Customer service metrics are the guiding compass for companies navigating the vast sea of customer interactions and are crucial in pinpointing what truly resonates with the audience. However, with a myriad of metrics available, determining which ones are pivotal to enhancing your customer service and ensuring your efforts yield tangible results is essential for delivering an exceptional customer experience.
Recognizing the impact of customer service is non-negotiable. A report from Zendesk indicates that after one or more negative experiences, around 80% of consumers are inclined to switch to a competitor. This stark reality highlights the critical need to keep a pulse on your customer service performance metrics to retain customers and stay competitive.
In this article, we’ve meticulously identified 12 essential customer service metrics that will offer an unobstructed view of your service’s performance and help you in shaping a superior customer service strategy.
Join us as we dissect each metric, understanding what customer service metrics are and how to measure customer service metrics effectively. By the end of this enlightening journey, you’ll not only comprehend but also excel in employing customer service metrics to revolutionize your customer interactions. So, let’s set sail into this comprehensive guide and redefine your approach to customer service excellence.
Related must-reads:
- 10 Reasons why is customer service important in 2024
- 15 Key customer service skills (How to develop them)
- 10 Great customer service experience examples in 2024
- Should your business outsource customer service?
What are customer service metrics?
Customer service metrics are the key performance indicators that track, measure, and analyze the efficiency and effectiveness of customer service. They are the pulse checks of customer service health, providing real-time insights into how the customer support team interacts with customers and handles their inquiries.
These metrics range from satisfaction scores, which reflect the customer’s overall contentment with the service, to operational metrics like average response time and First Contact Resolution (FCR), which focus on the speed and effectiveness of service strategies. By understanding these customer service performance metrics, from the time taken to respond to a query to the resolution rate of a query, businesses can create a clear, actionable picture of their service’s performance.
Why are customer service metrics important?
The importance of customer service metrics extends far beyond mere numbers on a dashboard; they are the critical indicators that shape the future of business. Happy customers often lead to thriving businesses, as they are more likely to return, refer friends, and make repeat purchases. Conversely, unhappy customers have the potential to harm your reputation significantly, sometimes with just a single negative review or social media post.
This dynamic landscape makes it imperative for businesses to keep a keen eye on performance metrics related to customer support quality. By consistently monitoring these metrics, you can detect early signs of trouble and implement corrective actions swiftly, preventing minor issues from snowballing into larger problems that could damage customer relationships.
Also, customer service metrics help in finding the answers to vital performance questions, such as:
- How accessible and user-friendly are customer engagement channels?
- Are sales processes streamlined and efficient within the organization?
- How effectively support teams are providing top-tier service?
- Does brand, product, or service messages resonate with customers?
- What is the genuine perception customers have of the company?
By addressing these questions, customer service metrics offer a transparent view into the heart of customer experience. They are the tools that help fine-tune your strategies, elevate customer interactions, and drive your business towards continued growth and success.
12 Customer service metrics to measure in 2024
As we stride into 2024, understanding and leveraging the right customer service metrics is pivotal for any forward-thinking business. Here are 12 essential metrics that will be instrumental in measuring, understanding, and enhancing your customer service throughout the year.
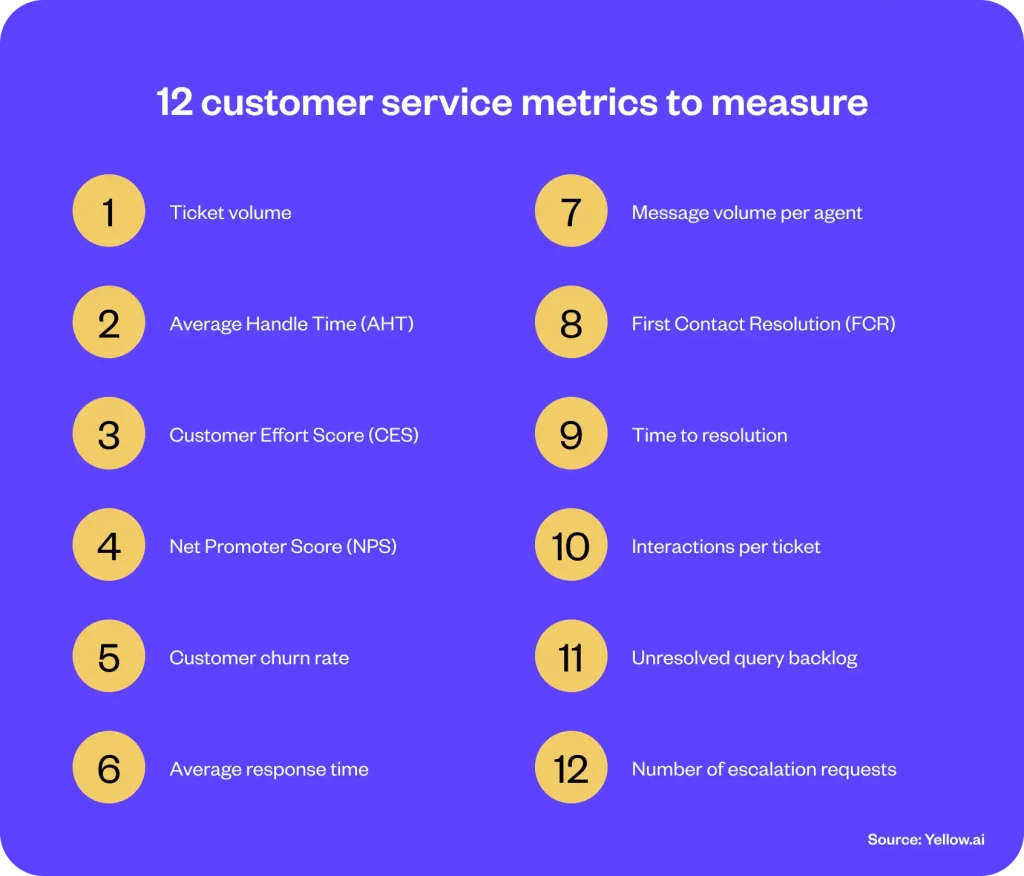
Metrics #1 – Ticket volume
Ticket volume quantifies the number of customer support requests received over a given period. It’s a direct measure of customer interaction and service demand. While high engagement can be positive, an excessive ticket volume may signal underlying issues with your product, service, or user experience. Monitoring this metric helps in identifying trends, managing resources, and maintaining the quality of customer service.
Method of calculation
To calculate ticket volume, tally the total number of support tickets received daily, weekly, or monthly. Comparing this number over different periods helps identify spikes or trends. It’s also beneficial to distinguish between new and resolved tickets to understand the team’s efficiency in addressing customer queries. For a nuanced view, consider segmenting the volume by the nature of inquiries or by specific events, like new feature releases, to pinpoint their impact.
Tip to improve ticket volume
Understanding ticket volume aids in resource allocation and strategic planning. A sudden increase might necessitate additional support staff or indicate issues requiring immediate attention. Analyzing the reasons behind ticket spikes — whether they’re product-related or due to service issues — allows you to address root causes and improve your offering. By preparing for anticipated increases in ticket volume, such as during new releases or promotions, you can ensure your team is ready to provide prompt, quality support. Regularly monitoring and responding to ticket volume trends helps in delivering a more responsive, proactive, and ultimately satisfying customer experience.
Increase CSE efficiency with Gen-AI-powered inbox
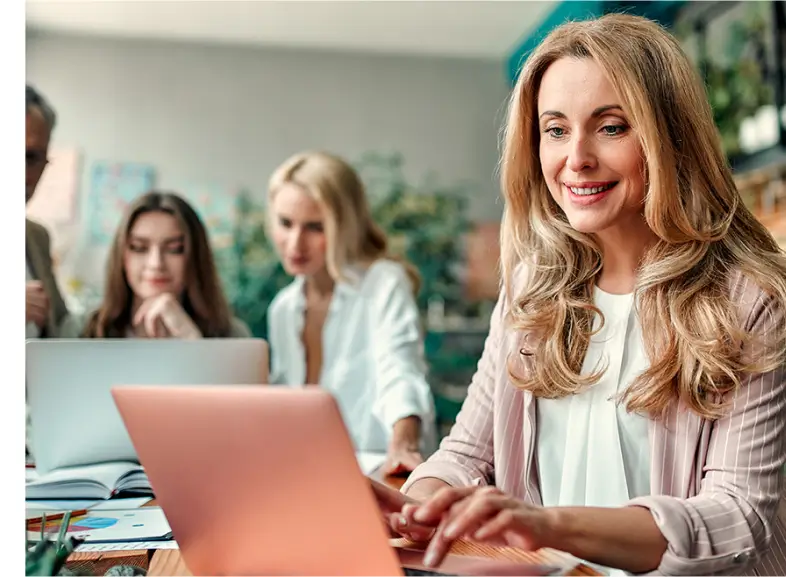
Metrics #2 – Customer Satisfaction Score (CSAT)
The Customer Satisfaction Score (CSAT) gauges customer contentment with your products or services. This metric involves questions about different aspects of the customer journey, with responses on a scale of 1-5 indicating satisfaction levels. CSAT reflects immediate customer sentiments and is a key indicator of service quality.
Method of calculation
Calculate CSAT by focusing on satisfied customers — those rating 4 or 5. Use the formula: (Number of 4s and 5s / Total survey responses) x 100 to get the percentage of satisfied customers. This method is preferred as the top ratings are strong predictors of customer retention.
Formula –
CSAT score = (No. of positive responses / No. of total responses) * 100
Tip to improve customer satisfaction score
CSAT is crucial for real-time feedback on service performance. Regularly measuring and analyzing CSAT helps pinpoint areas for improvement and guide strategic decisions. Additionally, understanding the reasons behind low scores through follow-up questions enables targeted actions to elevate the customer experience. Monitoring CSAT against industry benchmarks offers insights into competitive positioning and areas for enhancement.
Metrics #3 – Customer Effort Score (CES)
The Customer Effort Score (CES) is an essential customer service performance metric that measures the effort required by a customer to get an issue resolved, a request fulfilled, or a product purchased/returned. The premise is simple: the less effort customers have to make, the more likely they are to remain loyal. CES sheds light on the ease of interaction with your company and is a significant predictor of customer retention.
Method of calculation
CES is typically calculated using a single survey question such as, “On a scale of 1-7, how much effort was required to get your issue resolved?” Customers respond with a numerical value reflecting their perceived effort. To calculate the overall CES, take the average of these scores. Remember, a lower average indicates a smoother experience for the customer.
Formula:
CES % = (No. of respondents who rated high / No. of total respondents) * 100
Tip to improve customer effort score
Understanding your CES is invaluable for pinpointing areas in the customer journey that are causing friction. If scores indicate high efforts, dissect the process customers go through to use your product or service and assess each step individually. Look for patterns in the data — are there particular touchpoints where customers consistently report high effort? By identifying and addressing these pain points, you can streamline the customer experience, making it more effortless and thereby improving loyalty and satisfaction. Additionally, keeping your customer service channels readily accessible and maintaining up-to-date self-help documentation can significantly reduce customer effort and enhance the overall experience.
Metrics #4 – Net Promoter Score (NPS)
Net Promoter Score (NPS) is a key performance indicator in customer service that measures customer loyalty and the likelihood of your customers recommending your company, product, or service. It’s often regarded as the gold standard customer experience metric because it provides a clear and direct reflection of customer loyalty and sentiment. A higher NPS is desirable as it indicates a larger proportion of customers who are not just satisfied but are enthusiastic advocates of your brand.
Method of calculation
NPS is calculated using a simple formula: subtract the percentage of detractors (customers who scored 0-6) from the percentage of promoters (customers who scored 9-10). For instance, if you have 70% promoters and 10% detractors, your NPS is 60 (70-10). Responses from 7-8 are considered passives and are not directly factored into the score. The ultimate goal is to push your NPS as close to +100 as possible, indicating overwhelming customer loyalty and satisfaction.
Formula –
Net promoter score = % of promoters – % of detactors
Tip to improve net promoter score
Understanding your NPS is crucial for gauging the general sentiment towards your brand and identifying how many of your customers are truly engaged and likely to spread positive word-of-mouth. A low NPS indicates a need for a thorough evaluation of not just your product but the entire customer journey. Investigating the reasons behind the scores, especially from detractors, can reveal specific areas for improvement. Enhancing these aspects can turn detractors into promoters, boosting your NPS, and consequently, your brand’s reputation and customer loyalty. Additionally, comparing your NPS with industry benchmarks can provide a clearer perspective on your standing and inform strategies to excel in customer loyalty.
Metrics #5 – Customer churn rate
The customer churn rate, often referred to as customer attrition, quantifies the rate at which customers cease their relationship with a business within a specific timeframe. It’s an essential barometer of customer contentment and allegiance. A diminished churn rate generally indicates higher customer satisfaction and long-term business health, while an elevated churn rate can be a red flag, signaling potential issues in product, service, or overall customer experience.
Method of calculation
To determine the churn rate, divide the number of customers lost during a given period (such as a month, quarter, or year) by the initial total customer count at the start of that period, and then multiply by 100 to express it as a percentage. For example, if you commence the month with 100 customers and 15 depart by the end, your churn rate is (15/100) * 100% = 15%. Consistently tracking this rate over time can uncover patterns and provide insights into the impact of customer retention strategies.
Formula:
Customer churn rate = (No. of churned customers during the period / Total number of customers at the start of the period) * 100
Tip to improve customer churn rate
Mitigating your churn rate is fundamental for sustaining a thriving enterprise. Understanding why customers depart is key. Dive into their feedback and service interactions to pinpoint prevalent issues. Direct conversations with departing customers can offer profound insights into systemic problems and individual grievances. By addressing these concerns through enhanced service, improved product offerings, and proactive customer engagement, you can foster a more loyal customer base. Regularly seeking and valuing customer feedback is not just beneficial; it’s essential for adapting and evolving in a way that resonates with your customer’s needs and expectations, thereby reducing churn and bolstering your business’s longevity and success.
Metrics #6 – Average response time
Average response time measures the duration between a customer’s inquiry and your team’s first response, typically in minutes or hours. This metric signifies your commitment to valuing the customer’s time and providing a positive experience. Quick response times are crucial for customer retention and satisfaction, with many expecting a response within just a few minutes.
Method of calculation
Calculate the average response time by subtracting the time of the customer’s initial request from the time of the first response. Average these durations over a set of interactions to gauge your team’s performance. For example, if a customer’s request at 1:00 PM receives a response at 1:15 PM, the response time is 15 minutes.
Formula
ART = Total time taken to respond / Total number of responses
Tip to improve average response time
Optimizing average response time is crucial for making customers feel heard and valued. Setting clear internal goals and expected response times sets the stage for efficient communication. Identifying and addressing reasons for delays, like understaffed teams or inefficient processes, is essential. A multi-faceted approach, including training, technology, and continuous monitoring, can significantly improve your response times, building a reputation for excellent customer service and encouraging customer loyalty.
Metrics #7 – Message volume per agent
Message volume per agent quantifies the number of customer messages or inquiries each team member handles, typically calculated per day, week, or month. This metric offers a transparent view of individual workloads and productivity levels within your customer service team. Unlike simply counting closed tickets, message volume provides a more nuanced understanding of the workload and engagement of each agent.
Related read: AI Agent Assist – Elevating customer support efficiency
Method of calculation
To calculate the message volume per agent, tally the total number of customer messages or inquiries an agent handles and divide it by the time period (day, week, or month). Comparing these numbers across your team can highlight disparities in workload and efficiency. For instance, an agent handling 200 messages per day is carrying a heavier load compared to one dealing with only 10. However, it’s crucial to consider the context and complexity of these interactions, as not all messages require the same effort or time to resolve.
Tip to improve message volume per agent
Monitoring message volume per agent is essential for balancing workloads and ensuring optimal team performance. A consistently high volume might indicate the need for additional staffing or better resource allocation to prevent burnout and maintain service quality. Conversely, a lower-than-expected volume could signal underutilization of resources or a need for further training to improve efficiency. Striking the right balance ensures that each agent can dedicate sufficient time and attention to each customer, leading to more effective resolutions and a better overall service experience.
Metrics #8 – First Contact Resolution (FCR)
First Contact Resolution (FCR) is a critical metric in customer service that measures the percentage of customer inquiries resolved during the first interaction. A high FCR rate is indicative of efficient and effective customer service, as it means customers get their issues resolved quickly without the need for follow-ups. It’s not just a measure of efficiency; it reflects customer satisfaction and operational effectiveness.
Method of calculation
To calculate FCR, divide the number of incidents resolved on the first contact by the total number of incidents. For example, if you received 100 inquiries and resolved 75 on the first contact, your FCR rate is 75%. You can also measure the average number of replies it takes to resolve an issue and the time from ticket submission to resolution for a more comprehensive understanding of your FCR.
Formula
FCR % = (Total issues resolved on first attempt / Total issues resolved) * 100
Tip to improve first contact resolution
Improving FCR directly enhances customer satisfaction by delivering quick and effective solutions. It requires well-trained staff and efficient processes. A high FCR rate reduces follow-up work, increasing customer trust and loyalty. Conversely, a very high FCR might indicate over-reliance on customer service for simple issues; enhancing self-service options like FAQs could help. Continuously monitoring and improving FCR is vital for boosting customer satisfaction and operational efficiency.
Metrics #9 – Time to resolution
Time to resolution is the duration it takes to fully resolve a customer inquiry from the moment it’s received until it’s considered solved. This metric is crucial as it significantly impacts customer satisfaction; the quicker an issue is resolved, the happier the customer generally is. Efficient resolution times reflect a responsive and effective support system, vital for maintaining a positive customer experience.
Method of calculation
To calculate the average time to resolution, add up the total time taken to resolve a set of customer inquiries and then divide by the number of inquiries. For instance, if it took 8 hours to resolve 24 inquiries, the average time to resolution is 20 minutes per inquiry. Tracking this metric over different types of inquiries and comparing it against past performance can offer insights into the efficiency and effectiveness of your customer support team.
Tip to improve time to resolution
Quick resolution times are vital for customer satisfaction, but the quality of service is equally important. If resolution times are lengthy, investigate potential causes like training needs or complex queries. Efficiently addressing these issues can significantly boost satisfaction. Additionally, regularly reviewing individual cases with prolonged resolution times can uncover systemic issues or training opportunities. Remember, the goal is to provide swift, comprehensive solutions, balancing speed with thorough problem-solving to ensure a seamless support experience.
Metrics #10 – Interactions per ticket
Interactions per ticket measure the average number of exchanges between a customer and support agents needed to resolve an issue. This metric provides insight into the complexity of customer inquiries and the efficiency of your support team. A lower number generally indicates more efficient problem-solving capabilities, while a higher number might suggest a need for improved processes or training.
Method of calculation
To calculate interactions per ticket, count the total number of interactions (including messages, calls, transfers, or any other form of communication) for a set of resolved tickets and then divide by the total number of tickets. This will give you the average interactions needed per ticket. Monitoring this over time can help identify trends and areas for improvement in your customer service process.
Tip to improve interactions per ticket
Reducing the average number of interactions per ticket is crucial for efficient and customer-friendly support. High interaction counts may indicate that your team isn’t understanding issues quickly enough or that customers are being passed around between agents. To improve, ensure your team has access to comprehensive customer data and history to avoid repetitive questioning. Training agents to ask the right questions and offering canned responses for common issues can also speed up resolutions. Additionally, equipping your team with the tools to resolve inquiries on the first interaction whenever possible can significantly enhance the customer experience.
Metrics #11 – Unresolved query backlog
The unresolved query backlog represents the number of customer inquiries that remain unaddressed over a specific period. This metric is crucial for assessing the workload and efficiency of your customer service team. A growing backlog can indicate bottlenecks and a potential decline in customer satisfaction, as unresolved issues can lead to increased frustration and diminished trust in your service.
Method of calculation
To calculate the unresolved query backlog, subtract the number of inquiries resolved by your team from the total number of open inquiries. For instance, if there are 50 open customer inquiries and your team resolves 20 in a day, the backlog is 30 inquiries. Regularly monitoring this figure can help track your team’s progress and ability to keep up with customer demand.
Tip to improve unresolved query backlog
Maintaining a minimal unresolved query backlog is essential for providing timely and effective customer service. A consistently increasing backlog necessitates immediate action, such as hiring additional customer service staff or improving internal processes and efficiency. If slow responses from specific departments, like the product team, contribute to the backlog, establishing better communication and problem-solving mechanisms is crucial. Analyzing the reasons behind unresolved tickets can provide insights into systemic issues and areas for improvement.
Metrics #12 – Number of escalation requests
The number of escalation requests is a crucial customer service metric that counts how often a customer issue is transferred to a higher authority or specialized team for resolution. Frequent escalations might indicate complex issues or suggest that frontline support agents lack the necessary skills or resources. While some escalations are inevitable, a high rate can lead to customer frustration and extended resolution times.
Method of calculation
Calculate the escalation rate by dividing the total number of escalated inquiries by the total number of received inquiries. For example, if out of 100 total inquiries, 10 were escalated, then your escalation rate is 10%. Monitoring this metric helps assess the efficiency and capability of your initial support level and the complexity of issues encountered.
Tip to improve the number of escalation requests
The number of escalation requests can significantly impact customer satisfaction. A lower escalation rate generally means issues are resolved quickly and efficiently at the first point of contact, leading to happier customers. To minimize escalations, ensure your team is well-trained and has access to comprehensive knowledge resources. Encourage them to fully understand the problem before escalating and to provide detailed information to the next level to avoid customers having to repeat themselves. Analyzing common reasons behind escalations can also highlight specific areas for improvement, whether it’s additional training, process changes, or enhanced tool support. By effectively managing and reducing escalation requests, you can streamline the resolution process and enhance the overall customer experience.
Effective methods for tracking customer service metrics
Tracking customer service metrics effectively is key to gaining insights and making informed decisions. Various methods, including surveys, live chat, chatbots, CRM, and social media, offer unique advantages in capturing this valuable data. So, let’s explore these effective methods for tracking these crucial customer service performance metrics.
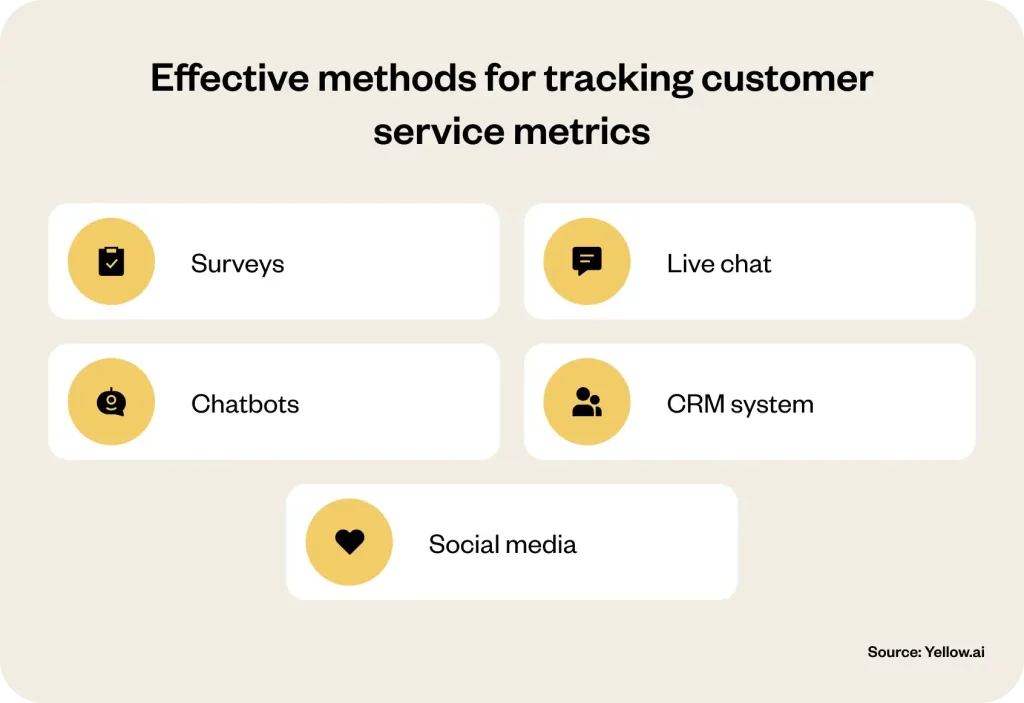
Method 1: Surveys
Surveys are a direct and versatile method for gauging customer satisfaction and service quality. They can be distributed via email, embedded on websites, or even conducted over the phone. By asking specific questions about various aspects of the service experience, businesses can collect valuable feedback and quantify it through metrics like CSAT, NPS, and CES. The key to effective surveys is crafting clear, concise questions that encourage honest and useful responses, enabling you to pinpoint areas for improvement and track changes over time.
Method 2: Live chat
Live chat offers real-time interaction with customers, making it an invaluable tool for measuring immediate satisfaction and understanding issues as they arise. It allows for quick resolution of problems and can significantly reduce response time and resolution time metrics. By analyzing chat transcripts, businesses can identify common issues, track the efficiency of their agents, and gather direct feedback from customers. Implementing live chat not only enhances the customer experience but also provides a rich source of data for improving service strategies.
Related read: What is live chat? The definitive guide for businesses
Method 3: Chatbots
Chatbots are the ace of spades in the deck of customer service trends and tools and now with no-code chatbot builders building chatbots has become very easy. They offer a 24/7 solution for tracking and improving various metrics. Capable of handling an array of inquiries simultaneously, chatbots significantly reduce response time and ticket volume while maintaining a constant level of service. They gather real-time data, providing insights into customer behavior and preferences, which can inform and enhance your customer service strategy. By automating routine tasks, chatbots free up human agents to focus on more complex issues, ensuring a well-rounded and efficient customer service experience.
Related read: Customer Service Chatbots – Benefits and Examples
Method 4: CRM system
CRM systems are pivotal in managing and analyzing customer interactions throughout their lifecycle. They serve as a comprehensive platform, collecting and organizing customer data from various touchpoints, including surveys, live chats, and purchase histories. This centralized database enables businesses to track customer service metrics like resolution times, satisfaction scores, and interaction history. By providing a detailed view of customer interactions, CRMs help identify trends, predict future behaviors, and tailor services to individual needs, ultimately enhancing the overall customer experience and fostering stronger, more personalized relationships.
Method 5: Social media
Social media platforms are powerful tools for tracking customer sentiment and engagement in real-time. They provide a public forum where customers often share their experiences and seek support. By monitoring mentions, comments, and direct messages, businesses can gauge customer satisfaction and respond promptly to inquiries or complaints. Analyzing social media interactions helps identify common concerns, measure response times, and understand the public perception of your brand. Engaging actively on these platforms not only aids in resolving issues but also demonstrates your commitment to customer service, enhancing your reputation and customer relationships.
Related read: An effective how-to guide for social media customer service
How Yellow.ai can help you automate your customer support?
In an era where customer support can make or break a business, Yellow.ai stands out by offering advanced AI chatbots that not only automate customer support but also help meticulously track and improve key customer service metrics. So, let’s explore how this transformative technology can enhance each aspect of your customer experience operations.
Collecting data
AI chatbots from Yellow.ai excel in collecting comprehensive data from each customer interaction. They record crucial details like queries, preferences, and satisfaction levels, essential for tracking all the customer service metrics. This continuous data collection enables businesses to analyze trends and make informed decisions, optimizing their customer service strategy. Each interaction becomes a valuable source of insight, allowing businesses to tailor their services effectively to meet customer needs.
Ensuring 24/7 availability
Yellow.ai’s AI chatbots provide uninterrupted 24/7 service, ensuring customers always have access to support. This continuous availability significantly improves metrics like Customer Satisfaction Score (CSAT) and Average Response Time, as customers receive prompt answers anytime. It also efficiently manages the Message Volume per Agent by handling routine inquiries, thus freeing up human agents for more complex issues. The result is a customer service experience that’s not only responsive but consistently available, boosting customer trust and satisfaction.
Scalable customer support
Yellow.ai’s platform is designed for scalability, ensuring that as your business grows, your customer support capabilities can expand seamlessly. This means maintaining high-quality service without significantly increasing resources or costs. As the customer base broadens, Yellow.ai’s chatbots efficiently manage the growing volume of interactions, ensuring your service quality remains consistent and responsive. With this scalable support, businesses can confidently grow, knowing their customer service is robust and adaptable.
Related read: How to scale customer support in your organization?
Conducting sentiment analysis
Yellow.ai’s AI chatbots incorporate sentiment analysis to discern and respond to customer emotions, enhancing interactions with empathy and accuracy. This ability to understand sentiment significantly boosts customer satisfaction and improves CSAT scores, as customers feel genuinely understood and supported. The chatbots’ empathetic responses ensure that each customer interaction is not only efficient but also emotionally resonant, fostering a positive customer experience.
Resolving customer’s most frequent queries
Yellow.ai’s AI chatbots efficiently handle and resolve common customer queries. Capable of assisting hundreds of customers simultaneously, these chatbots ensure prompt, accurate responses to frequent questions without delays, enhancing efficiency and customer satisfaction. This streamlined approach allows for quick, effective service, and meeting customer needs more efficiently.
Automating tedious tasks
Yellow.ai effectively automates routine customer support tasks, freeing up your team to address more specialized issues. This reduces burnout and enhances overall efficiency and job satisfaction. Experience the streamlined operations and improved customer support with our dynamic automation platform (DAP).
To further illustrate the impact of Yellow.ai’s AI chatbots, let’s delve into real-world case studies. These examples will showcase how businesses have successfully automated their customer service, enhancing efficiency and customer satisfaction with Yellow.ai’s innovative solutions.
Success stories from Yellow.ai
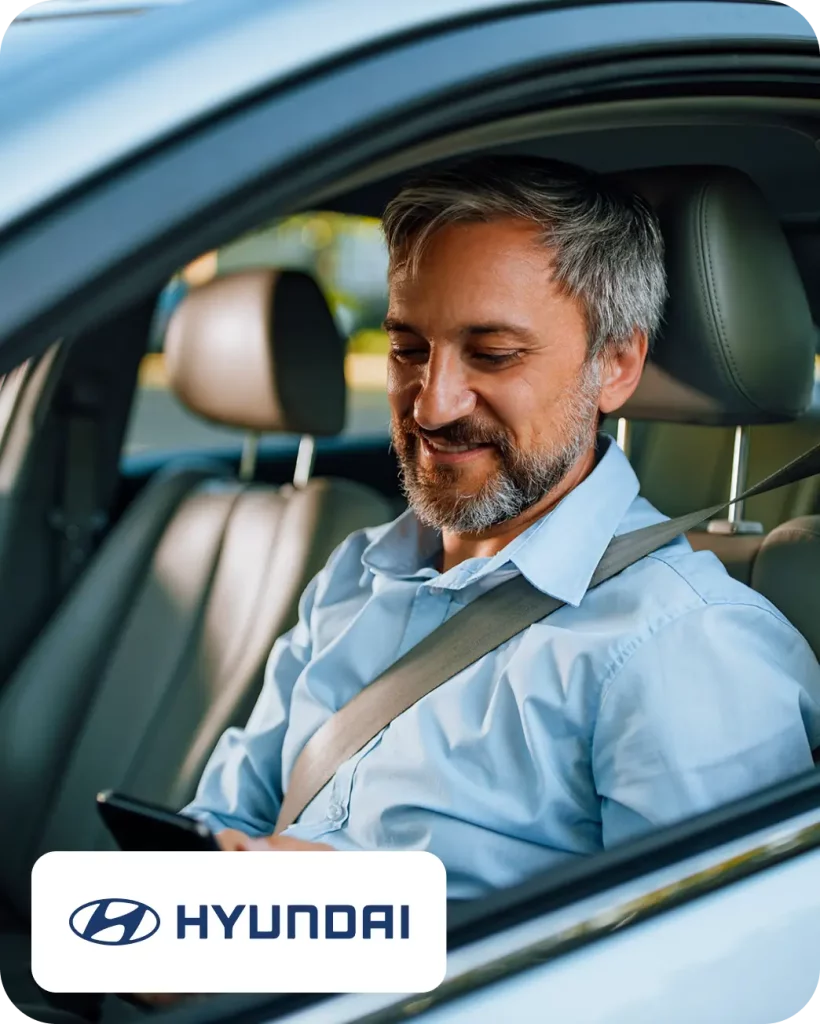
Hyundai boosts revenue and improves customer service with AI automation
Explore how Yellow.ai’s automation led to ~1000 car sales, a 10% retail conversion rate, and over 1.4 million user impressions
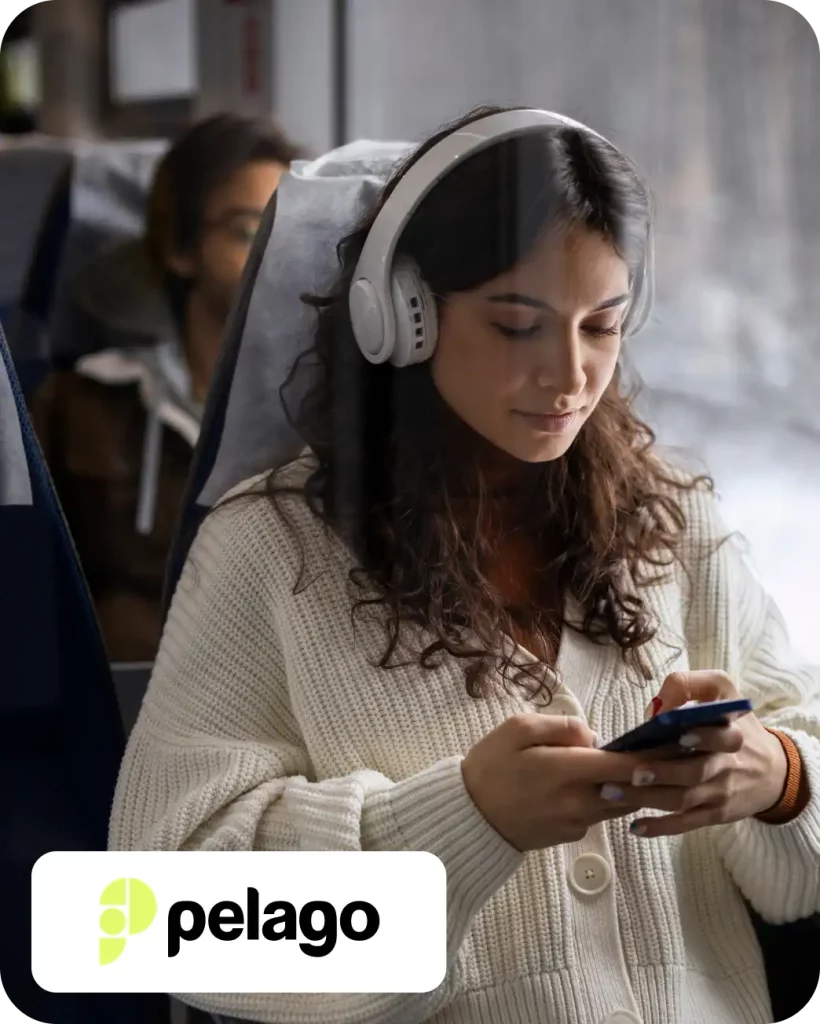
Pelago reimagines customer experience with generative AI powered conversational AI agents
Within a mere six weeks of going live, Pelago not only onboarded over 5,000 users but also achieved a striking 50% deflection rate!
Conclusion
As we’ve explored throughout this blog, tracking and improving customer service metrics are crucial for delivering a top-notch customer experience. Each metric, from ticket volume to number of escalation requests, offers valuable insights into the performance and efficiency of your customer service operations. Understanding and acting upon these metrics can significantly enhance customer satisfaction, loyalty, and overall business success. In today’s competitive landscape, where customer experience can set you apart, leveraging these insights is not just an option; it’s a necessity. In this journey towards exceptional customer service, Yellow.ai’s advanced AI chatbots emerge as a formidable ally. Whether through detailed data collection, sentiment analysis, or efficiently handling customer queries, Yellow.ai’s chatbots can elevate your customer service to new heights.
Empower your customer service with Yellow.ai
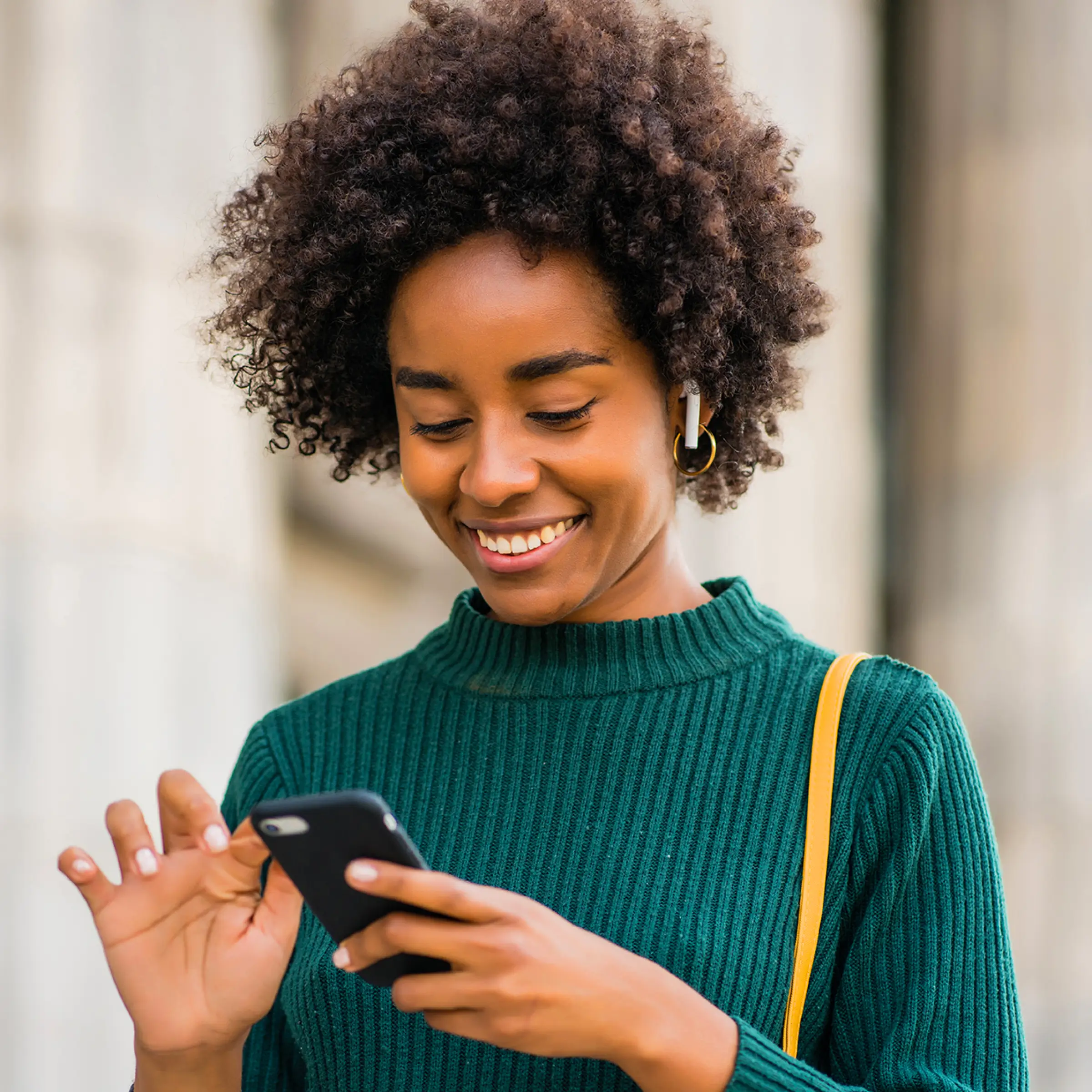