Transcript
Tara Shankar – 00:00:25:
Hello and welcome to Not Another Bot: The Generative AI Show. I’m your host, TJ, and joining me today is David, who has been instrumental in shaping how businesses and enterprises perceive and leverage artificial intelligence, a very well-known name in the industry. Known for his deep understanding of AI’s multifaceted impacts and potential, David’s invaluable contributions have significantly influenced the growth trajectory of numerous organizations worldwide. His professional journey has been a fascinating blend of technical prowess and executive leadership. Currently, he is the global product lead at Google and the founder and general partner at DataPower Ventures. What truly sets David apart is his visionary perspective and thought leadership. He is a forward thinker who understands not just the technical facets of AI, but also its ethical implications and societal impacts. Critically important today’s day and time, he navigates the complexity of this field with a clarity of thought and a commitment to making technology accessible, equitable, and fair. Welcome, David, and I can’t tell you how excited to have you here on the show.
David Yakobovitch – 00:01:28:
Thanks, TJ. It’s a pleasure.
Tara Shankar – 00:01:29:
Awesome. Well, let’s get started. So, David, could you start by telling us about your journey? Like, I’m intrigued, frankly, just looking at the amount of things you’ve done, the voice you are in the industry, the things you have been doing to make AI adoption and journey so easy for enterprises and businesses. And from studying finance, information systems, and statistics to becoming the global product lead at Google and a founder at DataPower Ventures, what were some pivotal decisions or experiences that led you to your current position today?
David Yakobovitch – 00:01:56:
Well, I really appreciate everything you’ve shared TJ. And as we know, the data industry is always evolving. And for myself, I got involved just around the 2010 time period actually working in insurance and doing actuarial science work. And very early back then, our work was setting the right guardrails, setting the right systems in place when pricing insurance and developing systems that you put the consumer’s mind first, that you’re ensuring that you’re building responsible systems. Now, these systems were built On-premise. They were built on IBM Mainframes. They had different Visual Basic Scripts. They look very different than today’s AI for systems with Python being built responsibly with different interfaces and different Cloud environments. But it got me thinking the possibilities of where the data industry was headed and that led this whole journey the past 10-plus years where I worked at five startups in the data space, first to early stage ones, building early versions of Netflix and Snapchat, and then to startups where we did a whole ton of data science consulting both On-prem and Cloud for Fortune 500 and SMBs on how to build Data Science Workflows, on how to think about end to end solutions so that companies could accelerate their growth and unlock the power of their data. During that journey and startups, I launched DataPower Ventures to better accelerate the data economy by looking at these treasure troves of data sets and developer tools and apply insights through the machine learning and AI capabilities. And that journey has continued to accelerate. Today we have over 25 portfolio companies. And as we all know, the space is continuing to heat up today. It seems that AI is on everyone’s mind and beyond being involved in the venture capital space. Just about a year ago, I joined Google. I’m focused on their data products, specifically creating insights, supporting, understanding all about the data, ML and AI landscape. I do a lot of cross-product initiatives where we’re working with variety of models, variety of data sets, variety of testing. To build inclusive and to build accessible systems like you commented at the onset. So it’s definitely a journey that doesn’t stop. And I think that the key to our conversation today will be around these ethical implications. I’ve always been a big proponent inclusivity and that’s something that we’re starting to see on the forefront today. A lot of thought leaders, especially in the EU and others are beginning to bring this top of mind.
Tara Shankar – 00:04:47:
Right. So, well, I think one thing you just called out is the data science aspect of it. And given your journey, we’d love to know what first sort of sparked your interest in data science and how did that evolve into a focus on fairness and AI-first product or being particularly high aspect of the things. Can you share your particular motivation or maybe a story that inspired you to kind of go behind, you know, ethical AI and fairness and AI-first products?
David Yakobovitch – 00:05:24:
So for me, my passion has always been around mathematics. I’m one of those rare cases of both a product and VC leader who believes that everything starts to map. I love Yann LeCun for a lot of his focus on getting back to the basics. And for me, ever since middle school, high school, I did these national and international math competitions. Back then, you would actually do calculations by hand or with these TI-84 calculators. And it was so fascinating that before we call the data science, you would insert a new data point and then see how a linear regression would drift based on the impact of that one data point. Little do we know back then that this was called Model Drift. And this is about the explainability of models that today is being uncovered by tons of platforms, both at the big three Cloud and a variety of startups where trying to uncover, trying to be responsible about data. Once I moved on from those days, a lot of the work started of course with the classic spreadsheets that we’d see in Google Sheets and other platforms. And then went into the more robust systems of working with SQL and working with Python and R and his programming languages that just the sheer momentum of being there during the evolution of the Jupiter notebook of seeing now where any developer could unlock a linear regression or more powerful neural network on their local machine in a notebook and see those results became quite powerful. And that journey’s only continued. It was just two, three years ago that we could run our own image nets or convolution nets on our own notebook and see right there if something is a dog or a cat. Of course, fast forward through the GPT-powered craze of 2023. We’re now looking at much broader use cases, which expand across all sets of both enterprise and consumer businesses.
Tara Shankar – 00:07:27:
Lovely. And I think my worst interaction with machine learning while learning through the process was with R and Python. It’s just amazing, you know, how you explain the whole thing and the evolution of, you know, the notebooks from there. So totally. I’ve been linear regression, boosted decision trees, you know, those are like, we kind of, you know, build those or run those with R and integration to, let’s say some of the coding tools and SQL server. I’m glad that you called it out by SQL server days, our integration is where it came very close to learning it. So thanks for talking about that for sure.
Tara Shankar – 00:08:18:
So now that we know a little bit more about your thought process around data science and how you kind of came closer to ethical AI and why you care about it, could explain the concept of fairness in AI for its products and why is it so critical today with the means of maybe, you know, defining some measures in this context, some examples of how bias in AI can impact real-world outcomes and also some common sources of bias in AI today, like just your thought process around that.
David Yakobovitch – 00:08:54:
Yeah. So I think when you think about any automated product, the goal of automation is to create things that are better, faster for society. And when you’re creating AI-first products, these are often products that require a lot of nuance to make a decision. The early decision models were the ones that perhaps you and I would apply for a credit card or a home mortgage online and then get an instant result. You’ve been approved, or we need extra time to process your results. Those were models, but they often seemed very opaque and dark and it wasn’t clear what all the inputs and criteria were. And that I think is what spawned a lot of action from policymakers in the States and Europe and abroad to say, it’s great that we have automation. It’s great that we’re building better, faster systems, but are they truly better, faster? We need to unlock these inputs because as a society, we have the responsibility to the user to provide systems that are fair, where the user understands the inputs, where they’re given the support and feedback to know why a decision was made. And those decisions go beyond this case of getting approved for a credit card or a mortgage, and now seem to be pervasive all throughout society.

The challenge with these systems is getting it right for 90% doesn’t work. Getting it right for 95% doesn’t work. You need to get a 99-plus percentage. And these edge cases take a lot of data to be built up over time. That’s why I think to build fairness in AI, it starts with large data sets.

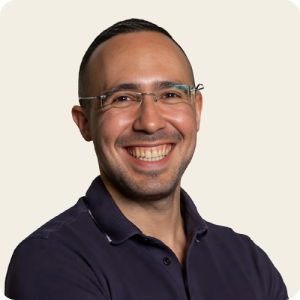
David Yakobovitch
Global Product Lead at Google

There’s a lot of startups today that work with computer vision to detect your face, anything from unlocking a phone to at the airport, being admitted or not admitted to go through an entry point, perhaps your Visa expired and that can now be done through automation with AI. But what if your face is mistaken as someone else’s face and you’re not admitted? You see here what happens is we’re coming into this conundrum of fairness with AI that often true. The benefit of the doubt is that developers are building with the best intent. I don’t think developers are saying, let’s build it so someone gets rejected and doesn’t get admitted. The challenge with these systems is getting it right for 90% doesn’t work. Getting it right for 95% doesn’t work. You need to get 99 plus percentage right. And these edge cases take a lot of data to identify and it takes that data to be built up over time. That’s why I actually think to build fairness in AI, it starts with large data sets. It doesn’t matter in the long run how large your model is. If this foundation model is, as we’ve seen many this year, some of them have been hundreds of billions of parameters. Some of them have been just about 10 or 20 billion. You don’t necessarily need incredibly complex compute and hyper tuning. But if you can get a very specific and broad data set, that’s the power to bring in more fairness to your models.
Tara Shankar – 00:12:12:
Yeah, I think that’s one thing, you know, as we’re kind of moving towards the large language model, especially in our domain even more, we’d certainly have seen how to kind of make a better one. And everybody is trying to do that with a smaller model size, less parameters and reducing hallucination and accuracy for sure. The one thing that’s coming to my mind though is how some of the common challenges which businesses face or may be facing with fairness in AI products, how do they overcome these hurdles today? Like, it looks like it’s a, even though the adoption could be exponential, but then there’s this massive concern around the fairness in the IFS products or the hallucinations or the biases. And how do you manage trade-offs between different fairness metrics?
David Yakobovitch – 00:13:12:
First, I think it’s important to think about where are these large language models or this generative landscape moving towards? It started with these foundation models just in the last couple of years and now the last six months, right? We saw the evolution of Bard and Bard 2 and PaLM and Med-PaLM 2 and ChatGPT 2, 3.5, 4, among many other participants in the space. And a lot of the changes here was to enable flexibility for the data inputs. I think we’re starting to move into a space much similar to the evolution of databases. Today, you and I both have been involved in the database industry. And we know that there’s a few hundred big databases out there, Cloud, On-prem. But originally, it started with maybe Oracle. And then it splintered off into these dozens and now hundreds of players. I think we’re going to move into a similar direction with the models. We’re going to have these big Bard and ChatGPT models that are going to get smaller as everyone tries to figure out how to create them for specific use cases. And over the coming months and years, we’ll move into a society where there’s hundreds of these foundational models. I think we’ve started seeing some early data points that indicate that. For example, Bloomberg created their own version, BloombergGPT, based on their fixed income and equities data. Google created Med-PaLM 2 , based on health care data. Very specific. And I think we’re going to see that across all scopes of the economy.

We’re going to have these big Bard and ChatGPT models that are going to get smaller as everyone tries to figure out how to create them for specific use cases. And over the coming months and years, we’ll move into a society where there are hundreds of these foundational models.

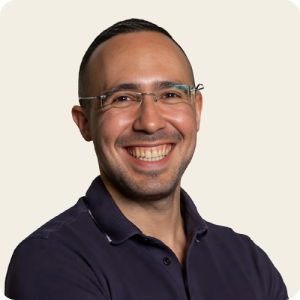
David Yakobovitch
Global Product Lead at Google

Now, if you’re a company, whether you’re a large enterprise or an SMB, and you are considering, how do I bring generative technology into our products to offer to our customers while building something that is safe, responsible, and fair? I think there’s two things to think about. Number one, what in your products or your offerings today, is there a gap that these generative products can enhance to make your product better or to offer additional value to your customers? So let’s say your product today is a legal brief reading software. So maybe you’re an enterprise company and you have software that finds key phrases and key review pieces and dockets. And all this is done through your fantastic technology using a variety of OCR and ML and AI techniques prior to the generative techniques. But now you’re saying, how can we offer more? Well, perhaps, and as I’m speaking about this use case today, right, so I’m offering this free counsel, perhaps it’s, oh, let’s take those dockets and then generate summaries based on the content or generate suggestions about decisions to make. Now, the challenge with generating anything not by a human is to not take it at face value as 100% true. And this is the early issues we’re experiencing in the generative movement. There was a case in the early part of 2023 where there was a lawyer who was presenting a case to a judge and they just literally were presenting everything that the foundation model gave to them without even reviewing it. And it was quite shocking, the judge actually scolded the lawyer and actually suggested to even disbar them for going so much that case. And so I think it requires a certain level of wisdom to work with these models and it requires a collaboration between humans and machines. Even if fast forward a couple years, these models reach perfection in whatever perfection is, there will be new edge cases. You do need oversight and support to ensure that they’re having the right levels of trust and those guardrails. So I share that case. That’s I think part one about how do you implement it or consider implementing it to your product. Part two is about, you mentioned TJ about all these metrics. And as we know in the data science world, there are dozens of different inputs that you can consider, you know, you could start with the basic accuracy, precision, recall and standard metrics. But the challenge with your model is there’s so many things that may get right and so many things that may get wrong. And it’s to your benefit to actually track that data for each metric and over time, see where that performance drifts, whether it improves or not, based on the new data inputs and the training techniques or technology that you use to get better results. You may not get tens across the board or 100 percent of everything. Maybe early on, one metric is 40 percent and then that is 80 percent. I think, again, the goal is progress, not perfection. And as long as we’re building these systems where it’s humans and machines together, that we’re going to go in the right direction. Otherwise, when we choose to exclude a human, that’s where we see the EU Commission and other bodies say not so fast. We need to consider policy and the impact on society.
Tara Shankar – 00:19:06:
Absolutely. And I think that’s the more we’re going towards automation even here. Right. I think one of the key things we’re trying to talk about and discuss while we talk to our prospects and customers is how do we bring the human in the loop to make the whole journey more productive. Right. In our scenario, we talk about agent assistance. How do we make their life easier? Like to your point, having a specific LLM for maybe summarization, one for maybe just Q&A, I think that tremendously helps because it’s focused. They get more accurate or at least close to accurate answers, which eventually helps them either drafting a better response, understand the sentiments, summarize the whole conversation that might have happened before it reaches them. So I think it’s tremendous impact. But the whole total automation, 100%, I think that’s something which I’m assuming to your point, maybe it’ll come in some time, but it still needs its own tons of regulations to go through in different industries and in others scenarios, the hallucination to deal with is the right assumption, David.
David Yakobovitch – 00:20:04:
I think the challenge with the pace of innovation with foundation models is they’re both going to unlock opportunity, but they’re also going to have a disruption curve. And there’s early data showing that counter to the popular belief that all this AI is going to create more jobs. The short-term disruption is actually going to be quite painful, and we are seeing that across the board. One example is an education company. So this is public data. Maybe if you’re someone who has children in college or you’ve gone through, perhaps you’ve been to some of these platforms in the past where you get study tips and recommendations and this platform is actually called Chegg. Maybe you’ve gone there, you’ve looked at textbook, you’ve got reviews or feedback. Well, Chegg actually has a pretty healthy business, pretty predictable, cyclical customers coming in on the school cycles. Well, the early data showed that since these large models came out and offered these free tutoring-ish services where someone could say, “‘Hey, is this the right solution to my math problem?’ And then the model says, “‘Oh no, try fixing this.'” That Chegg’s business went down year over year in 96%. And now Chegg is scrambling to create their own Chegg AI model among other things. But so of course, in the world of free capitalism, there’s the opportunity for any business to be out there in support, though there is some long tail effects that we’re not aware of and that disruption will trickle down all across the global economy. In this example, thinking about Chegg, well, that’s the one proof point that we see publicly. But what about all the private tutors and SAT and ACT companies and organizations that have run a whole industry to support education success for high school and college admissions?

The challenge with the pace of innovation with foundation models is they’re both going to unlock opportunity, but they’re also going to have a disruption curve. And early data is showing that counter to the popular belief that all this AI is going to create more jobs.

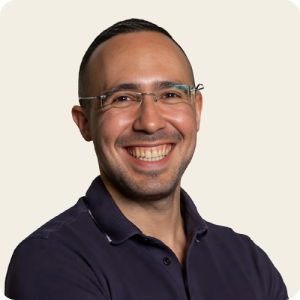
David Yakobovitch
Global Product Lead at Google

It’s too early to tell what that disruption looks like. And while some of that may be warranted, you may have learners who are excited to be self-disciplined and have their super powered assistant, to others, it might be too disruptive in the short term if we don’t offer adequate opportunities to rescale, upscale and expand new opportunities in society. So I think there’s a lot to consider with these models. And I think for those reasons, I’m in support with the EU’s policy. They just came out towards the end of Q2 with their review on how transparent and fair foundation models are everywhere from the Bards to the ChatGPTs and others. And I think their scoring of all these dimensions is a step in the right direction.
Tara Shankar – 00:22:53:
Awesome. Thanks for the insights there. I think now that we’re talking more about bias in AI systems, and certainly it all comes from the data, the way we train it. So as we know, the major source of bias in the AI system is training data. How do you approach the task of collecting and preparing unbiased data? And can you also talk about some of the pitfalls in data collection that can introduce bias and how to avoid them as enterprises take this journey? It’s an established practice to an extent, but I think bias and then certainly getting outcomes off it is equally critical to consistently being discussed. And lastly, can you speak through the challenges for identifying and handling hidden biases in the data?
David Yakobovitch – 00:23:31:
So when we’re thinking about data for models, collection is definitely one of the key elements that is all about getting new data and constantly having those inputs are critical so you can refine or improve your model. If you don’t have new data, right, you don’t have a new source of water to drink from. And so it’s critical to have that to improve your models. Now, when you’re gathering a data set, depending on how questions are asked can impact how that data is collected. It could be as simple as maybe you have an API is providing certain inputs. This is from an iPhone or an Android device. This is from a web browser or a Mobile App. By not collecting certain information, there’s certain insights that you would not be able to surface in the first place. So it’s important to collect those insights. But second, it’s important to be responsible with collecting them. So I don’t think data collection in itself is the biggest risk that we’re seeing in the space, but it’s more about training on the data that’s collected and making sure that the right techniques for anonymizing certain information and keeping certain information private, I think that’s an area that we need more focus on. If an example of those app usage data provided information such as certain people’s demographics, where people live, this can create problematic issues once the models are trained. One really well-known public case a couple years ago is with one of my favorite sports, running. So I do a lot of half marathons and marathons. I love to run. I love to track my running data. I love to see all the insights here. The leading running platform is known as Strava. So Strava is this app. And for those who run, you’ll turn on the app and it’ll track all your data. And it submits it to the platform. And then you could socially share it with others and then see certain insights on where would recommend you to run if you’re in a certain area. Well, what so happened is that when some people got to a certain area, it recommended a certain place to run. And they were curious because that wasn’t the spot that was necessary nearby or accessible to most people. Well, it just so happened that that spot was a specific military base of the United States in an area that most people didn’t know about that was classified. And you can see how not thinking about releasing this feature as a product manager, the unintended consequence was like, uh-oh, some of this data got out there. What if it got into certain hands? Now, what Strava did overtime to solve for this, I don’t know if it’s the full solution, but they did take down that feature. So they recommend their left. But now when you share your running path with a friend, they intentionally exclude the first quarter and the last quarter mile of however far you run so that if someone finds you in real-time, you know, your privacy is secure. So I don’t know. Sometimes when these features are built, I’m always wondering, what’s the use case that you’re building this feature? Do you need it? Are you adding value? And I like that they’ve met the criticism and have provided somewhat of a solution, but there’s a lot more to encounter there. And so this comes back to that whole point about how you consume your data into the model and what those outputs could be.
Tara Shankar – 00:27:55:
Amazing. While you were speaking, this question kept coming to my mind, and we can go a little bit more technical too, and just for one of these questions, now that talking about different methods to also reduce bias, I think the mitigation part of it, right? If you have bias, what do you do? Right. So now we know there are some pre-processing, in-processing, post-processing methods, which exist to reduce bias. First, I would love your thoughts, and if you can elaborate a little bit on that. Second, some successful applications of post-processing methods, you know, whether it’s calibration or something else. And just like the way you are giving examples, I love the examples, David, by the way, just the use case and, you know, taking example help so much easier to understand. So any examples for processing methods, such as modifying the learning algorithm or the function itself and its effectiveness, any sort of knowledge sharing, which you may want to call out, to maybe the data scientists or the people who are working day in, day out on, even the data engineers like processing and pre-processing and post-processing of the data to reduce bias.
David Yakobovitch – 00:28:55:
Yeah, so I think what’s really fascinating today is when you think about the generative AI landscape, there’s a variety of practitioners needed to make models available and to be able to work with those, you need, for example, first, the data analysts who are collecting and nurturing that data to then pass it on to these data engineers, which are doing a lot of this pre-processing, ensuring that data is at a good level of data validation or data sanity and that cleanliness is making sure, is the data complete? If you’re collecting, for example, let’s say we have another scenario where you’re creating your own model to generate images and perhaps these images are very specific. You’re focused on generating objects in outer space and so you’re gonna collect data. Well, how complete is that? Do the images that you see, first off, are they representative of the model you wanna build? So perhaps you have an image of a dwarf galaxy and then you have an image of actually a Planet Earth toy being held by a toddler. So there’s a consideration, right? Will the model know that that Planet toy Earth is not actually in outer space? It may not. It requires that oversight. And there are tools you can use to generally identify real quickly like, hey, is this yes, no in the first criteria, but it will require that additional human testing, human validation or in the loop. I think post development of a tool, it’s all about, again, getting it tested, getting it tried out because there are gonna be gaps based on your data. So you might say generate a solar system and if all the inputs weren’t there before, these models will attempt to find the nearest prediction or find the nearest scenarios that are approximate. Really when it comes down to it, today the movement, this generative AI movement, everyone’s packing up into a pretty little package that here’s this LLM or this model that can do XYZ, but if we really drill it down to fundamental data science, we’re really just looking at nearest objects. We’re looking at these k-means or how is a point to another point in the whole collection of your data sets. And it’s for this reason, right? It goes back to that scenario I mentioned at the onset of our episode today about what if I did that linear regression by hand and I added a new data point and then there’s that drift or there’s that shift in the model. That’s exactly what happens when we insert new data points into building these foundation large language models. It’s to the point that if you were a data science team managing a model for a company and you’re collecting fresh data from the lake and suddenly 80% of the new data is of a completely different type of image than before. Suddenly, maybe on TikTok, it went viral, kids playing with planets Saturn and Jupiter and all these images and videos start coming into your data set. You’re gonna start seeing perhaps this performance drift, this decreased performance, feedback from users. No, this isn’t outer space. I don’t want someone else’s kid in my photo. So you can see here the narrative I’m describing that these models aren’t truly sentience and they do require a lot of testing. I think one of my biggest cautions to companies that want to integrate generative features into their product, it’s not launch it and it’s done and it just lives autonomously forever. You will need software engineer, data scientists, perhaps professional services company to help manage or software, right? To manage and support the end-to-end life cycle. Just like when you integrate an application with a database, you don’t touch it, set it and forget your database forever. Things start to go wrong. software always toils. There will always be degradation, broken queries. The same is occurring today with these models and will continue to occur. So it’s beneficial to think about that end-to-end system.
Tara Shankar – 00:33:28:
Coming back to fairness metrics in AI-first products, could you discuss the role of fairness metrics in AI-first products and the challenges of choosing and implementing them, especially if you could talk through a situation where two fairness metrics can contradict each other and how you would handle it or probably some ideas of doing so. And second, how are they incorporated into the model training and evaluation process given they would need to be doing so given the discussion we just had because it may impact the overall outcome.
David Yakobovitch – 00:34:47:
So the challenge with fairness for a model is it depends on who your audience is or what the use cases you look at. Let’s say for example, you have one model and it’s gonna be some sort of text generation model for your company. Let’s go back to the example earlier of the BloombergGPT. So Bloomberg created this large language model called BloombergGPT that includes a treasure trove, finance and equity data. And the purpose is, well, for those of us who know Bloomberg in the financial industry, they first got famous for these Bloomberg terminals where their developers could type shortcuts and code and find price action to make a trade and generate profitability from Bloomberg as an organization or support other traders or hedge funds or quant shops. So could they have this BloombergGPT model where instead of just searching with a query, you could type into that search, Google and then get some recommendations based on things occurring in the news that day or other parameters. And this could be beneficial, especially if the data sets large and it’s public and it’s universal. There’s a ton of information available on stock symbols in the Fortune 500. And so you would gain more insights here and perhaps the software engineers that are managing BloombergGPT are seeing that usage and the benefits. But what happens if we’re looking for more obscure over-the-counter symbols or penny stocks or reverse splits? There’s some really nuanced cases. The data may be incomplete and the data may actually be so incomplete that it could cause a poor decision-making a choice from a trader and without the right language to suggest that this is not financial advice. This is generated by a machine. This is here to support you as a data point is a chance that decisions might be made slower or decisions may be made that reduce profitability. And so when we’re thinking about the metrics that are being captured, this will vary from organization to organization. It may be as straightforward as, do we have an inclusive metric where we’re not getting bug reports that we’re seeing vulgar or profane or racist content? So that could actually be like a metric, the number of bugs occurring monthly went from 20 to 10. Okay, it looks like we’re doing better here potentially, but could it be that developer productivity has sped up? Back to our case with the lawyer working on the legal briefing software, could it be that now billables are up because the lawyer is able to work a little bit faster? They’re able to get the summaries quicker, could be beneficial, but could they be making the wrong decision or could their insights actually be inaccurate? And so I think it’s gonna be critical, whichever organization you are, whether you’re gonna use an off-the-shelf tool, whether you’re gonna build a tool, buy a tool, you do wanna determine what are those north star metrics for your customer or your user before you implement the tool and then start to track those and see if they’re heading in that direction you want. Saving money, making money, customer success, going up, bug reports, and there could be dozens of other variables that you choose. I think it’s important though that we choose those metrics early on because you can’t measure what you don’t track and otherwise you’re like, oh, I think it’s going well. It looks like our revenue is up to the right. Yeah, but is that from the model or something else?
Tara Shankar – 00:38:35:
Very rightly said. And I think one thing that comes to my mind on top of that is we’re talking about the ethical considerations when designing and deploying, fair AI-first products, but also there is this consistent requirement from customers to have more accuracy, efficiency, privacy, response times to their queries. How do you see that balance coming together? Is it like, is there a trade-off to try and maintain building fair AI-first products or being more considering the ethical aspects of AI? Do we have to have like some trade-off between accuracy, efficiency, response times, and privacy?
David Yakobovitch – 00:39:12:
So while from a product perspective, we always think of trade-offs from a user perspective, I think it’s about managing expectations in the sense that the last six months, everyone now, everyone’s mom, everyone’s grandma, everyone’s great grandma knows about generative AI. It like took the world by storm, but it doesn’t mean the software is perfect. It’s not like you take out your Pixel phone or your iPhone and you marvel at that piece of hardware and software, how flawless it is that when was the last time on your Google or Apple device, an App crashed and your phone became unusable, like the blue screen of death we see from Windows machines. We don’t see that too often anymore, but we’re gonna see a lot of early issues with the models. And I think because this industry is so nascent and evolving so rapidly, we need to be willing to give them time to improve and to know that this journey will be taking the next year to five years, depending on the models to get there. I think we do need oversight, of course, so we maintain that fairness, because if we don’t, we’re going to encounter issues like described about the earlier case of applying for a credit card or a home mortgage and not knowing where you rejected because the model thought that you were an alien or was it because you had too much debt to income ratio, so it was taking a very measured approach there. So I think that’s something to think about. And it’s all of our responsibility to respect this opportunity. Unlike previous cycles of technology, which were quite theoretical in the AI space, it’s quite pleasant to see this transition towards a preeminent technology that everyone can use and sign on to your search engine today on Google and get a generative response. I think it’s the early days. And my general take over time is think about job descriptions in the last 20 years. They got to a point where everyone, whether you were a secretary or an executive, usually the job description set experience with spreadsheets, working with wrangling data, doing some basic insights. Today in 2023, if you ask someone, hey, can you work with a spreadsheet? They’re like, oh yeah, sure. I can type some data, do a formula. Well, I think that’s the space we’re gonna move towards with these large language models. We’re gonna move to a space where there’s a ton of products that you use, maybe use Adobe FireFly to generate images and you’re gonna need to do these prompts. So instead of coding in a spreadsheet, you’re gonna write a prompt, create an image of the solar system and then you see that, or maybe more advanced prompts, right? Create the image of the solar system in a panoramic view with HD macro lens. And so I think it’s also to our benefit as a society that we’re gonna need to enable the user, respect the user to be able to successfully use these products. Whenever we’ve had issues in the past with software and hardware, what do you do as a software engineer? Do you blame it on the user? It’s the user’s fault. They didn’t do it right. Come on, we built it right. We answered the requirements. I think it might be the UI, the UX, the experience, right? Something there, we have to go back and understand what broke, what didn’t happen. And so we can ship these models, we can build these tools, but we have that responsibility to enable each and every one of our customers and users to succeed and thrive with them. And I think if we do that, both from the data perspective unfairness, the process of building and maintaining these models, and then ensuring that everyone’s successful using the technology, while the short-term remains unclear, a lot of ambiguity, a lot of change in this industry, I think in the coming years, we’ll all have our own version of these super-powered assistants that we’ll be using to accelerate different parts of our life.
Tara Shankar – 00:46:27:
Well explained. Well, David, I’ll take one last question for you. I think this is critical to land and it’s been just amazing so far, the entire discussion, and this is probably the last question, which kind of brings everything together. One is, and it’s purely like your prediction about how the concept of fairness in the IFRS products might evolve in the next five years. I would have asked 10, but with the pace of innovation, I think in a five years makes sense and particularly in light of technological advancements and evolving societal expectations and what implications this could have for businesses. And why they should really care about what are things they could start doing now so that they don’t have to redo things later on, because everybody also wants to jump into the, this journey and the wave of generative AI and large language models. So we’d love to get your final thoughts on those before we sort of say goodbye today.
David Yakobovitch – 00:47:19:
So I think the way that we build a more humane future and improve fairness for models is through continual validation, continual testing, continual providing of that feedback. And it’s an uncomfortable thing to do as engineers, as users, we like to ship product and use product and just have it work, but we often don’t want to provide that feedback if it’s not comfortable. So I think it’s going to require companies that are shipping generative products to make an easy experience for users to share their feedback and to make them know that you value that feedback and that their trust and then their support for your product means everything. Because the only way to get products right, to be fair, is to validate with these edge cases. And although for the purposes of the show, I keep mentioning them as edge cases, they’re anything but edge cases. We’ve seen decisions this year in the court system where the Supreme Court knocked down affirmative action because they believe that’s an edge case. But is that so? Millions of people would say no, right, that there’s benefits to the world and society to level up the playing field, to bring fairness to everyone. And when I see humans on the court who are elevated to a position of authority to say affirmative action should be knocked down, but who are you to say that? Who says your legal judgment is above another lawyer? What would ChatGPT, what would Bard say to turning down affirmative action? They might say it’s actually beneficial for society, for fairness and inclusion. So I think this framework where I’m thinking of using super-powered assistance and having inclusion in systems, it’s going to be essential for us to get products right in the coming years. But the only way to do that is to take the approach that the EU is taking. We have to have policy first. We have to have openness and fairness for the models, because if we don’t, we’re going to see the early blunders that we saw from ChatGPT, where a persona like Sydney came out that almost caused people to commit suicide. I mean, these are things that could have been completely prevented, but there was carelessness and recklessness from some users and no organization is going to get it right in the short term. But it’s having the commitment of leaders who take inclusive principles, accessible principles and inclusion by design that will get us there in the coming years. But it’s going to be a rocky road and every model is going to be different. So the challenge is understanding that one model is not all of them. So when someone comes to love Adobe FireFly and says, you made for me this beautiful picture of the solar system. But when I used mid-journey, it didn’t happen. Wow. Generative AI tech must be bad. No, it’s a different model, right? We need that feedback to understand what’s going on. So it’s definitely a journey. I’m excited for the road we’re going to take. I can’t wait for the future where all of us are going to be doing some prompt engineering ourselves and building hopefully a better and faster world.
Tara Shankar – 00:50:54:
Amazing. I’ll just leave right at that thought you just mentioned. I think that’s perfect ending to this podcast. Visit for sure. Well, on that note, David, I just want to thank you for being here on the show. And I can’t tell you how informative and deep these discussions were and so clear in terms of the fairness we want to bring in the AI products and why we should consider that first. Totally an experience and then learning for me as well. And I’m sure it’s going to be for the audience. So I want to thank you so much for your time and hope to kind of bring have you again to just discuss this further in some time when all of these adoption on the ethical side of the business for the eye gets even more mainstream further from here. So thanks for your time today.
David Yakobovitch – 00:51:34:
Beautiful. Thanks for having me, TJ.
Tara Shankar – 00:51:36:
Thank you.