the company that brings the model forward that says we have this base data and we’ve curated it very carefully. and we’re going to give you an instance of it that is secure, that you put in your own proprietary data, and we know that domain so well, we can support you and your people using this model for that purpose. whether that purpose is to run payroll or design rocket engines or figure out what path my janitor should go through the building to clean it the most efficiently. What’s the application? And we’re not there yet. This is what’s over the horizon.
Tara Shankar – 00:37:57:
Amazing. Well, on that thought and then specific industries and things we’re talking about, certainly I’m intrigued by your broad experience in digital media for the longest time. And you could see Generative AI and large language models are heavily disrupting the digital media landscape. Now, could you provide insights into how this disruption has influenced the value and measurement in digital media? What’s the sort of change you’re seeing? How people are changing their strategies from a marketing perspective, advertising? It’s crazy and the most impacted area at the moment for Generative AI. I would love to know your thoughts for sure.
Jim Sterne – 00:38:32:
In my perspective, this is taking the vision that I had in my advertising book, which I guess was 1997, and saying, oh, we have the technology for this now. We can put the right message in front of the right person at the right time. We have, we’ll call it traditional AI, machine learning, that can take all the attributes that I know about a given prospect, how often they come back to my website, which ads have they been exposed to, what white papers have they downloaded, are their friends on a knowledge graph, who are their influencers, and I can’t put that into a spreadsheet to make sense of it, but I can give it to a machine learning model and say when are they most likely to respond to what kind of message with which visuals and what to offer. And then Generative AI can create the creative for me to those specs and put it out there. That’s where we’re going. The first time I heard somebody say that in the future you would be able to ask your television set, I’d like to see a 90-minute movie with Tom Hanks and Glenda Jackson and Laurence Olivier and George Clooney in a heist movie that has Brad Pitt as a spy with lots of car chases and a happy ending. I said, you know, that’s crazy. Today that’s not crazy. And the actors are striking because it’s not crazy.
Tara Shankar – 00:39:59:
It is not crazy anymore. There’s so much has happened even in the, well, the fix is another thing, right? I mean, you could now create those entities and personalities with AI modeling. So while you spot on like, you know, creation of videos, creation of the entities, person, personas are so much easier now for sure.
Jim Sterne – 00:40:19:
And then there’s the final over the horizon, which is now possible, which if you go to my LinkedIn profile, you’ll see as one of my featured posts, it is five years ago, a video of me having a conversation with my personal AI agent who has agency and the conversation is about how I need to get my car repaired. And the agent recommends instead I buy a new car and has already negotiated for the model that it knows I want, because it overheard me talking to my wife about it and it can be delivered after my dental appointment that I’m about to be late for.
Tara Shankar – 00:40:54:
I’m good. Definitely looking into that video for sure. That’s awesome.
Jim Sterne – 00:40:58:
And what that means to marketing is that we as marketers need to create systems that will interact with prospects systems. So when a prospects agent says he might be wanting to buy a new dishwasher, that the manufacturer or the sales side of that equation has a listening device out there that can receive that intent from that agent and negotiate with it for appropriate style, size, price, installation and warranty. That’s going to be a significant challenge.
Tara Shankar – 00:41:32:
It is. Well, this is going to be a closing question. It’s been just amazing. But as you’re here, we’d love to know your thoughts because so many people are going to just learn a ton from the session. Looking back at your career and the vast changes you have seen in the industry, what would you say to those who are just starting their journey in the AI and the digital analytics field? It could be corporations. It could be individuals. We could just like top three things you want to call out before we close the session.
Jim Sterne – 00:41:58:
Top three things. Forget everything you know. Re-learn how to use a computer. Subject matter expertise is the crucial part. The human in the loop. So Generative AI is an absolutely fantastic, brilliant, unexperienced sidekick to a subject matter expert. Because just coming out of school, I could say, tell me everything about rocket engines and I won’t know if it’s hallucinating or not. The subject matter expert knows. So the advice to the student is dive into a domain, whether it’s business or research or medicine, the narrower your focus on an actual domain, the faster you can become an expert, a journeyman about that topic so that you can determine if the machine is giving you good advice or not. So subject matter expertise, understand where Generative AI is valuable and where it’s, you know, don’t ask it that kind of question. That’s important. And then the third piece of advice is forever and ever you may want to go back to school and take some psychology classes because it’s all about humans.

“Forget everything you know. Re-learn how to use a computer. Subject matter expertise is the crucial part. The human in the loop. So Generative AI is an absolutely fantastic, brilliant, unexperienced sidekick to a subject matter expert. Because just coming out of school, I could say, tell me everything about rocket engines and I won’t know if it’s hallucinating or not. The subject matter expert knows.”

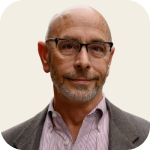
Jim Sterne
President at Target Marketing of Santa Barbara

Tara Shankar – 00:43:08:
That is so true. This human in the loop part is actually a very critical part, which I’m normally hearing, but also not the way you explain a few, taking two examples. I think it’s making total sense. And I think the whole combination of subject matter experts, bringing things together, having the understanding and also a sort of process to how you want to decide which use cases really need it, because it looks like you can apply it everywhere, but there will be some specific use cases you need to really kickstart with, especially for enterprises even more. Because I think everybody’s trying to take all of it and see, you know, whether it works for them across the domain, but I think they need to sit back and plan this better for sure.
Jim Sterne – 00:43:47:
I have a desire for you and your company that I’m hoping that people working with large language models will create sooner rather than later is instead of me asking for the machine to give me an answer, I give the machine a problem I’m trying to solve or a goal and it asks me questions and that will be prompt engineering.
Tara Shankar – 00:44:08:
That’ll be prompt engineering for sure. We here actually do goal-based nodes in our entire buildup, but certainly it’s more asking the question, getting back in return, but I think that’s amazing if we can have bi-directional discussions on this for sure. I mean, we understood the importance of runtime rather than build time. Everything is dynamic at the moment, but there’s still quite a bit of learning. We have a number of customers who have adopted, but this one, which you just called out, I think could be awesome to just have a reply back and saying, asking more questions. It happens in the voice a lot more. I think chat-based, we need to see how we bring that together, but suggestion taken, Jim.
Jim Sterne – 00:44:47:
And then the marketplace will be custom curated vector databases.
Tara Shankar – 00:44:52:
Cool. Well, the custom curated vector database I might add to that because that looks like an ending. Could you explain that a little bit more, Jim, before we go?
Jim Sterne – 00:45:00:
A vector database is semantic relationships that don’t fit in a context Window when in a prompt Window, curated vector database of payroll is offered to me and instead of buying and running a payroll package, instead I would say to the system, look at all of the States that my employees live in, go get the appropriate tax rules for those States. And at the end of the month, please run payroll. That’s programming. And the competition will be who has the most viable, trustworthy, authenticated vector database for payroll. They will be the ones who make the most money.
Tara Shankar – 00:45:43:
Totally. Awesome. Jim, on that note, I really want to thank you for your time today. It’s been amazing of a discussion, so much of insights.
Jim Sterne – 00:45:52:
Thank you, TJ. It’s been a great conversation. Really enjoyed it.
Tara Shankar – 00:45:57:
How impactful was that episode? Not Another Bot: The Generative AI Show, is brought to you by yellow ai. To find out more about Yellow.ai. And how you can transform your business with AI powered automation visit yellow.ai. And then make sure to search for The Generative AI Show in Apple Podcasts, Spotify and Google Podcasts or anywhere else podcasts are found. Make sure to click subscribe so you don’t miss any future episodes. on behalf of the team here at yellow ai. Thank you for listening.